MASTER: Multi-task Pre-trained Bottlenecked Masked Autoencoders Are Better Dense Retrievers
MACHINE LEARNING AND KNOWLEDGE DISCOVERY IN DATABASES: RESEARCH TRACK, ECML PKDD 2023, PT II(2023)
摘要
Pre-trained Transformers (e.g., BERT) have been commonly used in existing dense retrieval methods for parameter initialization, and recent studies are exploring more effective pre-training tasks for further improving the quality of dense vectors. Although various novel and effective tasks have been proposed, their different input formats and learning objectives make them hard to be integrated for jointly improving the model performance. In this work, we aim to unify a variety of pre-training tasks into the bottlenecked masked autoencoder manner, and integrate them into a multi-task pre-trained model, namely MASTER. Concretely, MASTER utilizes a shared-encoder multi-decoder architecture that can construct a representation bottleneck to compress the abundant semantic information across tasks into dense vectors. Based on it, we integrate three types of representative pre-training tasks: corrupted passages recovering, related passages recovering and PLMs outputs recovering, to characterize the inner-passage information, inter-passage relations and PLMs knowledge. Extensive experiments have shown that our approach outperforms competitive dense retrieval methods. Our code and data are publicly released in https://github.com/microsoft/SimXNS.
更多查看译文
关键词
Dense Retrieval,Pre-training,Multi-task Learning
AI 理解论文
溯源树
样例
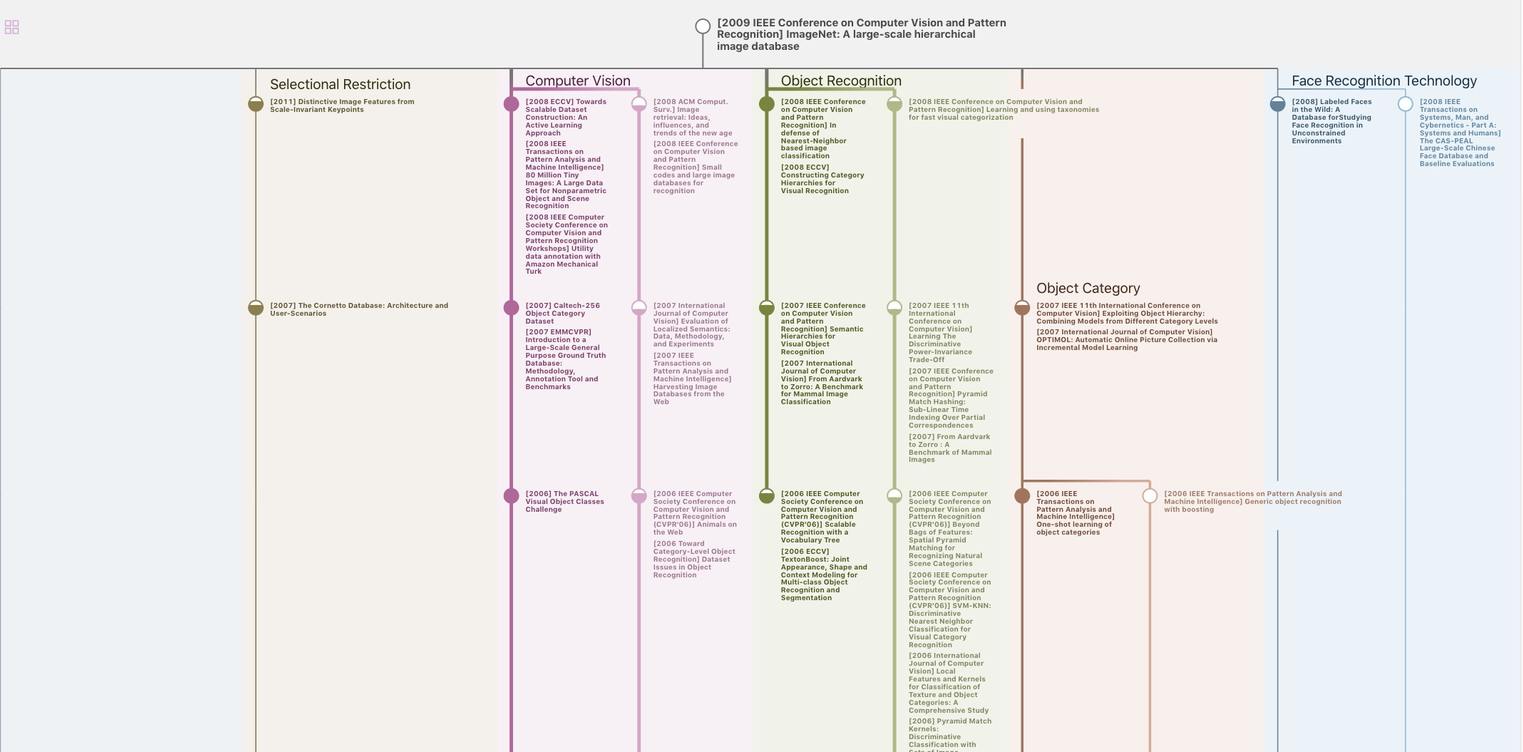
生成溯源树,研究论文发展脉络
Chat Paper
正在生成论文摘要