Privacy-Preserving Vertical Collaborative Logistic Regression without Trusted Third-Party Coordinator
SECURITY AND COMMUNICATION NETWORKS(2022)
摘要
Collaborative learning is an emerging distributed learning paradigm, which enables multiple parties to jointly train a shared machine learning (ML) model without causing the disclosure of the raw data of each party. As one of the fundamental collaborative learning algorithms, privacy-preserving collaborative logistic regression has recently gained attention from industry and academia, which utilizes cryptographic techniques to securely train joint logistic regression models across data from multiple parties. However, existing schemes have high communication and computational overhead, lose the ability to deal with high-dimensional sparse samples, cut down the accuracy of the model, or exist the risk of leaking private information. To overcome these issues, considering vertically distributed data, we propose a privacy-preserving vertical collaborative logistic regression (P-2 VCLR) based on approximate homomorphic encryption (HE), which enables two parties to jointly train a shared model without a trusted third-party coordinator. Our scheme utilizes batching method in approximate HE to encrypt multiple data into a single ciphertext and enable a parallel processing through single instruction multiple data (SIMD) manner. We evaluate our scheme by using three publicly available datasets, the experimental results indicate that our scheme outperforms existing schemes in terms of training time and model performance.
更多查看译文
关键词
privacy-preserving,third-party
AI 理解论文
溯源树
样例
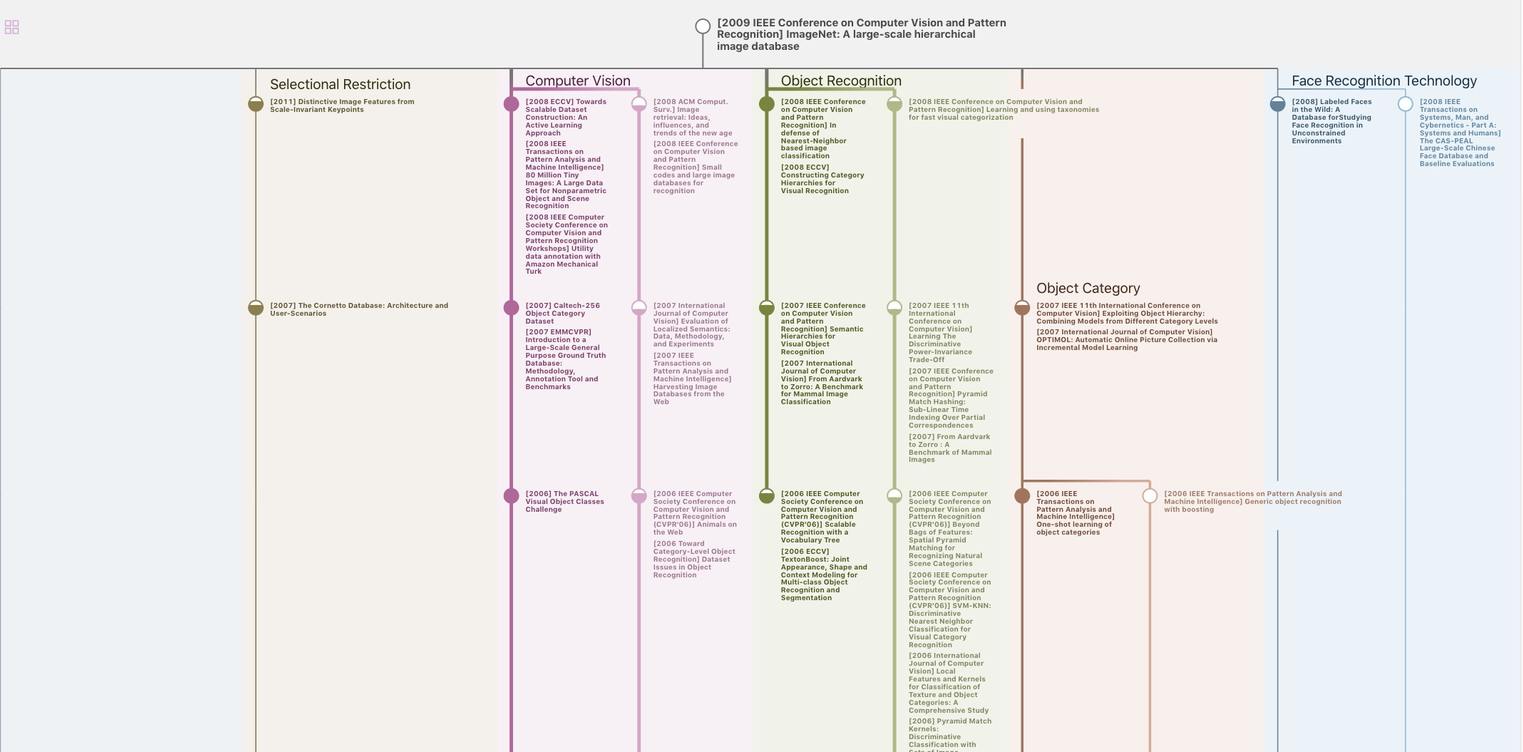
生成溯源树,研究论文发展脉络
Chat Paper
正在生成论文摘要