56 analysis of a 23,270-patient study: unsupervised machine learning with cluster analysis in patients discharged after an acute coronary syndrome
European Heart Journal Supplements(2022)
摘要
Abstract Background Characterization and management of patients with acute coronary syndromes (ACS) remain challenging, and it is unclear whether currently available clinical and procedural features can suffice to inform adequate decision making. Methods Details on patients discharged after an ACS were obtained by querying an extensive multicenter registry, detailing patient features as well as management details. Clinical outcomes included fatal and non-fatal cardiovascular events at 1-year follow-up. After missing data imputation, two unsupervised machine learning approaches (k-means and Clustering Large Applications [CLARA]) were used to generate separate clusters with different features. Bivariate and multivariable-adjusted analyses were performed to compare different clusters for clinical outcomes. Findings: 23,270 patients discharged after ACS were included. Two clusters were identified by k-mean algorithm (k1 and k2), and two clusters by CLARA algorithm (C1 and C2). Differences in 2-years outcomes between k1 and k2 and between C1 and C2 were substantial. K2 cluster (N=21,988) in comparison with k1 cluster (N=1,282) had significantly higher occurrence of death (9.5% vs 3.8%, p<0.001), reinfarction (7.2% vs 3.7%, p<0.001), and major bleeding (6.0% vs 3.0%, p<0.001). Similarly, C1 cluster (N=11,268) showed a worse prognosis than C2 cluster (N=12,002): death (4.8% vs 3.5%, p<0.001), reinfarction (4.5% vs 3.4%, p<0.001), and major bleeding (3.6% vs 2.8%, p=0.001). Most associations did not hold at multivariable analysis based on supervised learning techniques, with the exception of major bleeding (odds ratio=1.37 [95% confidence interval 1.02-1.83] for the k1/C1 subcluster vs k1/C2 subcluster, p=0.039. Conclusions A machine-learning based clustering approach is effective at face value to inform on the prognosis of patients with ACS managed invasively. These findings can be leveraged to inform decision-making in this setting, but also highlight the potential role of cluster analysis in first-in-man, and preapproval studies of medical devices.
更多查看译文
关键词
unsupervised machine learning,cluster analysis,acute coronary syndrome,machine learning
AI 理解论文
溯源树
样例
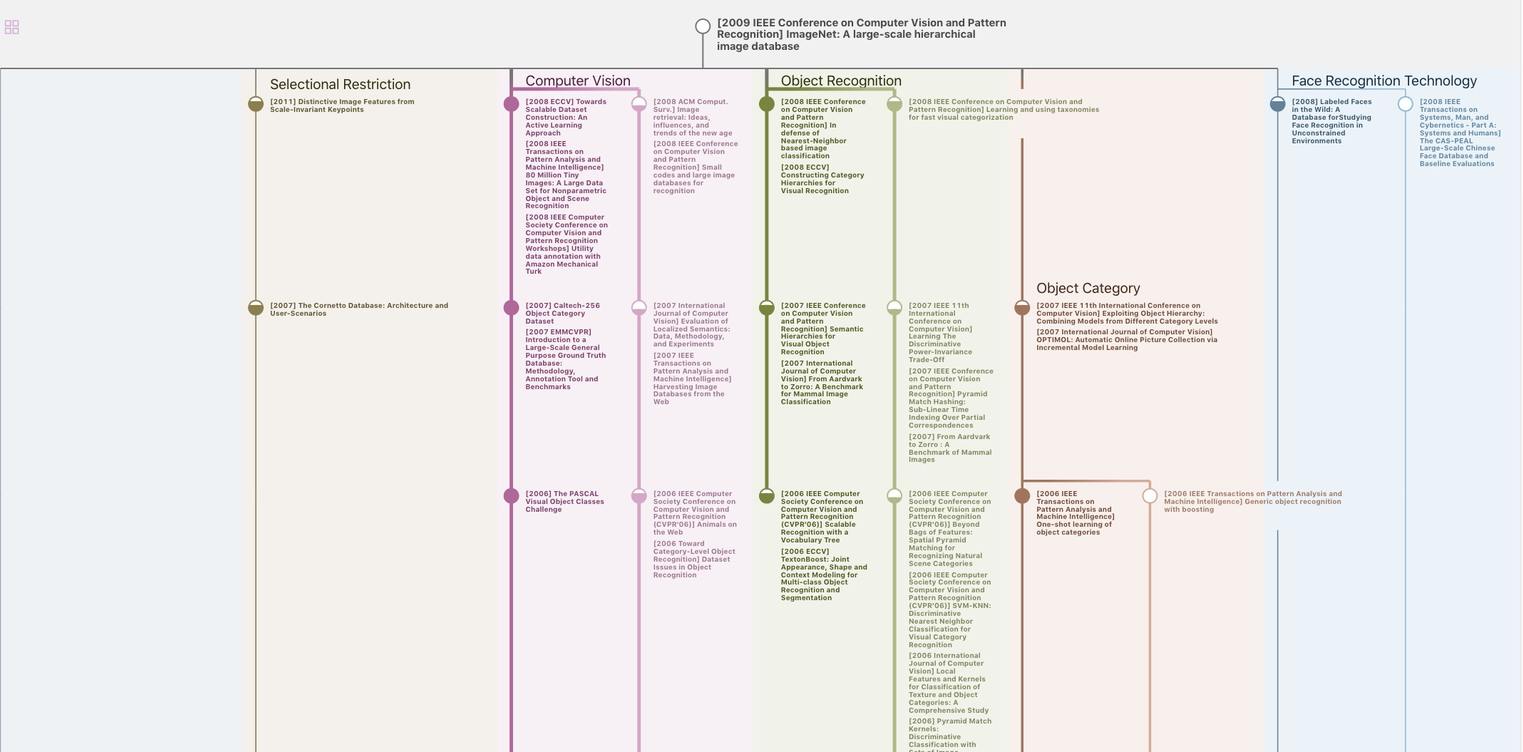
生成溯源树,研究论文发展脉络
Chat Paper
正在生成论文摘要