Terrain-Guided Flatten Memory Network for Deep Spatial Wind Downscaling
IEEE JOURNAL OF SELECTED TOPICS IN APPLIED EARTH OBSERVATIONS AND REMOTE SENSING(2022)
摘要
High-resolution wind analysis plays an essential role in pollutant dispersion and renewable energy utilization. This article focuses on spatial wind downscaling. Specifically, a novel terrain-guided flatten memory network (abbreviated as TIGAM) with axial similarity constraint is proposed. TIGAM consists of three elaborately designed blocks, i.e., the similarity block, the reconstruction block, and the denoise block. To achieve long-spatial dependence, the similarity block interpolates low-resolution data to high resolution in an axial attention manner. Meanwhile, the reconstruction block aims to obtain a clearer high-resolution representation in closed form. Taking both of the meteorological prior and network design principle into consideration, this article also proposes a flatten memory module with learnable input for high-resolution denoising. Furthermore, for accurate detail reconstruction, a terrain-guided enhanced loss is presented benefitting from the high-resolution remote sensing data. This loss function integrates wind spatial distribution and terrain elegantly. Extensive quantitative and qualitative experiments demonstrate the superiority of the proposed TIGAM.
更多查看译文
关键词
Spatial resolution, Superresolution, Meteorology, Surfaces, Mathematical models, Image reconstruction, Wind speed, Deep learning, flatten memory network, image super-resolution, meteorological methods, spatial wind downscaling
AI 理解论文
溯源树
样例
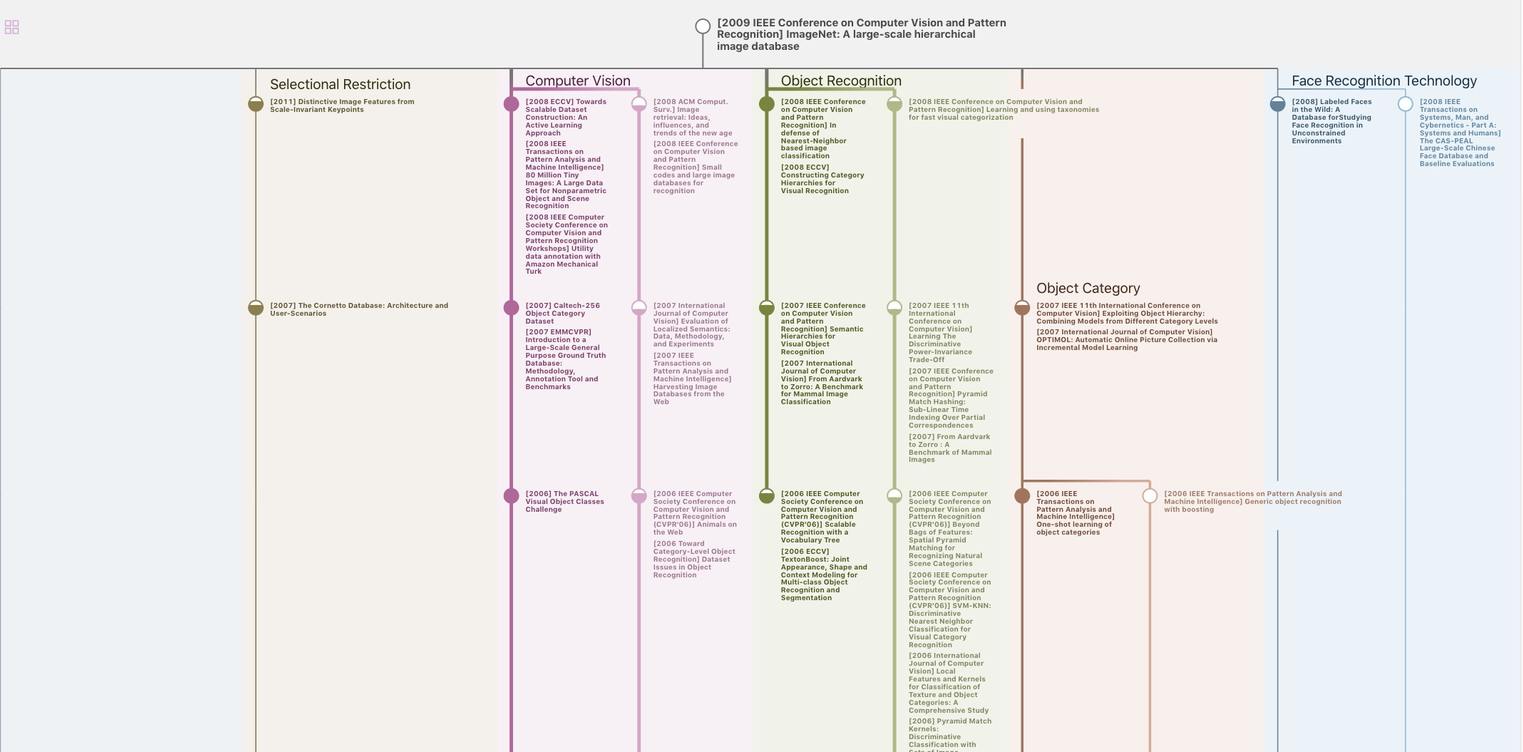
生成溯源树,研究论文发展脉络
Chat Paper
正在生成论文摘要