How robust are modern graph neural network potentials in long and hot molecular dynamics simulations?
MACHINE LEARNING-SCIENCE AND TECHNOLOGY(2022)
摘要
Graph neural networks (GNNs) have emerged as a powerful machine learning approach for the prediction of molecular properties. In particular, recently proposed advanced GNN models promise quantum chemical accuracy at a fraction of the computational cost. While the capabilities of such advanced GNNs have been extensively demonstrated on benchmark datasets, there have been few applications in real atomistic simulations. Here, we therefore put the robustness of GNN interatomic potentials to the test, using the recently proposed GemNet architecture as a testbed. Models are trained on the QM7-x database of organic molecules and used to perform extensive molecular dynamics simulations. We find that low test set errors are not sufficient for obtaining stable dynamics and that severe pathologies sometimes only become apparent after hundreds of ps of dynamics. Nonetheless, highly stable and transferable GemNet potentials can be obtained with sufficiently large training sets.
更多查看译文
关键词
graph neural networks, interatomic potentials, molecular dynamics
AI 理解论文
溯源树
样例
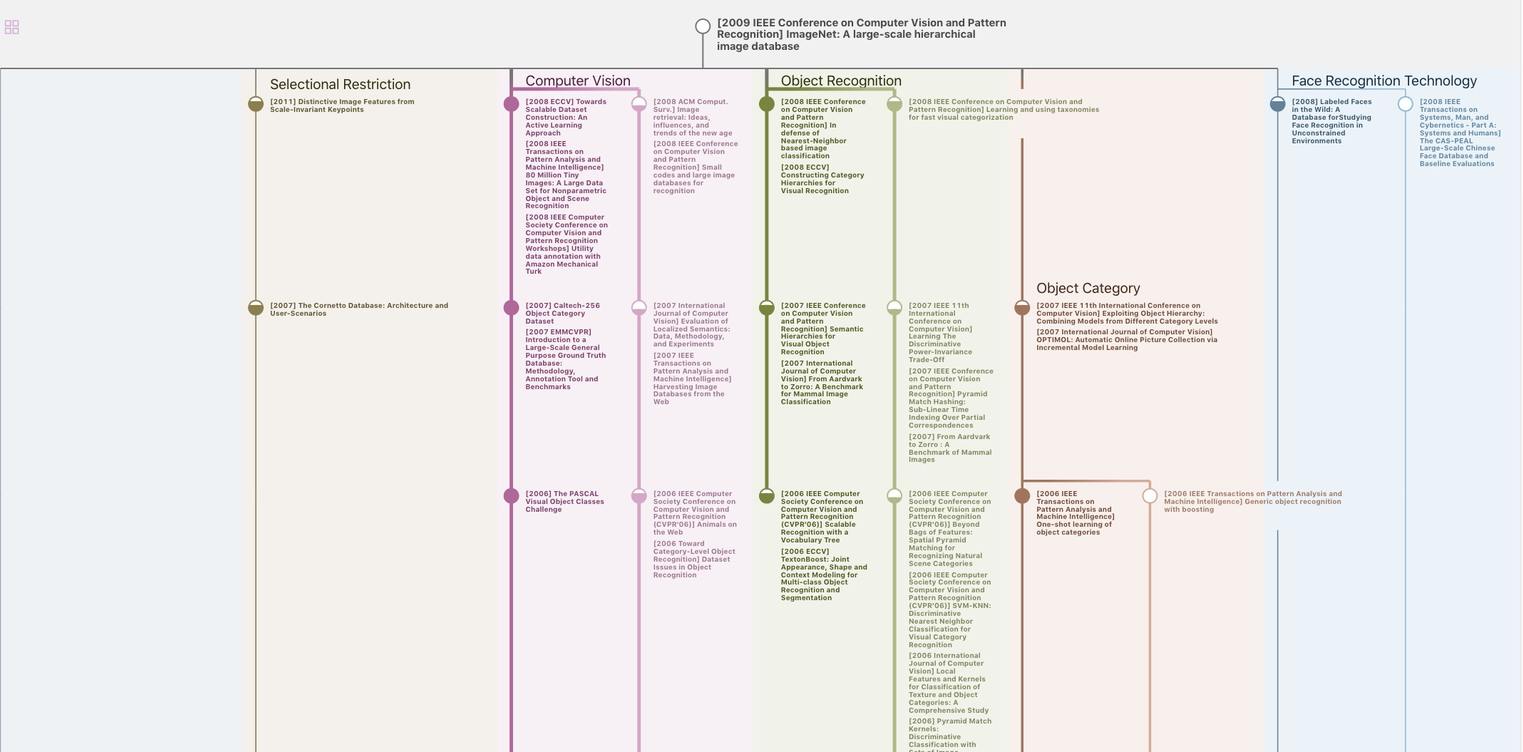
生成溯源树,研究论文发展脉络
Chat Paper
正在生成论文摘要