Personality Traits Can Predict Architectural Preferences: A Machine Learning Approach
PSYCHOLOGY OF AESTHETICS CREATIVITY AND THE ARTS(2022)
摘要
Personalization of different aspects of architectural designs is one of the most novel issues in the modern world. The present study aimed to predict the association between personality traits and architectural preferences. A total of 352 participants with architectural (experts) and nonarchitectural (nonexperts) education were asked to complete a demographic profile and the NEO Five-Factor Personality Inventory. They were then asked to choose their preferred images, which were previously rated based on three aesthetic variables, that is, color-contrast, abstractness-concreteness, and spatial openness, in a series of two-alternative forced-choice questions. By using a random forest classifier, an accuracy of 73 to 84% was achieved for the variables. Due to the complexity of rules in the random forest model, data were explored for more interpretable rules, and a rule-based classifier (Waikato Environment for Knowledge Analysis software) was used. Based on the findings, introverts had the opposite behavior compared to the general population; they preferred images of enclosed spaces and with high color contrast. They also preferred popular architectural styles to high-style designs. Otherwise, the preference for greater spatial openness was common in both expert and nonexpert groups, although it was more noticeable in female extroverts. Nonexperts with high levels of openness to experience were mostly attracted to abstract images. Experts and nonexperts showed similar preferences in terms of color contrast and spatial openness, while there was a significant difference between the two groups regarding their preferred abstract and concrete concepts. In conclusion, educational background and personality traits could influence aesthetic preferences.
更多查看译文
关键词
quadridimensional systematic model, personality traits, architectural preferences, machine learning, expert, nonexpert
AI 理解论文
溯源树
样例
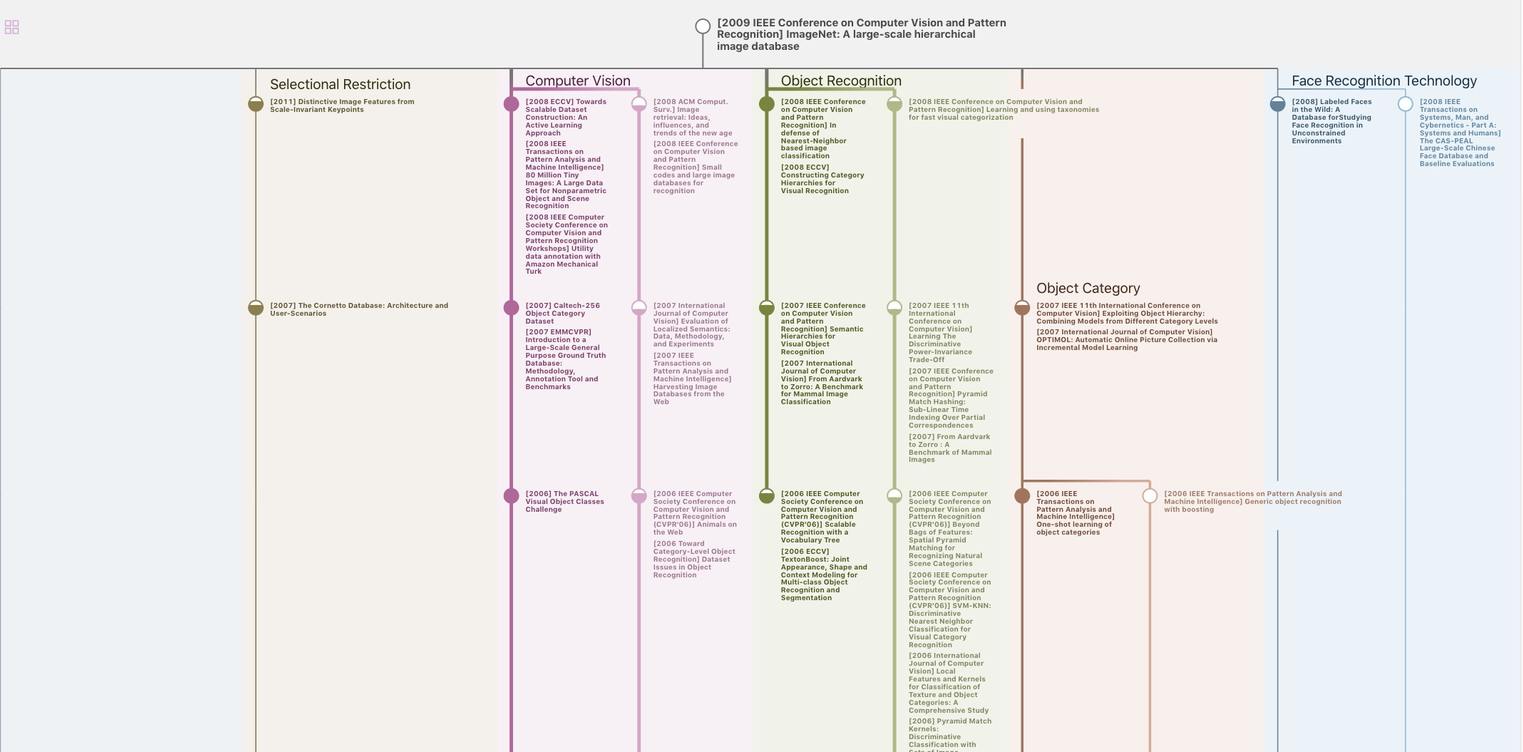
生成溯源树,研究论文发展脉络
Chat Paper
正在生成论文摘要