A bearing fault diagnosis method without fault data in new working condition combined dynamic model with deep learning
ADVANCED ENGINEERING INFORMATICS(2022)
摘要
Bearing fault diagnosis plays an important role in rotating machinery equipment's safe and stable operation. However, the fault sample collected from the equipment is seriously absent, which obstacles the establishment of the diagnostic model. In this paper, a novel false-real data synthesis method combined bearing dynamic model with a generated adversarial network is proposed to solve the problem of zero-shot in new condition. Firstly, the bearing dynamic model is constructed to simulate vibration data in different conditions. Secondly, the conver-sion model is trained by simulation data in different conditions, which will be employed to convert real-world data in the old condition into the conversion data in the new condition. Thirdly, the GAN model is trained by simulation data and real-world data in old condition and finetuned by simulation data and conversion data in the new condition. Finally, simulation data in the new condition are inputted to the finetuned GAN model to obtain generated data in the new condition, and the fault diagnosis model is trained by it. To validate the performance of the proposed method, various comparative experiments are carried out on one rolling bearing dataset. The results indicate that the proposed method can solve the problem of zero-shot in new condition with excellent diagnosis performance.
更多查看译文
关键词
Fault diagnosis, Bearing dynamic model, Generative adversarial network, Fault order conversion, Zero-shot in new condition
AI 理解论文
溯源树
样例
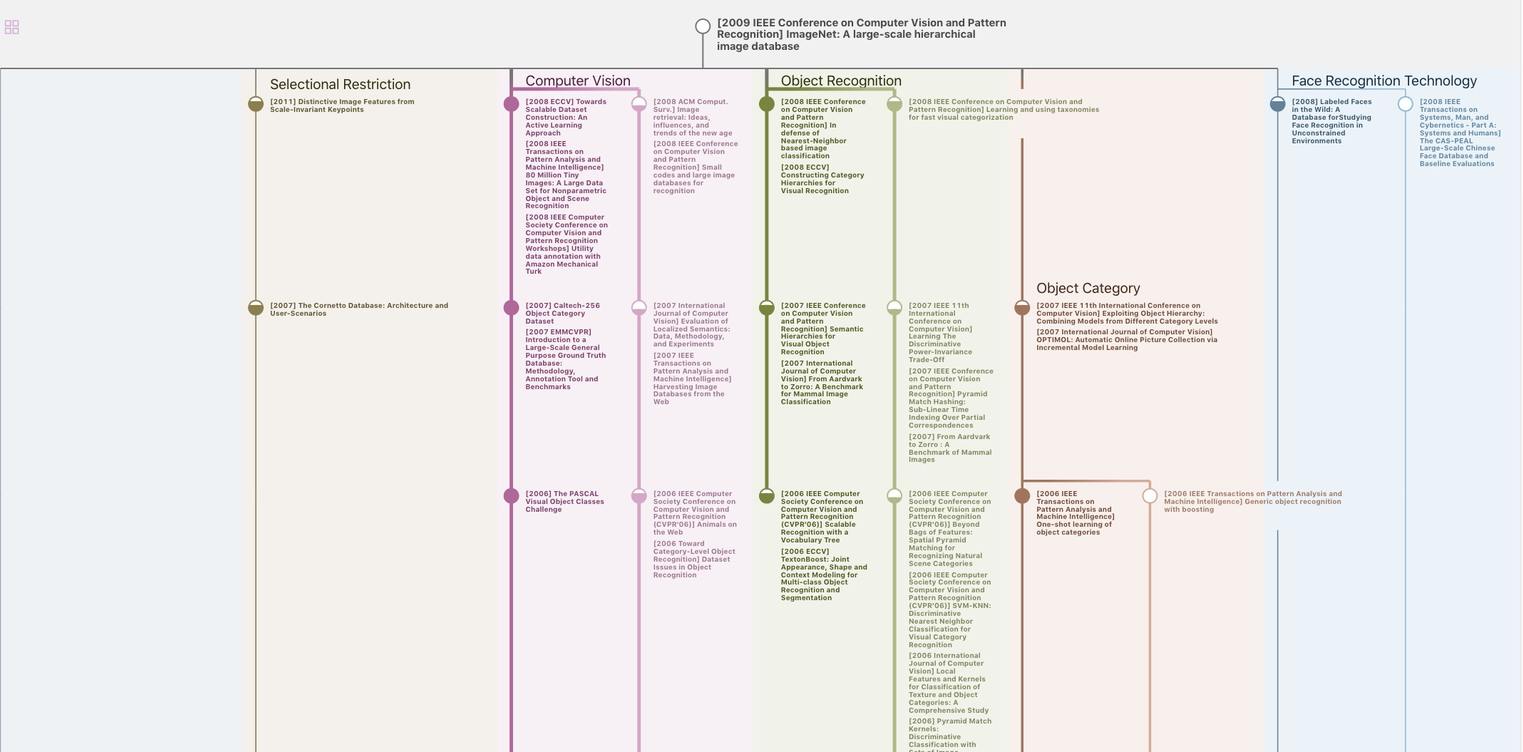
生成溯源树,研究论文发展脉络
Chat Paper
正在生成论文摘要