A novel YOLOv4-modified approach for efficient object detection in satellite imagery
EXPERT SYSTEMS(2023)
摘要
Interpreting high-resolution satellite imagery could be an expensive and time-consuming task for human eyes. Computer Vision and Deep Learning techniques can help to solve this major problem by applying detection algorithms, which can ease the task of analysing such images for the benefit of humans. It can help in changing the way we comprehend and anticipate the economic activity around the world. Such techniques help us to observe the urban development in high security areas such as national and international borders. Constant progressions in improving and making satellites deployment, a cost-effective process to strengthen the networks of satellite orbiting the earth is one of the reasons such tasks can be easily solved with the help of high-resolution images. Current computer vision research works have achieved significant milestones in accuracy and speed but, there are still room for improvements. In this paper, we addressed some of these methods to bring them to a combined pipeline and proposed a set of improvements to further improve the speed and the accuracy of the detections. We proposed a unified framework, which combines several object detection algorithms and the state-of-art architecture of YoloV4 along with the TensorFlow object detection API. This framework can detect small and well as large objects with improved speed and accuracy by using two detectors for different scales. Evaluation ran on these high-resolution images yield mAP of 85.6% F1-score of 0.84.
更多查看译文
关键词
adaptive pipeline inference system (APIS),computer vision,deep learning,satellite imagery,TensorFlow,YoloV4
AI 理解论文
溯源树
样例
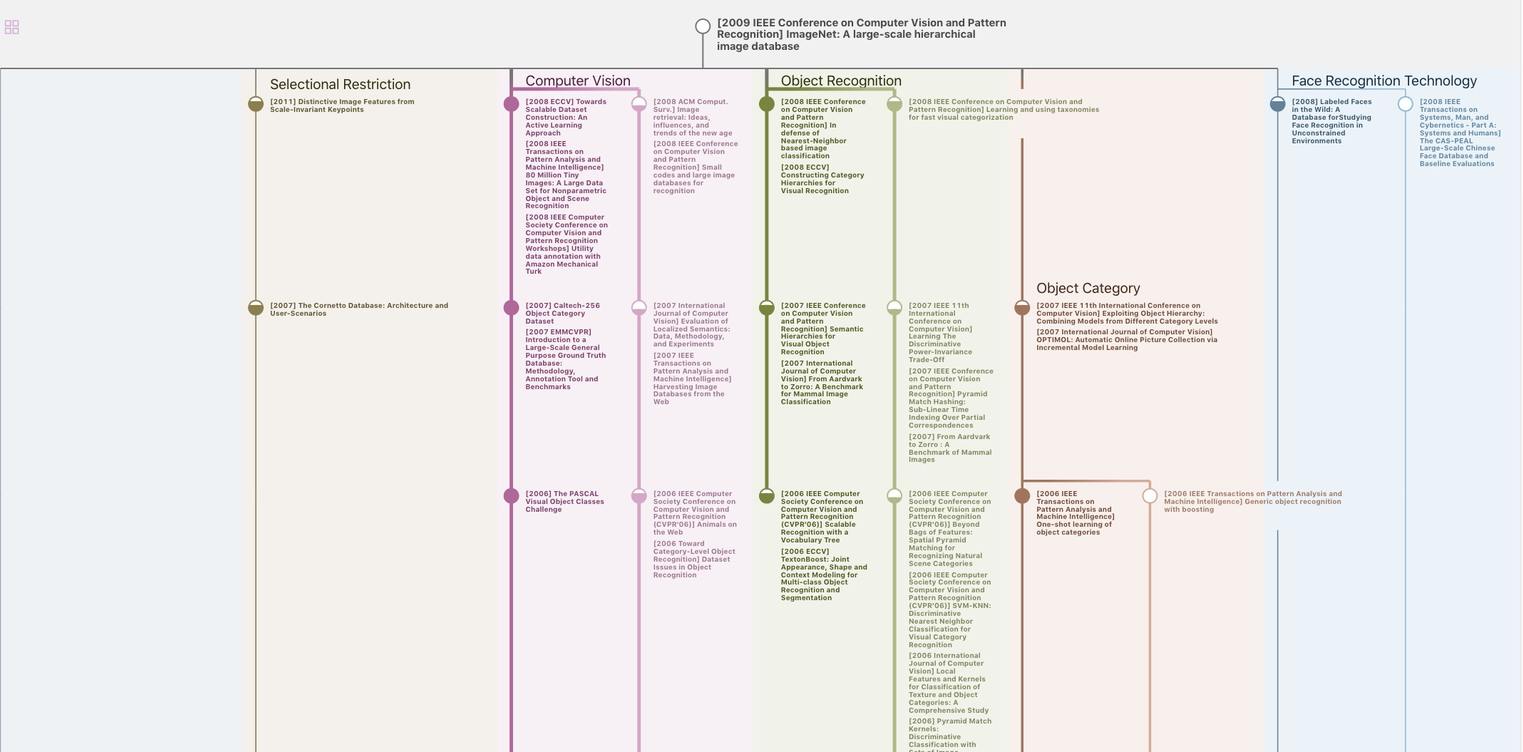
生成溯源树,研究论文发展脉络
Chat Paper
正在生成论文摘要