Multi-source domains transfer learning strategy based on similarity measurement for batch process quality prediction
CANADIAN JOURNAL OF CHEMICAL ENGINEERING(2023)
摘要
Generally, when multiple source processes are available for transfer modelling, an inappropriate transfer learning strategy may lead to the deterioration of model accuracy and even cause negative transfer. For this issue, a multi-source domains transfer learning strategy based on similarity measurement is proposed in this work for selecting the optimal transfer modelling strategy according to different scenarios. By counting the data amount and judging the similarity between each source domain and the target domain, the rich information contained in multiple source processes is well utilized to assist the modelling process in the target domain. The proposed strategy provides three alternative specific transfer mechanisms by setting the judgement conditions so that the possibility of negative transfer can get reduced and the accuracy of prediction can be guaranteed. To ensure the reliability of the transfer model and adapt to the change in conditions, the updating scheme based on online data is adopted. Finally, the mixed-effects Gaussian processes modelling method is taken as an example to verify the effectiveness of the proposed multi-source transfer learning strategy through the simulation experiment of the cobalt oxalate synthesis process.
更多查看译文
关键词
batch process,multi-source domains,quality prediction,transfer learning strategy
AI 理解论文
溯源树
样例
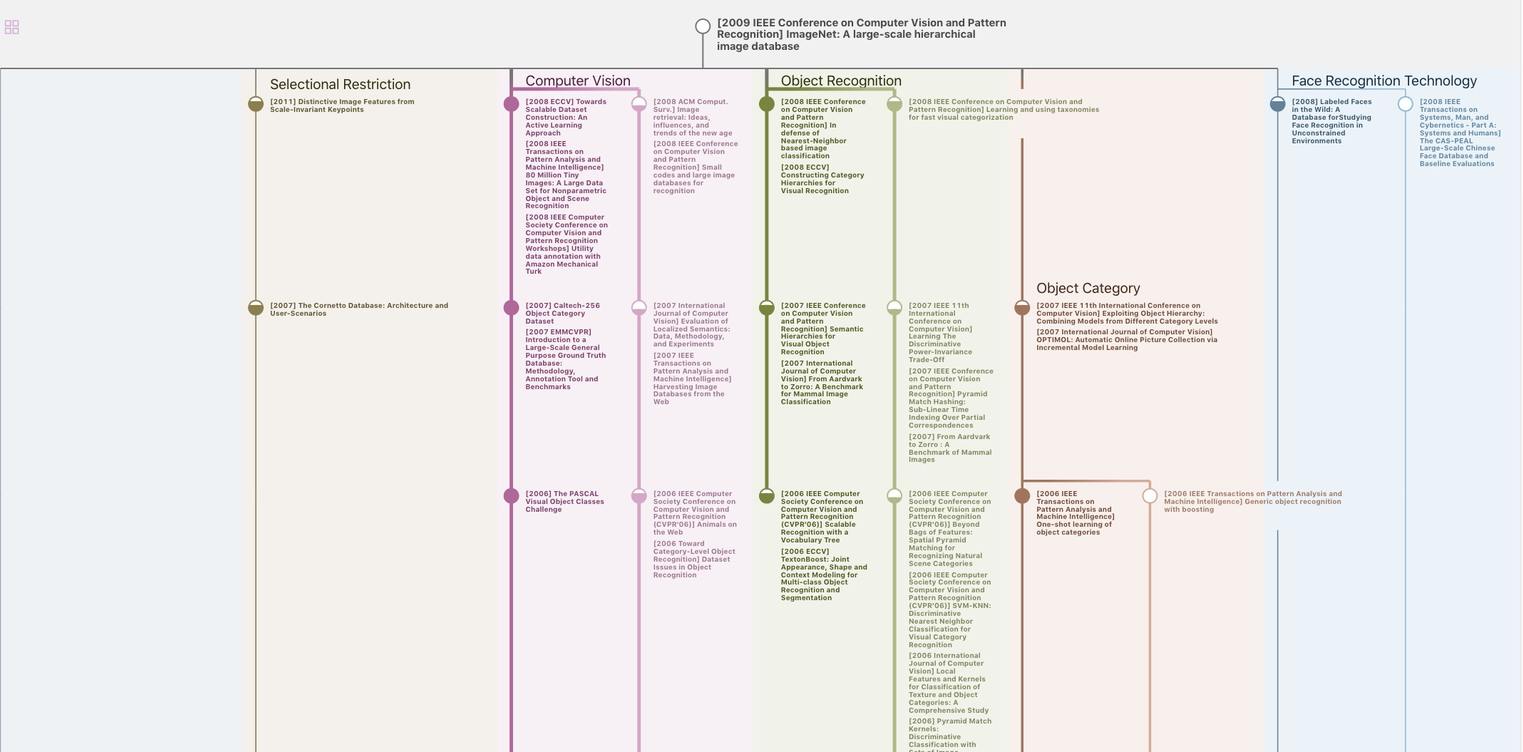
生成溯源树,研究论文发展脉络
Chat Paper
正在生成论文摘要