Analyzing Performance Effects of Neural Networks Applied to Lane Recognition under Various Environmental Driving Conditions
WORLD ELECTRIC VEHICLE JOURNAL(2022)
摘要
Lane detection is an essential module for the safe navigation of autonomous vehicles (AVs). Estimating the vehicle's position and trajectory on the road is critical; however, several environmental variables can affect this task. State-of-the-art lane detection methods utilize convolutional neural networks (CNNs) as feature extractors to obtain relevant features through training using multiple kernel layers. It makes them vulnerable to any statistical change in the input data or noise affecting the spatial characteristics. In this paper, we compare six different CNN architectures to analyze the effect of various adverse conditions, including harsh weather, illumination variations, and shadows/occlusions, on lane detection. Among all the aforementioned adverse conditions, harsh weather in general and snowy night conditions particularly affect the performance by a large margin. The average detection accuracy of the networks decreased by 75.2%, and the root mean square error (RMSE) increased by 301.1%. Overall, the results show a noticeable drop in the networks' accuracy for all adverse conditions because the features' stochastic distributions change for each state.
更多查看译文
关键词
autonomous vehicles, benchmark, lane detection, pre-trained networks, transfer learning
AI 理解论文
溯源树
样例
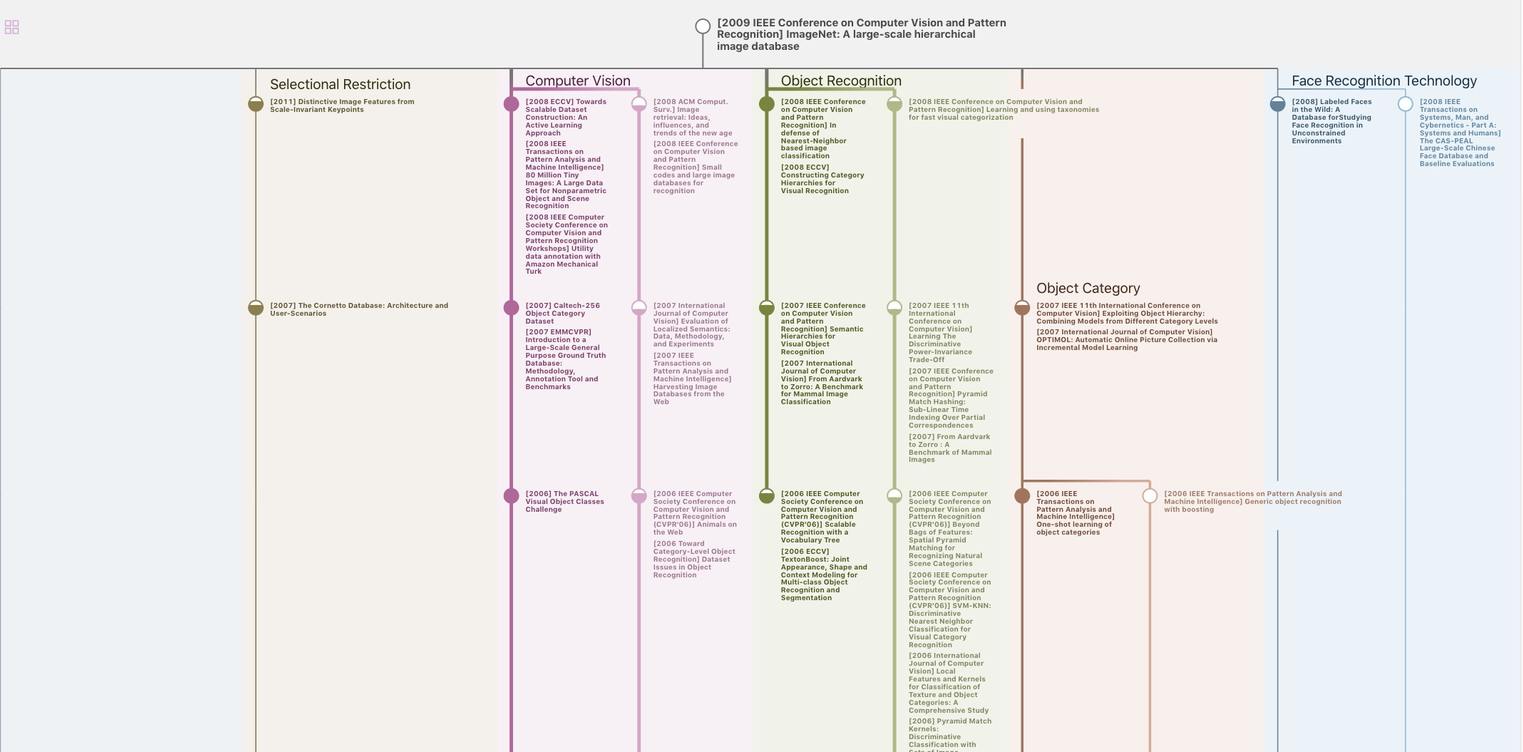
生成溯源树,研究论文发展脉络
Chat Paper
正在生成论文摘要