Machine-learning for designing nanoarchitectured materials by dealloying
COMMUNICATIONS MATERIALS(2022)
摘要
Machine learning-augmented materials design is an emerging method for rapidly developing new materials. It is especially useful for designing new nanoarchitectured materials, whose design parameter space is often large and complex. Metal-agent dealloying, a materials design method for fabricating nanoporous or nanocomposite from a wide range of elements, has attracted significant interest. Here, a machine learning approach is introduced to explore metal-agent dealloying, leading to the prediction of 132 plausible ternary dealloying systems. A machine learning-augmented framework is tested, including predicting dealloying systems and characterizing combinatorial thin films via automated and autonomous machine learning-driven synchrotron techniques. This work demonstrates the potential to utilize machine learning-augmented methods for creating nanoarchitectured thin films.
更多查看译文
关键词
Nanoscale materials,Techniques and instrumentation,Materials Science,general
AI 理解论文
溯源树
样例
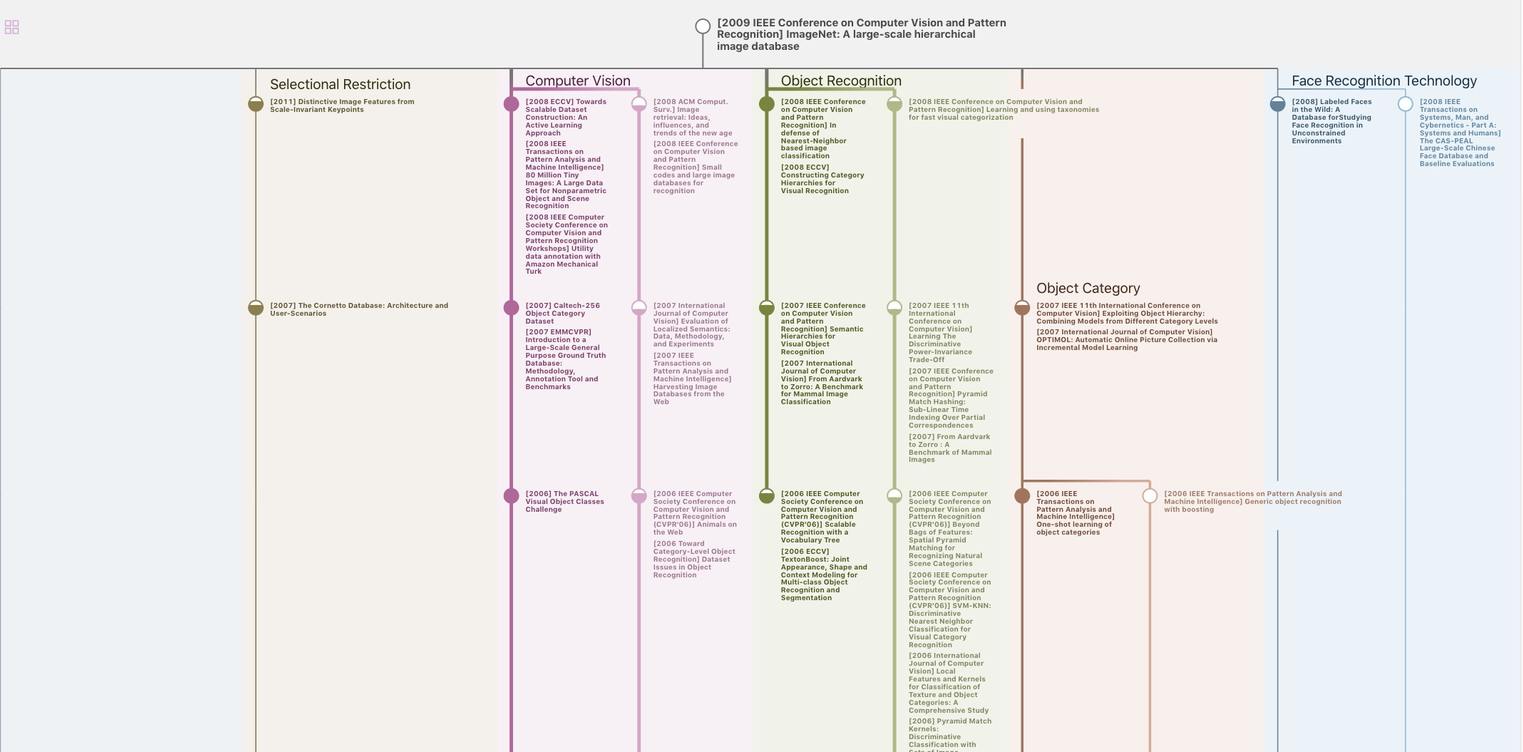
生成溯源树,研究论文发展脉络
Chat Paper
正在生成论文摘要