Time Series Attention Based Transformer Neural Turing Machines for Diachronic Graph Embedding in Cyber Threat Intelligence
COMPUTATIONAL SCIENCE, ICCS 2022, PT IV(2022)
摘要
The cyber threats are often found to threaten individuals, organizations and countries at different levels and evolve continuously over time. Cyber Threat Intelligence (CTI) is an effective approach to solve cyber security problems. However, existing processes are considered inherent responses to known threats. CTI experts recommend proactively checking for emerging threats in existing knowledge. In addition, most researches focus on static snapshots of the CTI knowledge graph, while ignoring the temporal dynamics. To this end, we create a novel framework TSA-TNTM (Time Series Attention based Transformer Neural Turing Machines) for diachronic graph embedding framework, which uses time series self-attention mechanism to capture the non-linearly evolving entity representations over time. We demonstrate significantly improved performance over various approaches. A series of benchmark experiments illustrate that TSA-TNTM could generate higher quality than the state-of-the-art word embedding models in tasks pertaining to semantic analogy, clustering, threat classification and proactively identify emerging threats in CTI fields.
更多查看译文
关键词
Threat intelligence, Dynamic knowledge graph, Time series attention, Graph embedding
AI 理解论文
溯源树
样例
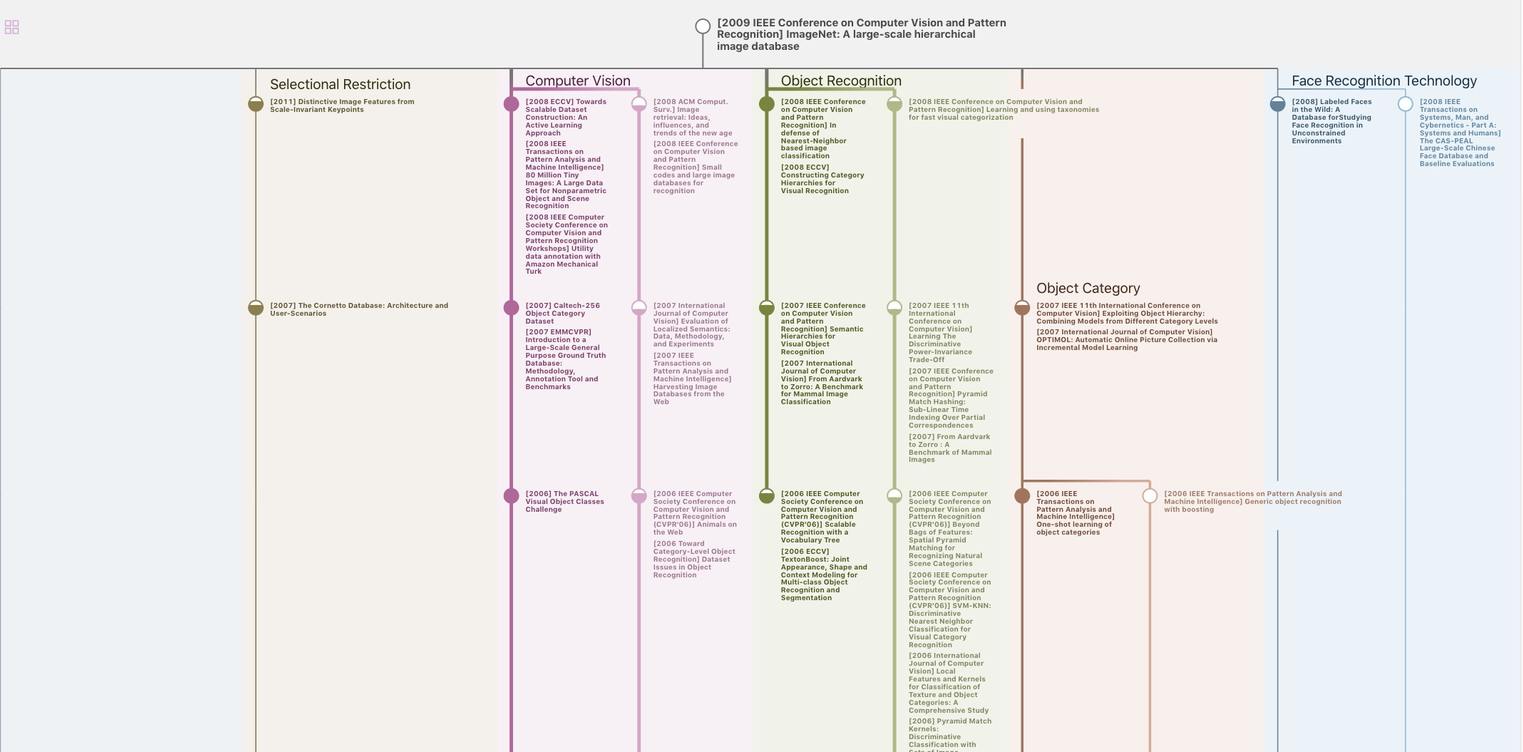
生成溯源树,研究论文发展脉络
Chat Paper
正在生成论文摘要