Time-frequency feature transform suite for deep learning-based gesture recognition using sEMG signals
ROBOTICA(2023)
摘要
Recently, deep learning methods have achieved considerable performance in gesture recognition using surface electromyography signals. However, improving the recognition accuracy in multi-subject gesture recognition remains a challenging problem. In this study, we aimed to improve recognition performance by adding subject-specific prior knowledge to provide guidance for multi-subject gesture recognition. We proposed a time-frequency feature transform suite (TFFT) that takes the maps generated by continuous wavelet transform (CWT) as input. The TFFT can be connected to a neural network to obtain an end-to-end architecture. Thus, we integrated the suite into traditional neural networks, such as convolutional neural networks and long short-term memory, to adjust the intermediate features. The results of comparative experiments showed that the deep learning models with the TFFT suite based on CWT improved the recognition performance of the original architectures without the TFFT suite in gesture recognition tasks. Our proposed TFFT suite has promising applications in multi-subject gesture recognition and prosthetic control.
更多查看译文
关键词
sEMG,gesture recognition,deep learning,neural networks,TFFT suite
AI 理解论文
溯源树
样例
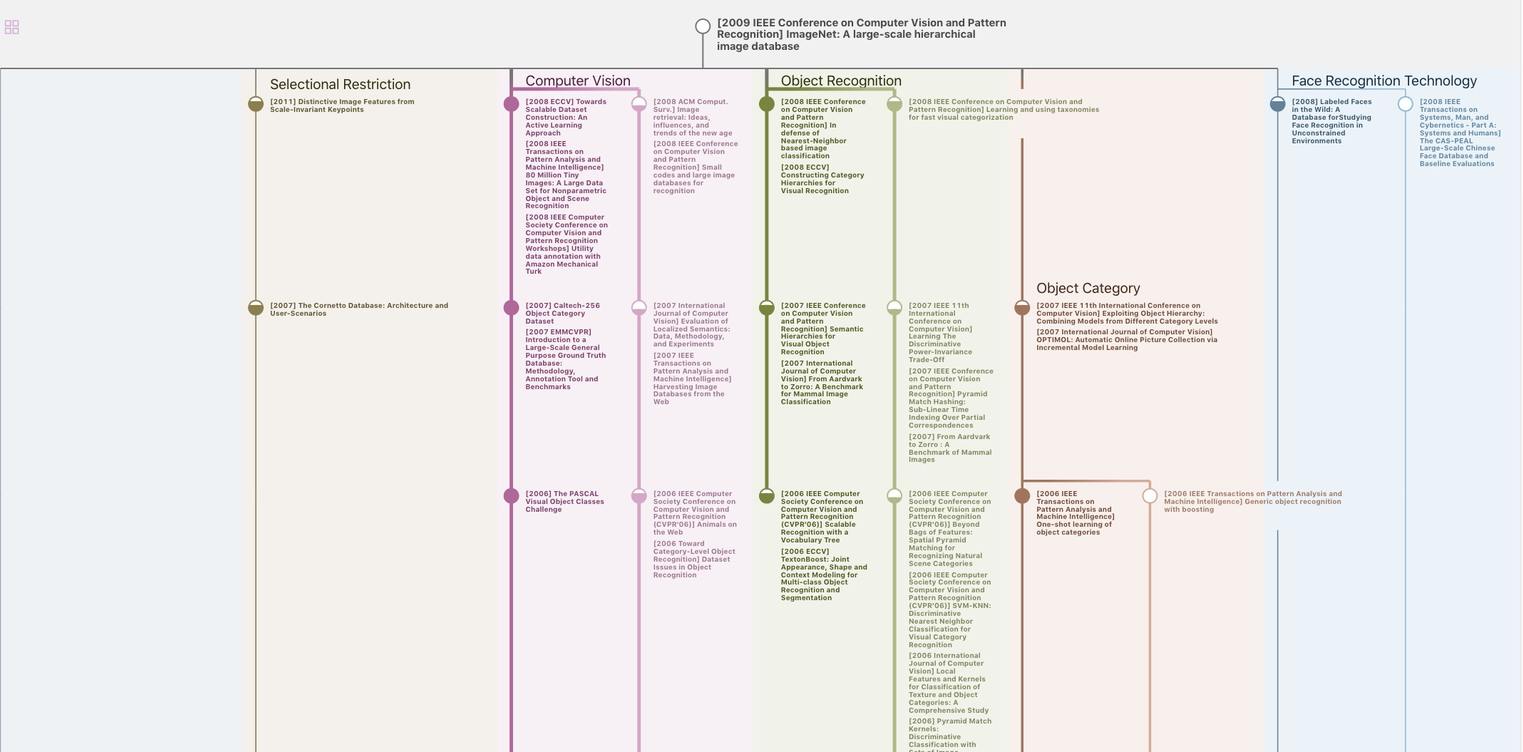
生成溯源树,研究论文发展脉络
Chat Paper
正在生成论文摘要