Sequential clustering and classification using deep learning technique and multi-objective sine-cosine algorithm
COMPUTERS & INDUSTRIAL ENGINEERING(2022)
摘要
This study introduced a novel data analytics-based sequential clustering and classification (SCC) approach. The proposed approach named deep MOSCA-SCC integrates a multi-objective sine-cosine algorithm (MOSCA), deep clustering technique, and classification algorithms to exploit data structure before implementing the prediction model. Herein, the autoencoder combined with the K-means algorithm was utilized for the deep clustering to reveal the data pattern. Regarding classification, support vector machine, back-propagation neural network, and decision tree classification were implemented to explore the correlated factors with the revealed patterns. To evaluate the performance of the proposed method, a comparison was conducted between the proposed deep MOSCA-SCC and other benchmark algorithms, including the NSGAII-SCC and the regular MOSCA-SCC. The deep MOSCA-SCC achieved better performance than other algorithms in terms of clustering sum of squared error and classification accuracy. In addition, deep MOSCA-SCC with a decision tree yielded the best accuracies with 92.6% and 87% when the numbers of clusters were equal to 3 and 4, respectively.
更多查看译文
关键词
Deep clustering, Multi-objective optimization, Feature selection, Multi-objectivesine-cosine algorithm, (MOSCA), Sequential clustering and classification
AI 理解论文
溯源树
样例
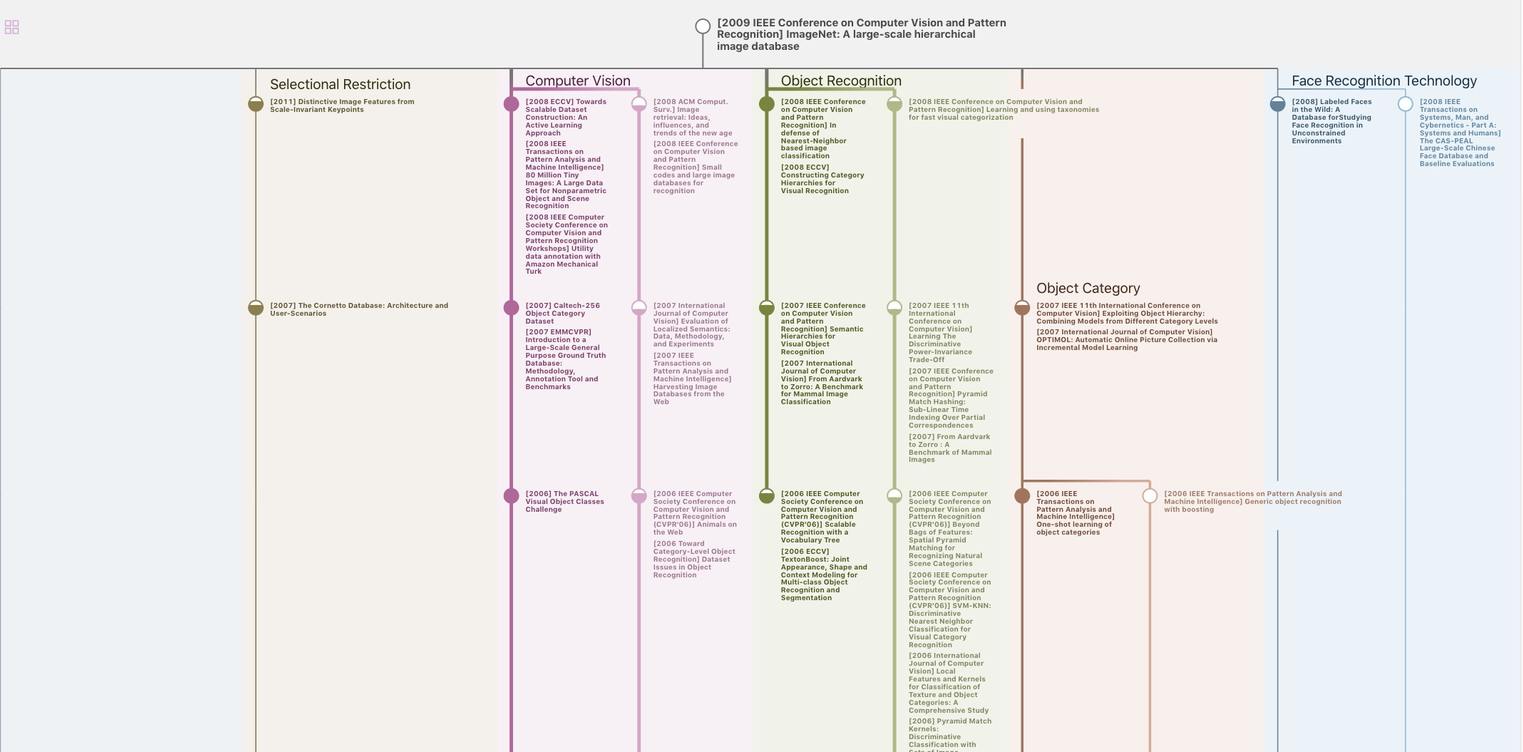
生成溯源树,研究论文发展脉络
Chat Paper
正在生成论文摘要