High-Cardinality Categorical Attributes and Credit Card Fraud Detection
MATHEMATICS(2022)
摘要
Credit card transactions may contain some categorical attributes with large domains, involving up to hundreds of possible values, also known as high-cardinality attributes. The inclusion of such attributes makes analysis harder, due to results with poorer generalization and higher resource usage. A common practice is, therefore, to ignore such attributes, removing them, albeit wasting the information they provided. Contrariwise, this paper reports our findings on the positive impacts of using high-cardinality attributes on credit card fraud detection. Thus, we present a new algorithm for domain reduction that preserves the fraud-detection capabilities. Experiments applying a deep feedforward neural network on real datasets from a major Brazilian financial institution have shown that, when measured by the F-1 metric, the inclusion of such attributes does improve fraud-detection quality. As a main contribution, this proposed algorithm was able to reduce attribute cardinality, improving the training times of a model while preserving its predictive capabilities.
更多查看译文
关键词
credit card fraud, fraud-detection system, high-cardinality attribute, pattern recognition, clustering, deep learning
AI 理解论文
溯源树
样例
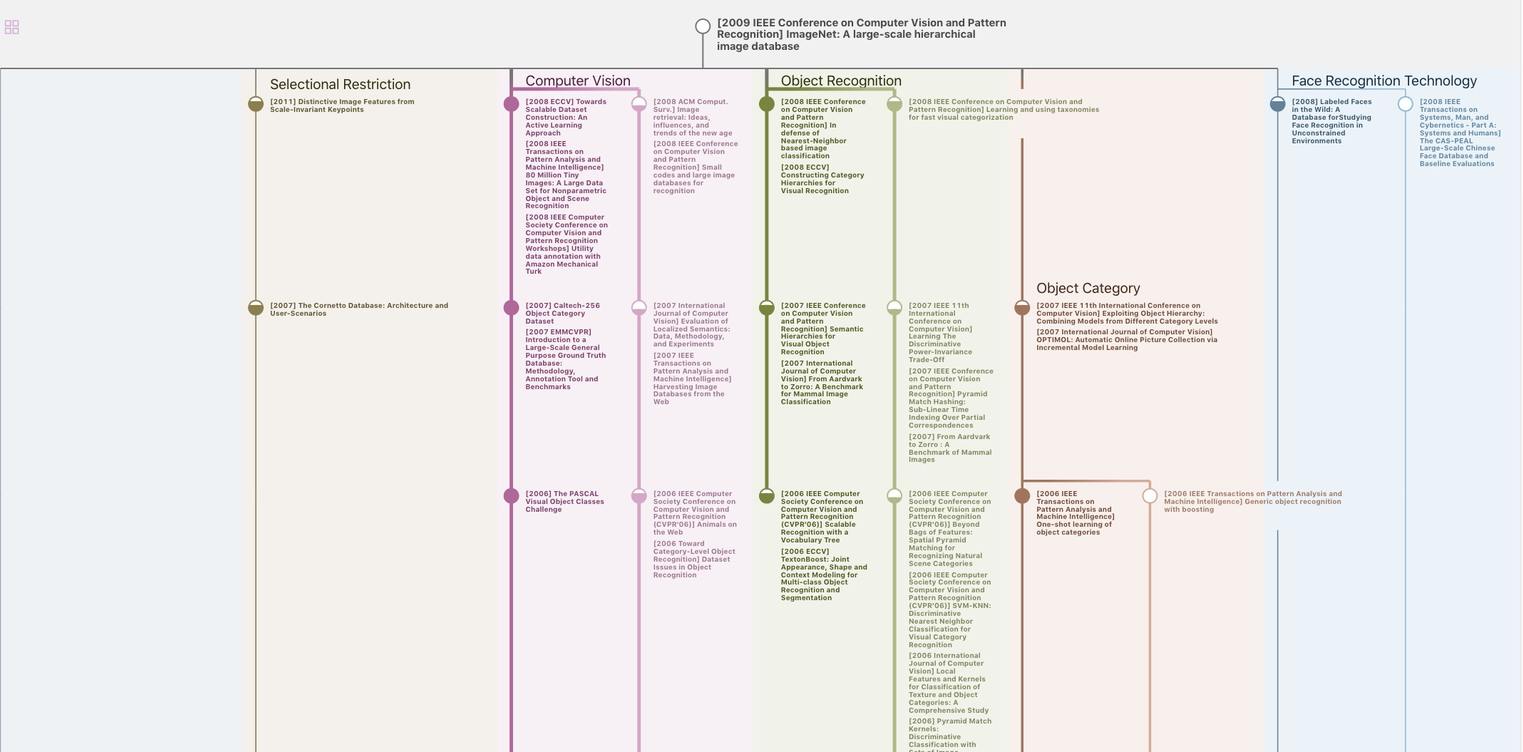
生成溯源树,研究论文发展脉络
Chat Paper
正在生成论文摘要