Customized Instance Random Undersampling to Increase Knowledge Management for Multiclass Imbalanced Data Classification
SUSTAINABILITY(2022)
摘要
Imbalanced data constitutes a challenge for knowledge management. This problem is even more complex in the presence of hybrid (numeric and categorical data) having missing values and multiple decision classes. Unfortunately, health-related information is often multiclass, hybrid, and imbalanced. This paper introduces a novel undersampling procedure that deals with multiclass hybrid data. We explore its impact on the performance of the recently proposed customized naive associative classifier (CNAC). The experiments made, and the statistical analysis, show that the proposed method surpasses existing classifiers, with the advantage of being able to deal with multiclass, hybrid, and incomplete data with a low computational cost. In addition, our experiments showed that the CNAC benefits from data sampling; therefore, we recommend using the proposed undersampling procedure to balance data for CNAC.
更多查看译文
关键词
imbalanced data classification, knowledge management, undersampling, decision-making, artificial intelligence
AI 理解论文
溯源树
样例
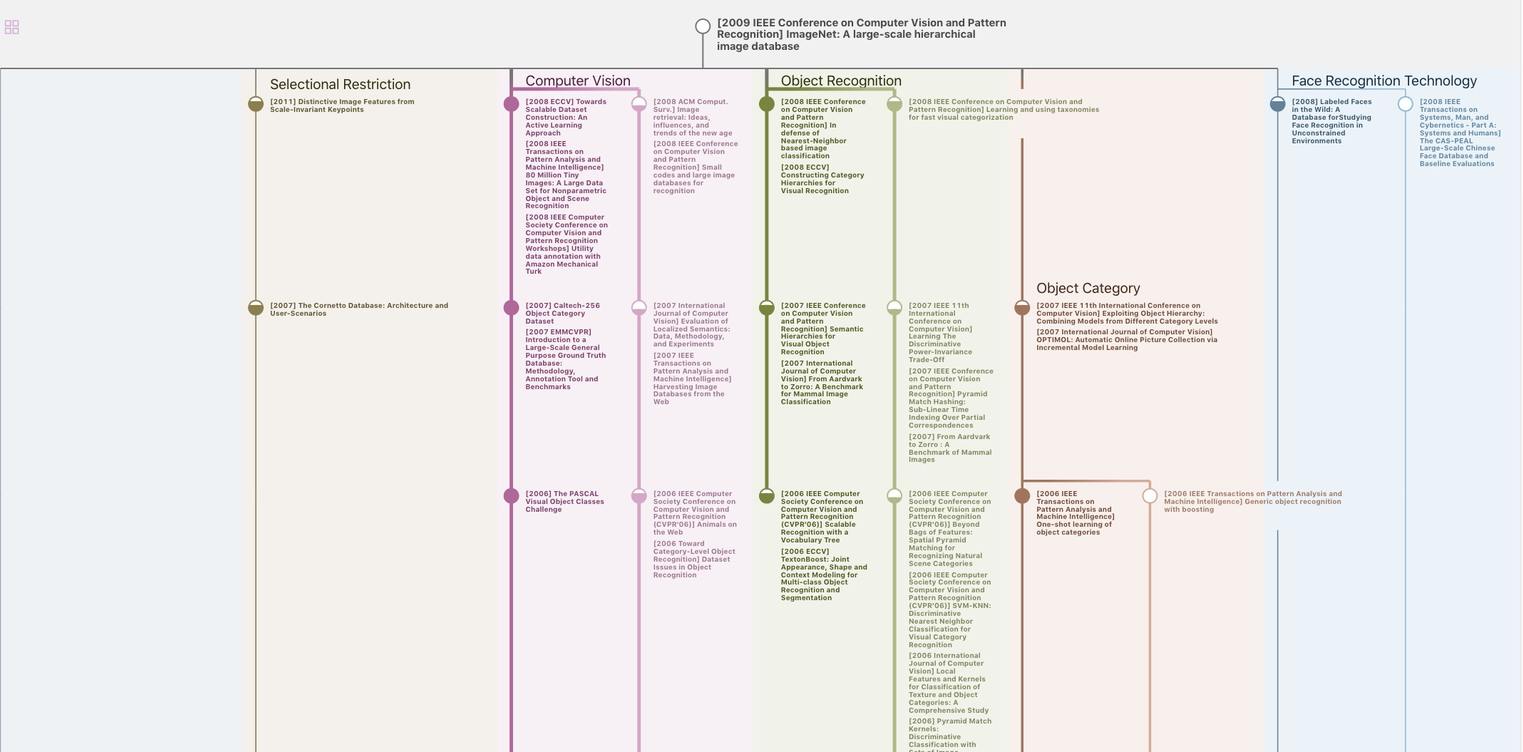
生成溯源树,研究论文发展脉络
Chat Paper
正在生成论文摘要