Non-convex regularization and accelerated gradient algorithm for sparse portfolio selection
OPTIMIZATION METHODS & SOFTWARE(2023)
摘要
In portfolio optimization, non-convex regularization has recently been recognized as an important approach to promote sparsity, while countervailing the shortcomings of convex penalty. In this paper, we customize the non-convex piecewise quadratic approximation (PQA) function considering the background of portfolio management and present the PQA regularized mean-variance model (PMV). By exposing the feature of PMV, we prove that a KKT point of PMV is a local minimizer if the regularization parameter satisfies a mild condition. Besides, the theoretical sparsity of PMV is analysed, which is associated with the regularization parameter and the weight parameter. To solve this model, we introduce the accelerated proximal gradient (APG) algorithm, whose improved linear convergence rate compared with proximal gradient (PG) algorithm is developed. Moreover, the optimal accelerated parameter of APG algorithm for PMV is attained. These theoretical results are further illustrated with numerical experiments. Finally, empirical analysis demonstrates that the proposed model has a better out-of-sample performance and a lower turnover than many other existing models on the tested datasets.
更多查看译文
关键词
Sparse portfolio selection, non-convex regularization, accelerated proximal algorithm, linear convergence, Sharpe ratio, turnover
AI 理解论文
溯源树
样例
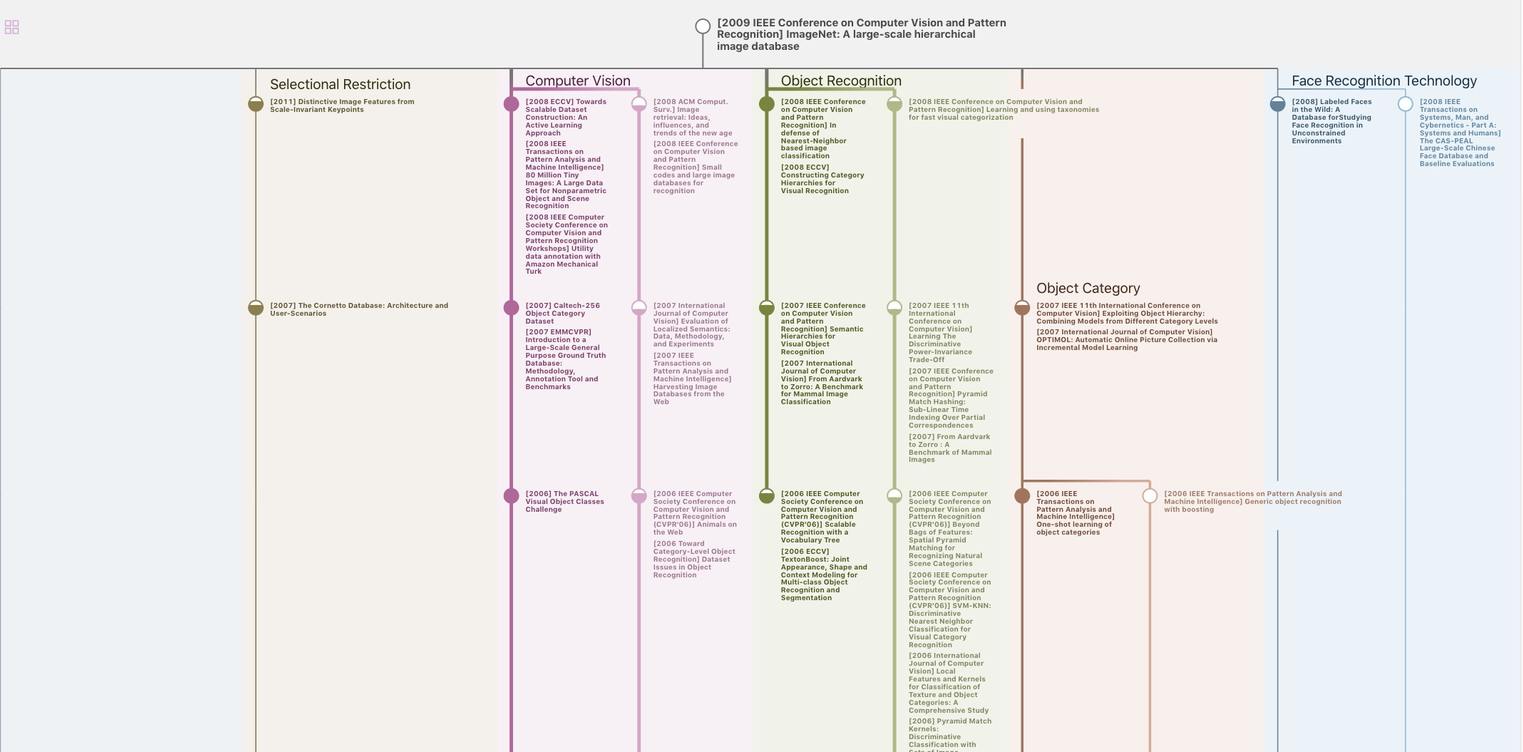
生成溯源树,研究论文发展脉络
Chat Paper
正在生成论文摘要