Multi-scale feature learning network with channel self-attention for remote sensing single-image super-resolution
INTERNATIONAL JOURNAL OF REMOTE SENSING(2022)
摘要
The performance of the remote sensing image super-resolution (SR) task has been significantly improved by applying deep learning. However, previously reported methods usually require sufficient synthetic datasets obtained by bicubic downsampling for long-term training, which can get better results when the input of the test phase is still the bicubic downsampling low-resolution (LR) image. However, when the input does not meet this bicubic downsampling situation, the performance of the model will be significantly degraded, and the generated SR image will be blurred. In order to better adapt to remote sensing image SR in different situations, this paper proposes a multi-scale feature learning network with channel self-attention for remote sensing single-image super-resolution (MSFLCSA). The proposed MSFLCSA does not need any extra synthetic paired dataset but one LR input image for training. Further, MSFLCSA uses a well-designed channel self-attention multi-scale feature learning network to fully learn the repetitive multi-scale features of the input image to realize remote sensing image SR tasks. Specifically, the proposed MSFLCSA extracts features of different levels through multi-column convolutions with different receptive fields to better learn the multi-scale features inside remote sensing images. In addition, the channel self-attention module constructs the channel dependence relationship between channels, selectively emphasizes the interdependent channel features, and further improves the learned multi-scale feature representation. Various types of qualitative and quantitative experiments have validated the effectiveness of MSFLCSA. Compared with advanced technologies, MSFLCSA has achieved superior performance.
更多查看译文
关键词
Channel self-attention, multi-scale feature, remote sensing image, image super-resolution, single image
AI 理解论文
溯源树
样例
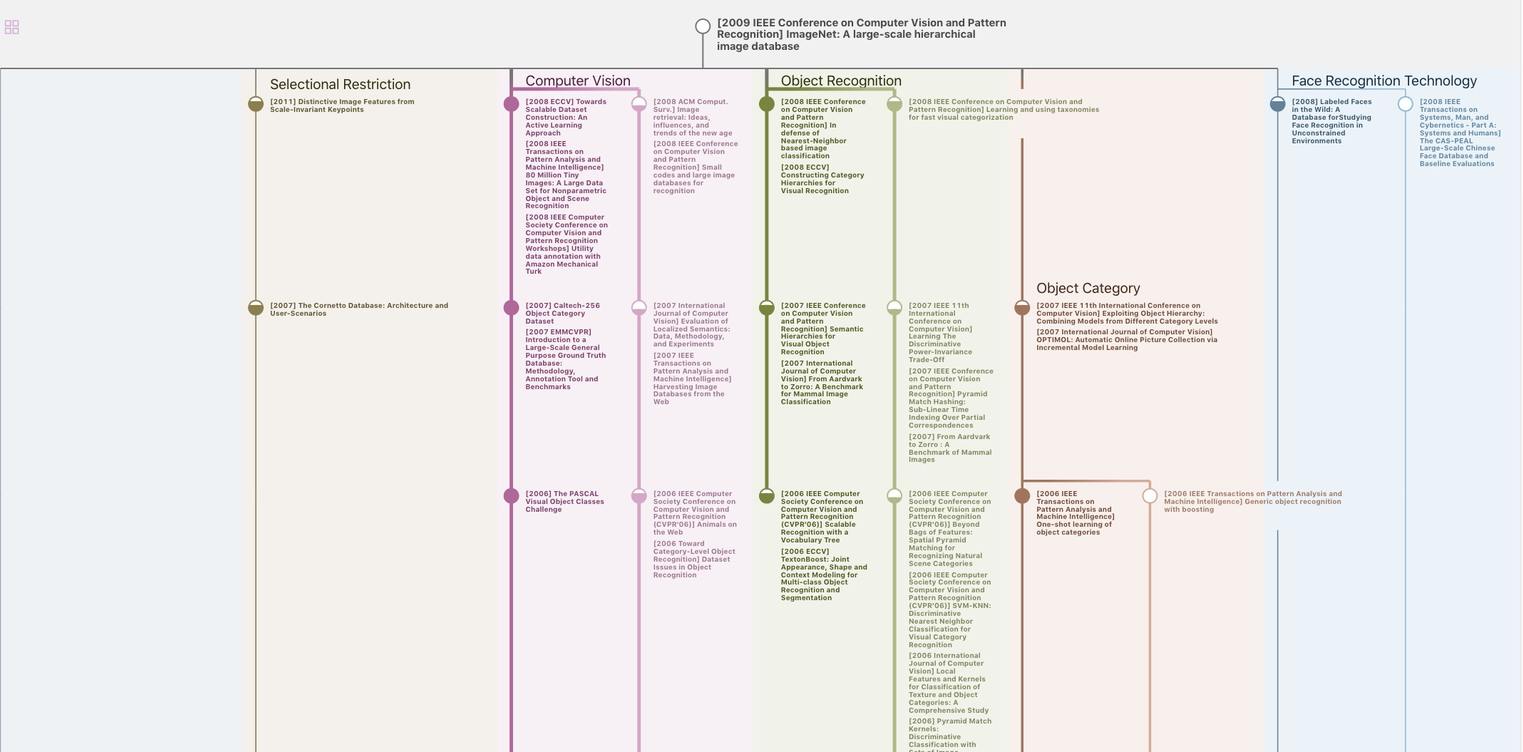
生成溯源树,研究论文发展脉络
Chat Paper
正在生成论文摘要