Ultrafast inverse design of quantum dot optical spectra via a joint TD-DFT learning scheme and deep reinforcement learning
AIP ADVANCES(2022)
摘要
Here, we report a case study on inverse design of quantum dot optical spectra using a deep reinforcement learning algorithm for the desired target optical property of semiconductor CdxSeyTex-y quantum dots. Machine learning models were trained to predict the optical absorption and emission spectra by using the training dataset by time dependent density functional theory simulation. We show that the trained deep deterministic policy gradient inverse design agent can infer the molecular structure with an accuracy of less than 1 angstrom at a fixed computational time of milliseconds and up to 100-1000 times faster than the conventional heuristic particle swam optimization method. Most of the effective inverse design problems based on the surrogate machine learning and reinforcement learning model have been focused on the field of nano-photonics. Few attempts have been made in the field of quantum optical system in a similar manner. For the first time, our results, to our knowledge, provide concrete evidence that for computationally challenging tasks, a well-trained deep reinforcement learning agent can replace the existing quantum simulation and heuristics optimization tool, enabling fast and scalable simulations of the optical property of nanometer sized semiconductor quantum dots. (C) 2022 Author(s).
更多查看译文
AI 理解论文
溯源树
样例
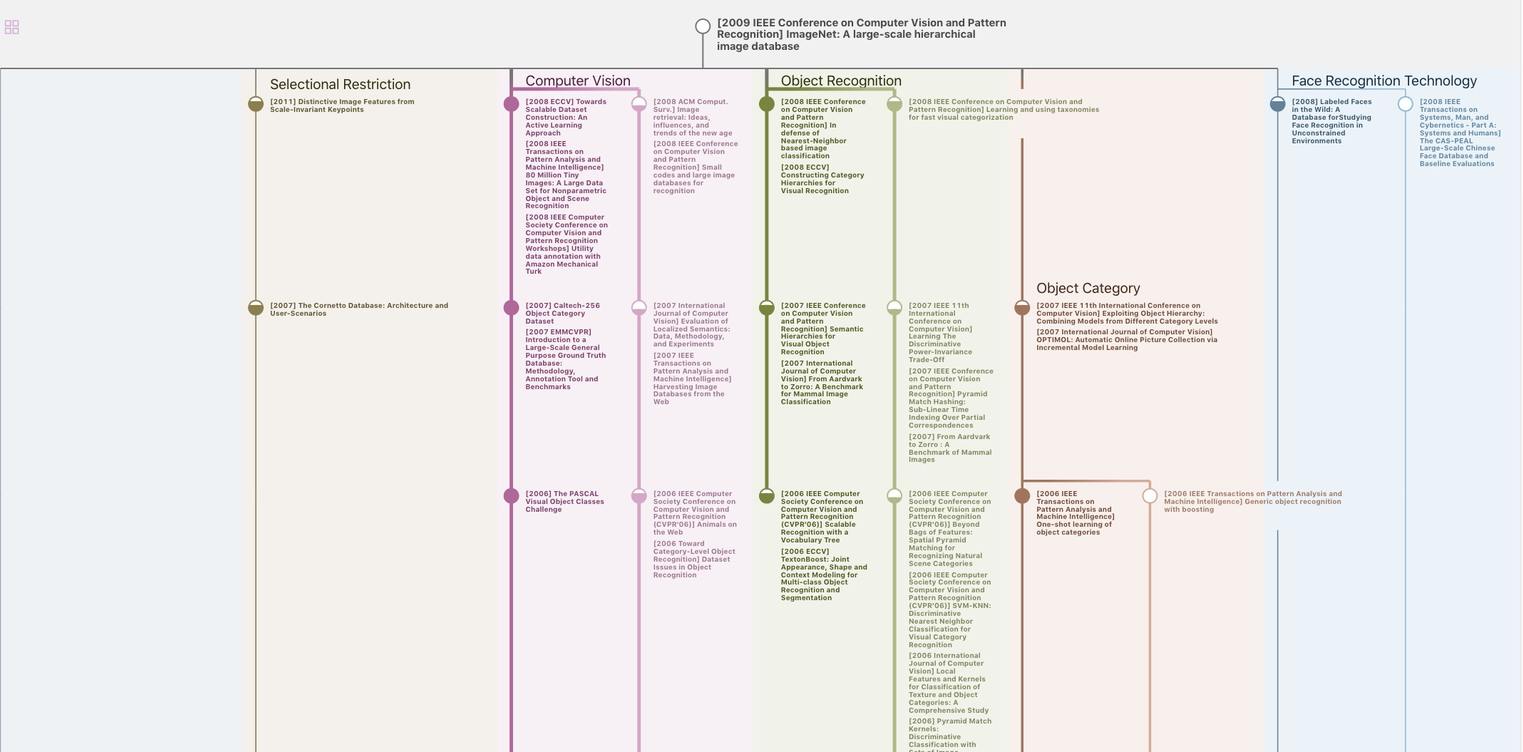
生成溯源树,研究论文发展脉络
Chat Paper
正在生成论文摘要