A Comparison of Neural Network-Based Strategies for Diesel Engine Air Handling Control
2022 AMERICAN CONTROL CONFERENCE (ACC)(2022)
摘要
Diesel air handling systems are becoming increasingly complex and commonly feature technologies such as exhaust gas recirculation (EGR) and variable geometry turbochargers (VGTs) in order to meet stringent emissions and fuel economy requirements. The control of such systems is challenging because they are nonlinear and have coupled dynamics. In this paper, artificial neural networks (ANNs) and recurrent neural networks (RNNs) are leveraged to control the low pressure (LP) EGR valve position and VGT vane position simultaneously on a light-duty multi-cylinder diesel engine. Intake manifold pressure (IMP) and the air-fuel equivalence ratio (lambda) are selected as control objectives, since they are directly relevant to engine emissions and power output. In addition, both signals are available on production engines so no additional hardware costs will be introduced. Steady-state experimental data is used to train the neural networks (NNs). The ANNs and RNNs with the minimum mean square error (MSE) for training data sets are further compared to conventional proportional-integral (PI) control with the validation data sets. Both of the selected NN controllers show almost no overshoot during the transient process and have steady-state errors of less than 4.0% for IMP or lambda, thus showing potential for further use in engine air handling control.
更多查看译文
关键词
network-based
AI 理解论文
溯源树
样例
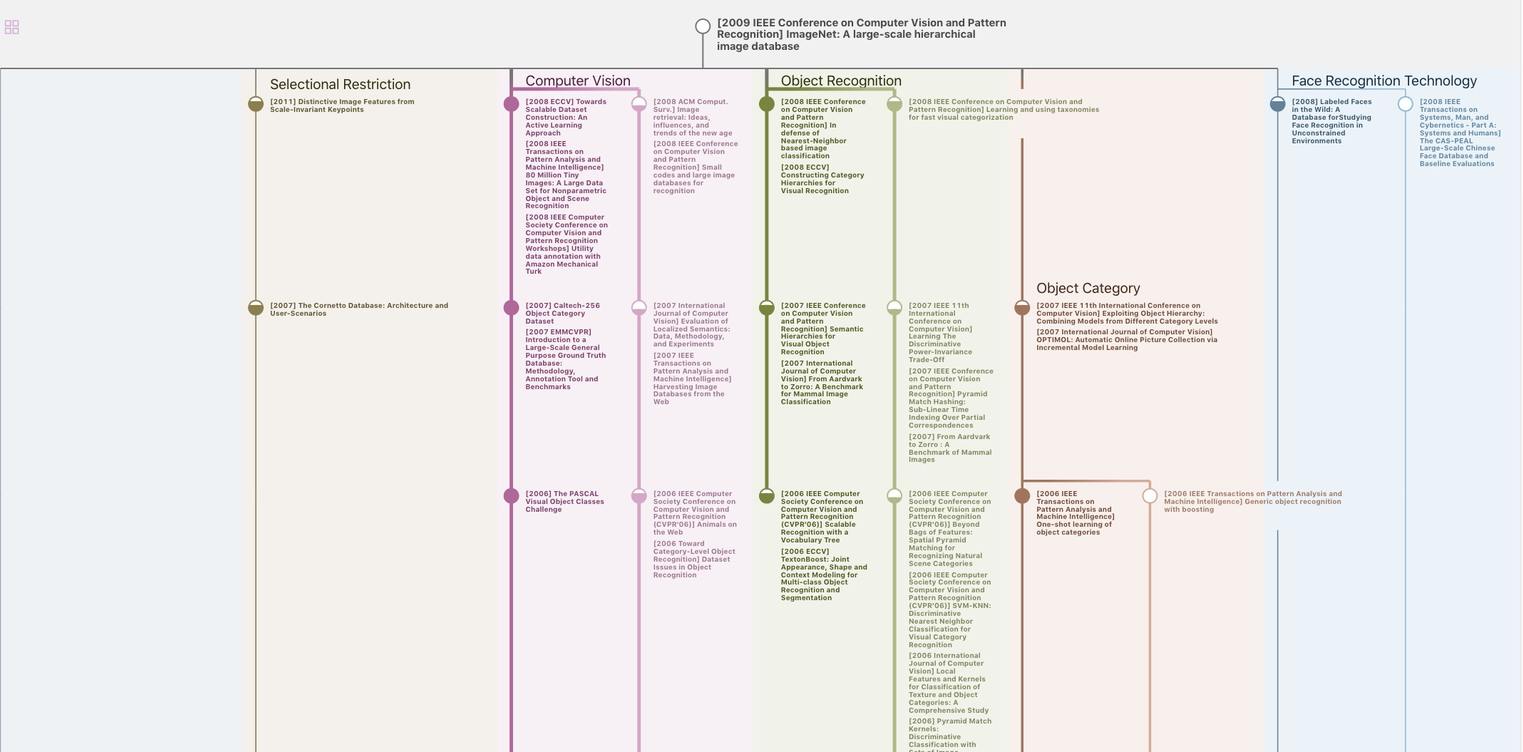
生成溯源树,研究论文发展脉络
Chat Paper
正在生成论文摘要