Clustering-Based Cross-Sectional Regime Identification for Financial Market Forecasting
DATABASE AND EXPERT SYSTEMS APPLICATIONS, DEXA 2022, PT II(2022)
摘要
Regime switching analysis is extensively advocated in many fields to capture complex behaviors underlying an ecosystem, such as the economic or financial system. A regime can be defined as a specific group of complex patterns that share common characteristics in a specific time interval. Regime switch, caused by external and/or internal drivers, refers to the changing behaviors exhibited by a system at a specific time point. The existing regime detection methods suffer from two drawbacks: they lack the capability to identify new regimes dynamically or they ignore the cross-sectional dependencies exhibited by time series data for the forecasting. This promoted us to devise a cluster-based regime identification model that can identify cross-sectional regimes dynamically with a time-varying transition probability, and capture cross-sectional dependencies underlying financial time series for market forecasting. Our approach makes use of a nonlinear model to account for the cross-sectional regime dependencies, neglected by most existing studies, that can improve the performance of a forecasting model significantly. Experimental results on both synthetic and real-world dataset demonstrate that our model outperforms state-of-the-art forecasting models.
更多查看译文
关键词
Regime switch analysis, Cross-sectional regime identification, Financial market forecasting
AI 理解论文
溯源树
样例
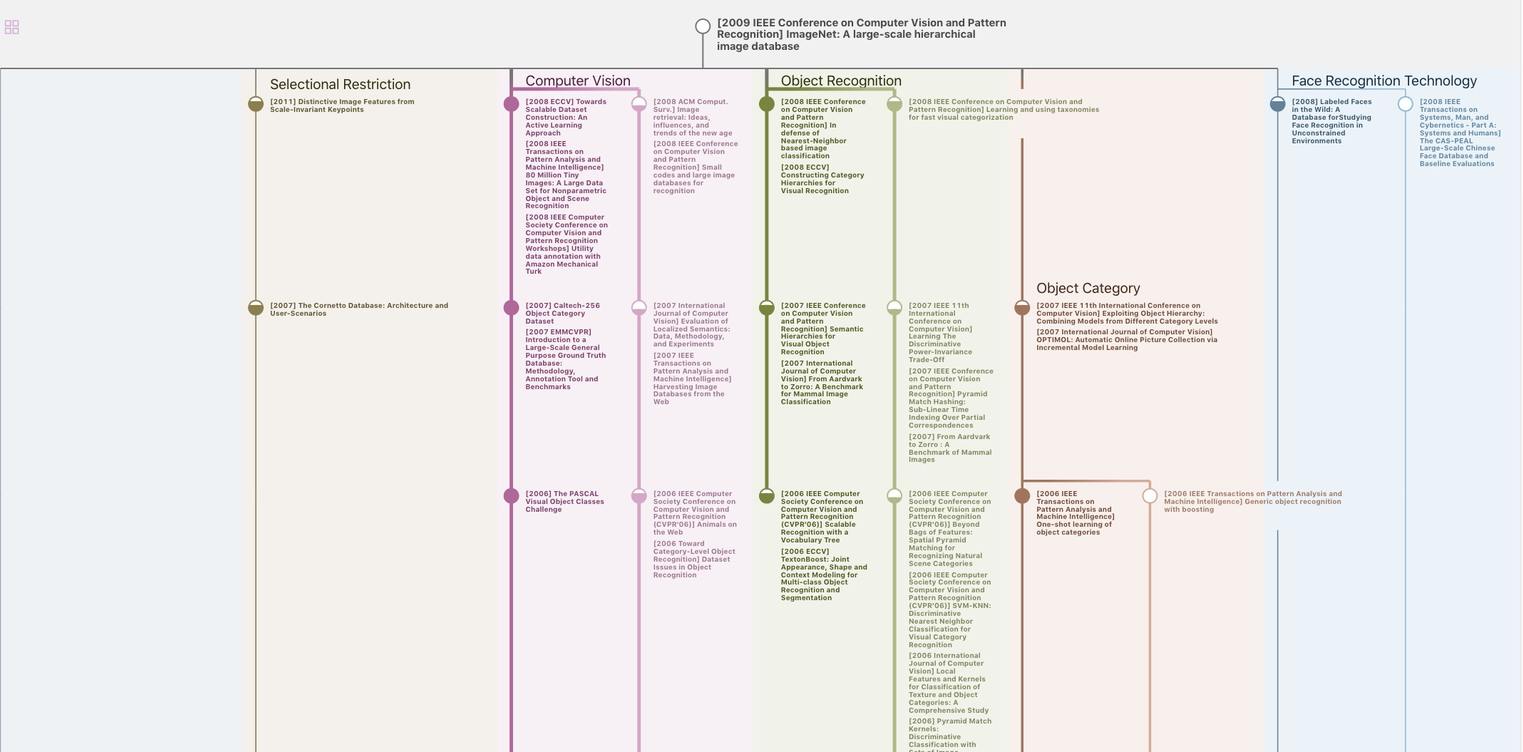
生成溯源树,研究论文发展脉络
Chat Paper
正在生成论文摘要