Layer-fusion for online mutual knowledge distillation
MULTIMEDIA SYSTEMS(2022)
摘要
Online knowledge distillation opens a door for distillation on parallel student networks, which breaks the heavy reliance upon the pre-trained teacher model. The additional feature fusion solutions further provide positive training loop among parallel student networks. However, current feature fusion operation is always set at the end of sub-networks, thus its capability is limited. In this paper, we propose a novel online knowledge distillation approach by designing multiple layer-level feature fusion modules to connect sub-networks, which contributes to triggering mutual learning among student networks. For model training, fusion modules of middle layers are regarded as auxiliary teachers, while the fusion module at the end of sub-networks is used as the ensemble teacher. Each sub-network is optimized under the supervision of two kinds of knowledge transmitted by different teachers. Furthermore, the attention learning is adopted to enhance feature representation in fusion modules applied to middle layers, which assists to obtain representative features. Extensive evaluations are performed on CIFAR10/CIFAR100, and ImageNet2012 datasets, and experiment results exhibit outstanding performance of our proposed approach.
更多查看译文
关键词
Online learning,Knowledge distillation,Feature fusion,Mutual learning
AI 理解论文
溯源树
样例
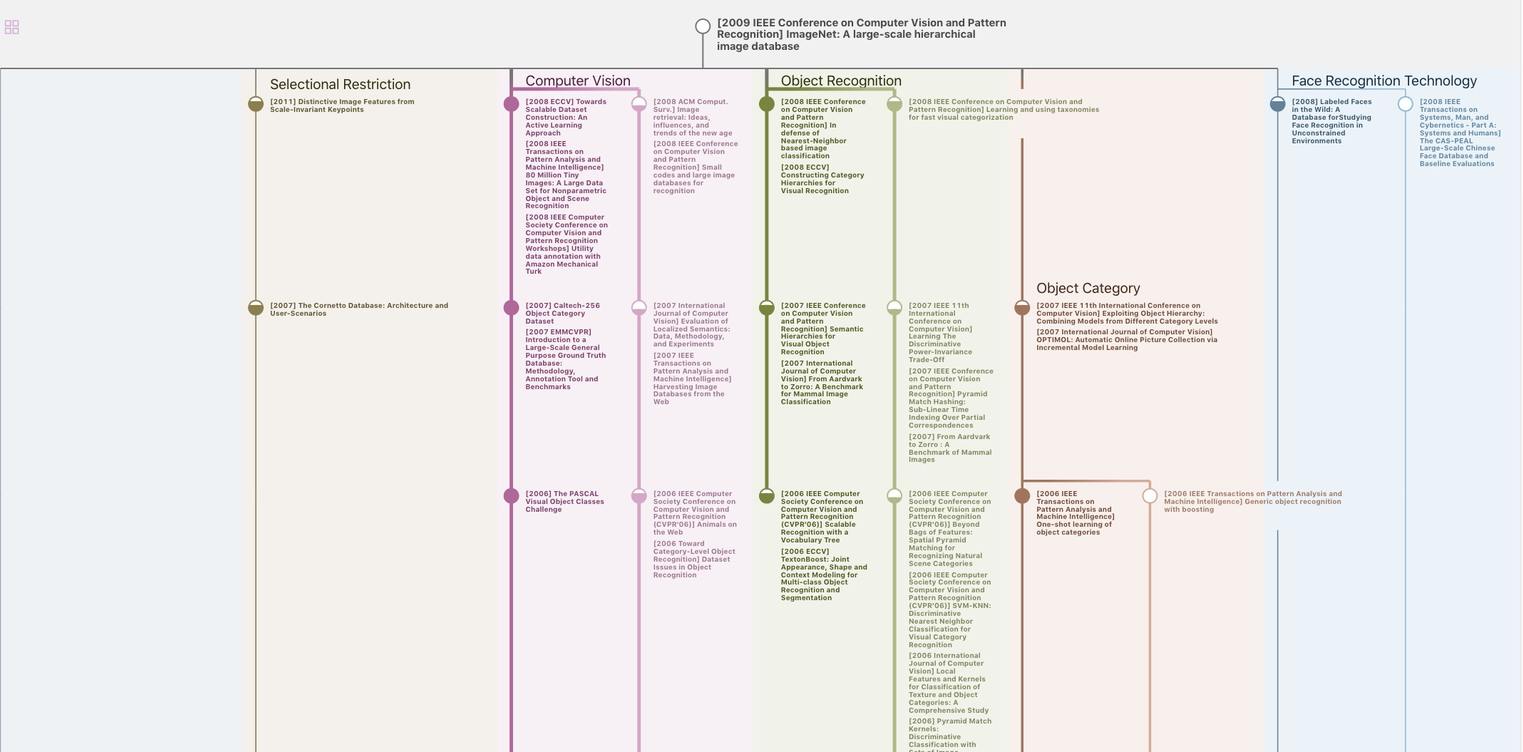
生成溯源树,研究论文发展脉络
Chat Paper
正在生成论文摘要