Dual sampling neural network: Learning without explicit optimization
PHYSICAL REVIEW RESEARCH(2022)
摘要
Artificial intelligence using neural networks has achieved remarkable success. However, optimization pro-cedures of the learning algorithms require global and synchronous operations of variables, making it difficult to realize neuromorphic hardware, a promising candidate of low-cost and energy-efficient artificial intelligence. The optimization of learning algorithms also fails to explain the recently observed criticality of the brain. Cortical neurons show a critical power law implying the best balance between expressivity and robustness of the neural code. However, the optimization gives less robust codes without the criticality. To solve these two problems simultaneously, we propose a model neural network, dual sampling neural network, in which both neurons and synapses are commonly represented as a probabilistic bit like in the brain. The network can learn external signals without explicit optimization and stably retain memories while all entities are stochastic because seemingly optimized macroscopic behavior emerges from the microscopic stochasticity. The model reproduces various experimental results, including the critical power law. Providing a conceptual framework for computation by microscopic stochasticity without macroscopic optimization, the model will be a fundamental tool for developing scalable neuromorphic devices and revealing neural computation and learning.
更多查看译文
AI 理解论文
溯源树
样例
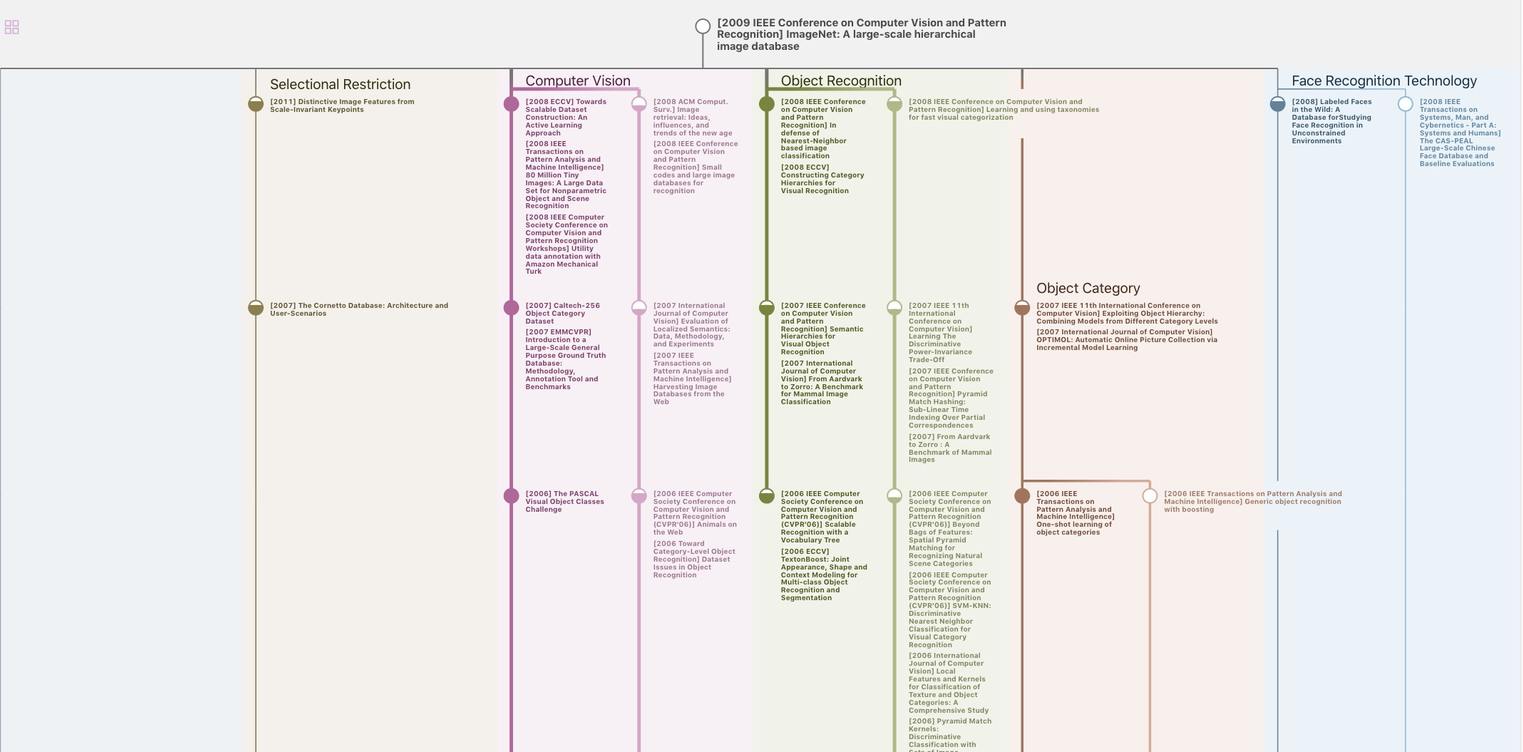
生成溯源树,研究论文发展脉络
Chat Paper
正在生成论文摘要