Community detection based on unsupervised attributed network embedding
EXPERT SYSTEMS WITH APPLICATIONS(2023)
摘要
Community detection methods based on attribute network representation learning are receiving increasing attention. However, few existing works are focused exclusively on unsupervised network representation learning for the task of community detection. They mainly capture information about the topology or attributes of the network, but do not fully utilize clustering-oriented information. In this paper, we present a community detection algorithm based on unsupervised attributed network embedding (CDBNE) to resolve the above issues. To be specific, we propose a framework that learns the representation based on network structure and attribute information and the clustering-oriented representation simultaneously. The framework includes the graph attention auto-encoder module, the modularity maximization module, and the self-training clustering module. Firstly, CDBNE encodes the topology structure and the node attribute with the graph attention mechanism. Secondly, it captures the mesoscopic community structure with modularity maximization. Finally, the self -training clustering module optimizes the representation learning process in a self-supervised manner to obtain high-quality node representation. The performance of CDBNE is verified with experiments on community detection tasks. According to the results on three datasets, CDBNE outperforms the state-of-the-art methods.
更多查看译文
关键词
Community detection, Graph auto-encoder, Unsupervised representation learning
AI 理解论文
溯源树
样例
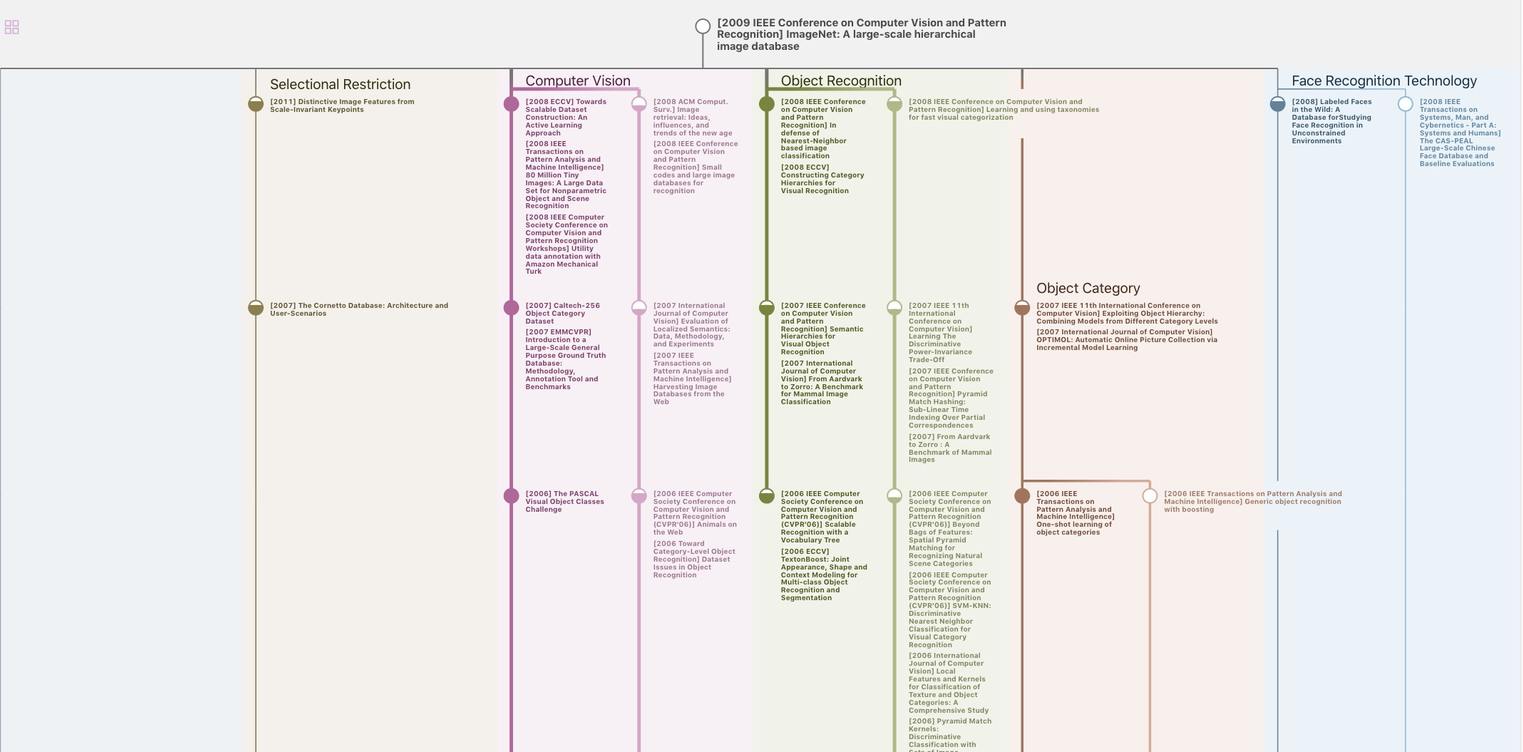
生成溯源树,研究论文发展脉络
Chat Paper
正在生成论文摘要