Target Selection and Sample Characterization for the DESI LOW-Z Secondary Target Program
arxiv(2022)
摘要
We introduce the DESI LOW-Z Secondary Target Survey, which combines the wide area capabilities of the Dark Energy Spectroscopic Instrument (DESI) with an efficient, low-redshift target selection method. Our selection consists of a set of color and surface brightness cuts, combined with modern machine learning methods, to optimally target low-redshift dwarf galaxies (z < 0.03) between 19 < r < 21. We employ a convolutional neural network (CNN) to select high priority targets. Our data validation shows that the CNN can achieve 90% completeness at z < 0.03 with 20% efficiency at selecting low-redshift galaxies, compared to compared to efficiencies of ~1% using traditional photometric methods. The LOW-Z survey has already obtained over 22,000 redshifts of dwarf galaxies ($M_*$ < $10^9$ $M_\odot$), comparable to the number of dwarf galaxies discovered in SDSS and GAMA. As a spare fiber survey, LOW-Z currently receives fiber allocation for just ~30% of its targets. However, we estimate that our photometric cuts are > 95% complete in target selection at z < 0.03 between 19 更多
查看译文
关键词
secondary target program,selection,sample characterization
AI 理解论文
溯源树
样例
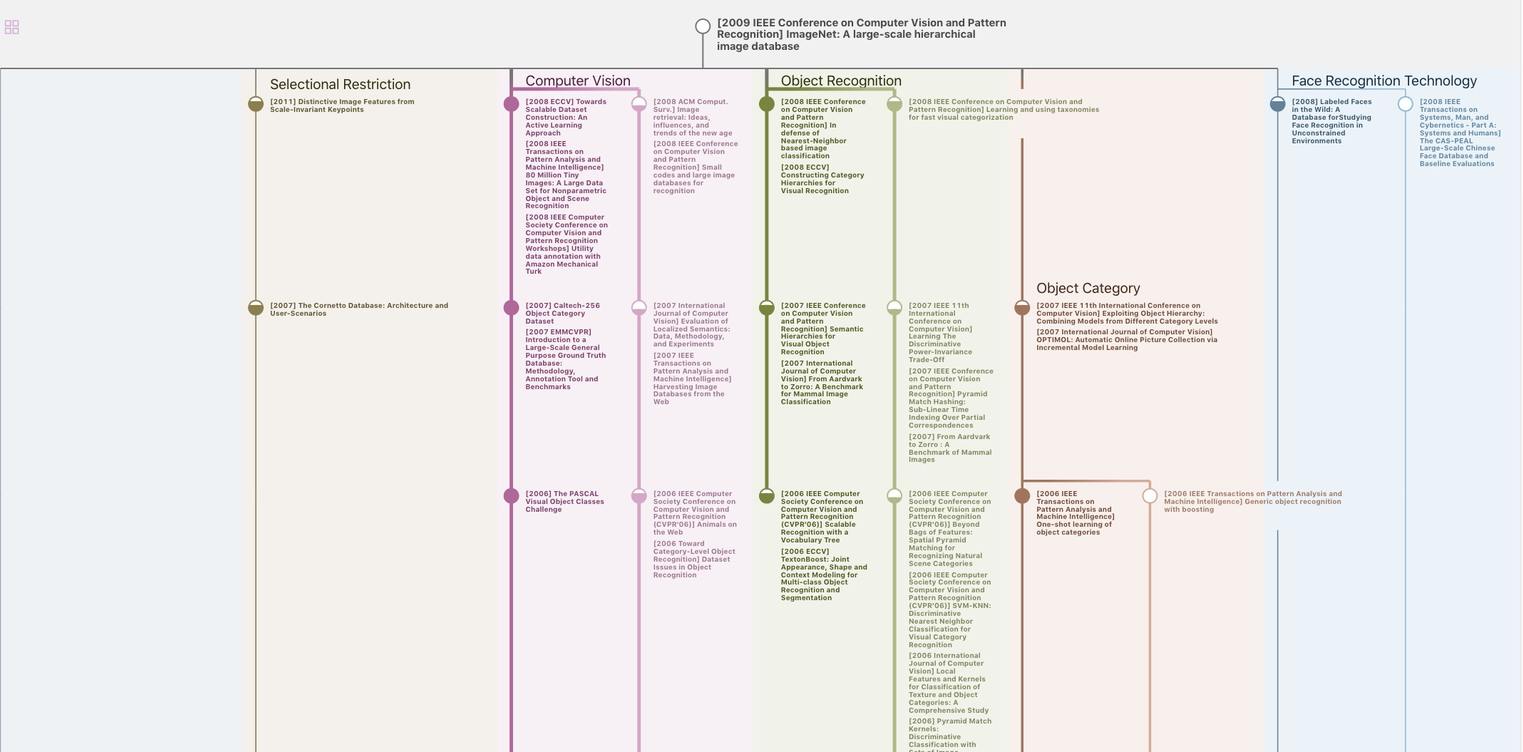
生成溯源树,研究论文发展脉络
Chat Paper
正在生成论文摘要