Extended Unbiased Distribution Free Estimator With Mean Structures
STRUCTURAL EQUATION MODELING-A MULTIDISCIPLINARY JOURNAL(2023)
摘要
To handle the nonnormal data issue, Browne proposed an unbiased distribution free (DF) estimator (gamma(U)(DF)) and an asymptotically distribution free estimator (gamma(ADF)) of the covariance matrix of sample variances/covariances gamma to calculate robust test statistics and robust standard errors. However, gamma(U)(DF) is ignored in methodological and substantive research, and has not been extended to models with mean structures. To improve robust standard errors and the model fit statistic for nonnormal data with mean structures (e.g., growth curve models), we propose an unbiased distribution free estimator with mean structures considered. In growth curve models, we apply gamma(U)(DF) to four robust statistics that have relatively simple forms and denote them as T-SB(U), T-MVA(U), T-MVA2(U), and T-COR1(U). We compare their performance with 7 robust test statistics that employ gamma(ADF). We find that with the same model fit statistic, gamma(U )(DF)generally leads to smaller Anderson-Darling distances from the theoretical distribution than gamma(ADF), except T-MVA2(U) in some skewed cases. Additionally, the p-values from T-MVA2(U) are distributed closest to the theoretical distribution Uniform(0,1) among the 12 examined statistics. In terms of Type I error rates, T-MVA2(U) and T-MVA2 are the most stable statistics. Additionally, gamma(U )(DF)provides smaller relative biases of the robust SE estimates than gamma(ADF). Hence, we suggest using gamma(U)(DF) in both model fit statistics and robust SE calculation. Among the model fit statistics using gamma U-DF, we suggest T-MVA2(U).
更多查看译文
关键词
Distribution free estimator, growth curve model, model fit statistics, robust statistics, structural equation modeling
AI 理解论文
溯源树
样例
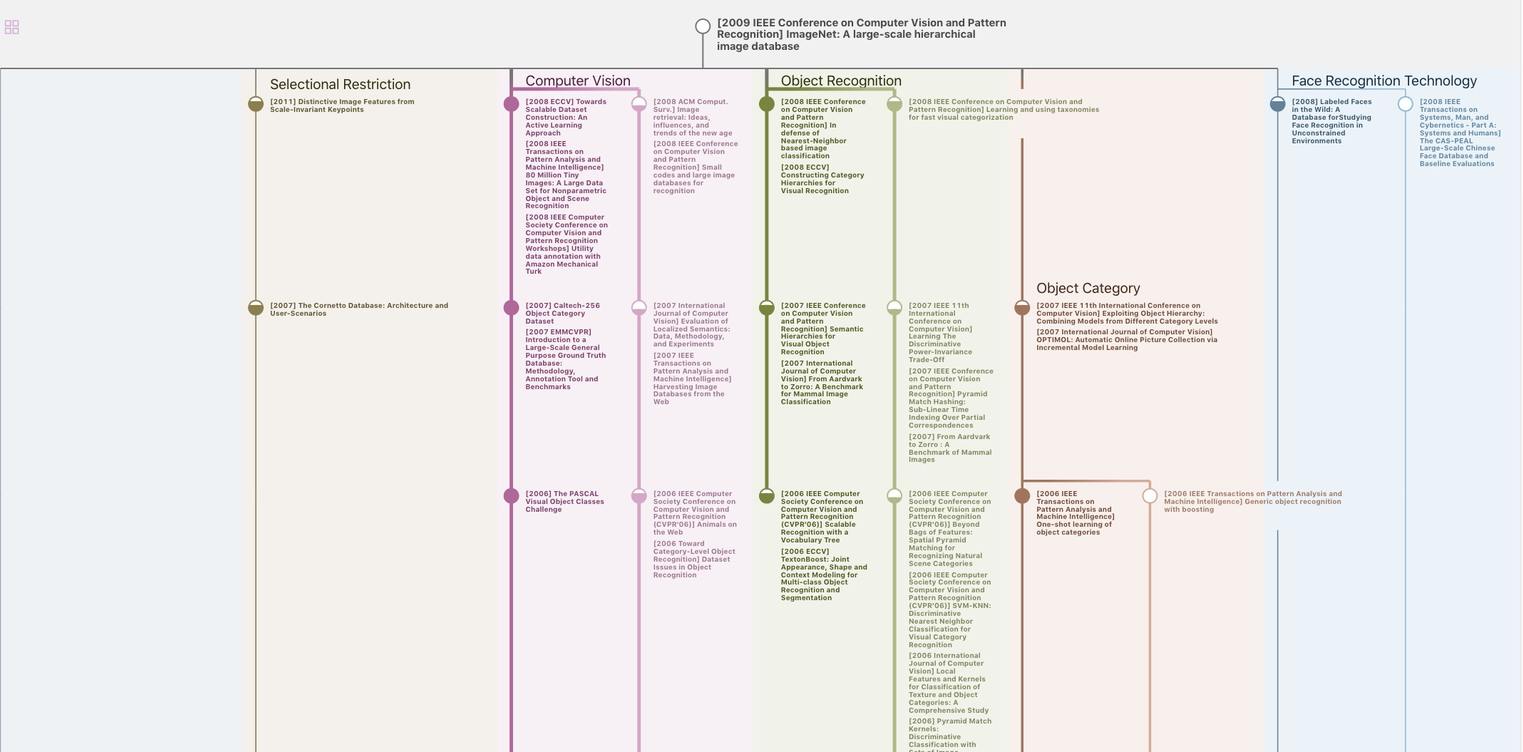
生成溯源树,研究论文发展脉络
Chat Paper
正在生成论文摘要