Efficient Self-supervised Learning with Contextualized Target Representations for Vision, Speech and Language
arxiv(2022)
摘要
Current self-supervised learning algorithms are often modality-specific and require large amounts of computational resources. To address these issues, we increase the training efficiency of data2vec, a learning objective that generalizes across several modalities. We do not encode masked tokens, use a fast convolutional decoder and amortize the effort to build teacher representations. data2vec 2.0 benefits from the rich contextualized target representations introduced in data2vec which enable a fast self-supervised learner. Experiments on ImageNet-1K image classification show that data2vec 2.0 matches the accuracy of Masked Autoencoders in 16.4x lower pre-training time, on Librispeech speech recognition it performs as well as wav2vec 2.0 in 10.6x less time, and on GLUE natural language understanding it matches a retrained RoBERTa model in half the time. Trading some speed for accuracy results in ImageNet-1K top-1 accuracy of 86.8\% with a ViT-L model trained for 150 epochs.
更多查看译文
关键词
contextualized target
AI 理解论文
溯源树
样例
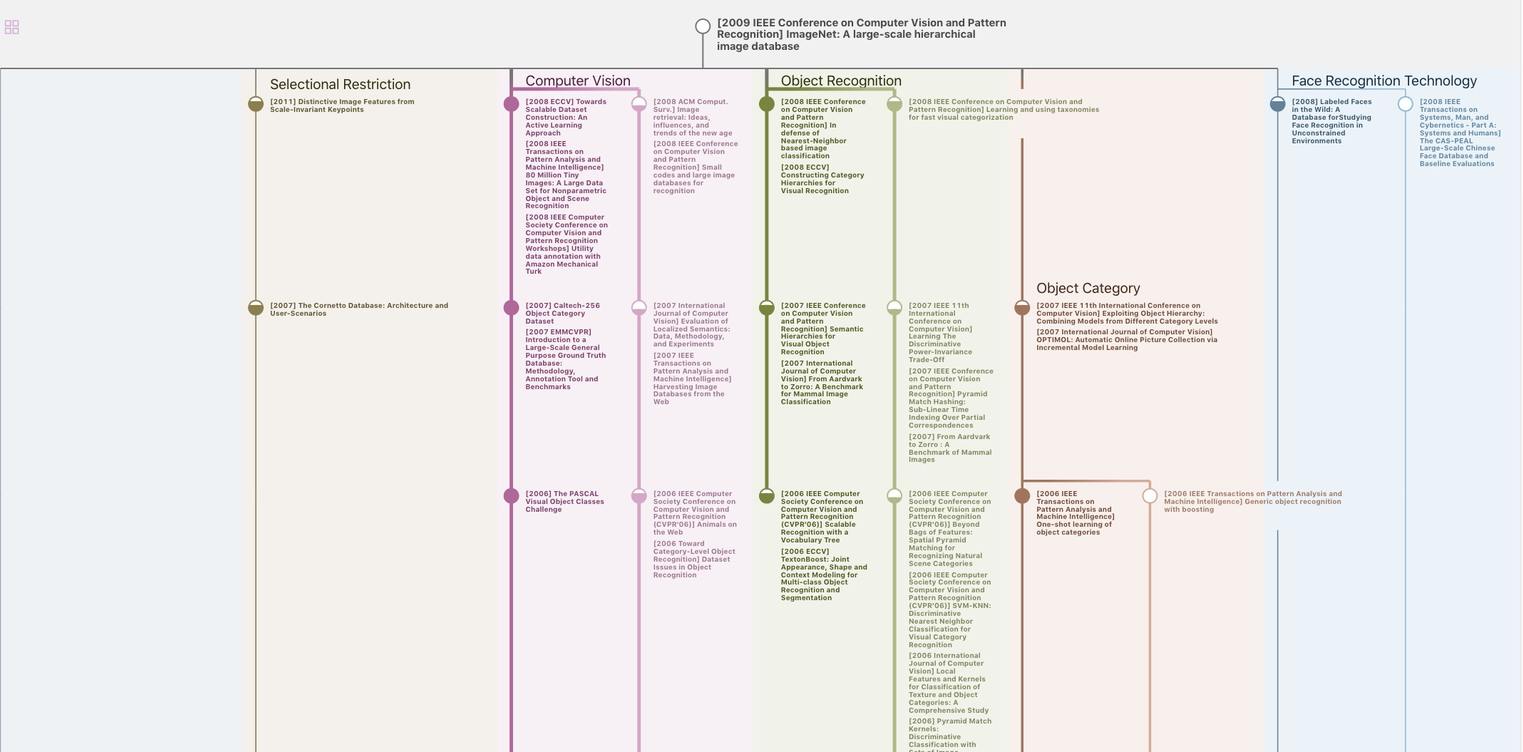
生成溯源树,研究论文发展脉络
Chat Paper
正在生成论文摘要