MIST: a Large-Scale Annotated Resource and Neural Models for Functions of Modal Verbs in English Scientific Text
arxiv(2022)
摘要
Modal verbs (e.g., "can", "should", or "must") occur highly frequently in scientific articles. Decoding their function is not straightforward: they are often used for hedging, but they may also denote abilities and restrictions. Understanding their meaning is important for various NLP tasks such as writing assistance or accurate information extraction from scientific text. To foster research on the usage of modals in this genre, we introduce the MIST (Modals In Scientific Text) dataset, which contains 3737 modal instances in five scientific domains annotated for their semantic, pragmatic, or rhetorical function. We systematically evaluate a set of competitive neural architectures on MIST. Transfer experiments reveal that leveraging non-scientific data is of limited benefit for modeling the distinctions in MIST. Our corpus analysis provides evidence that scientific communities differ in their usage of modal verbs, yet, classifiers trained on scientific data generalize to some extent to unseen scientific domains.
更多查看译文
关键词
modal verbs,neural models,text,large-scale
AI 理解论文
溯源树
样例
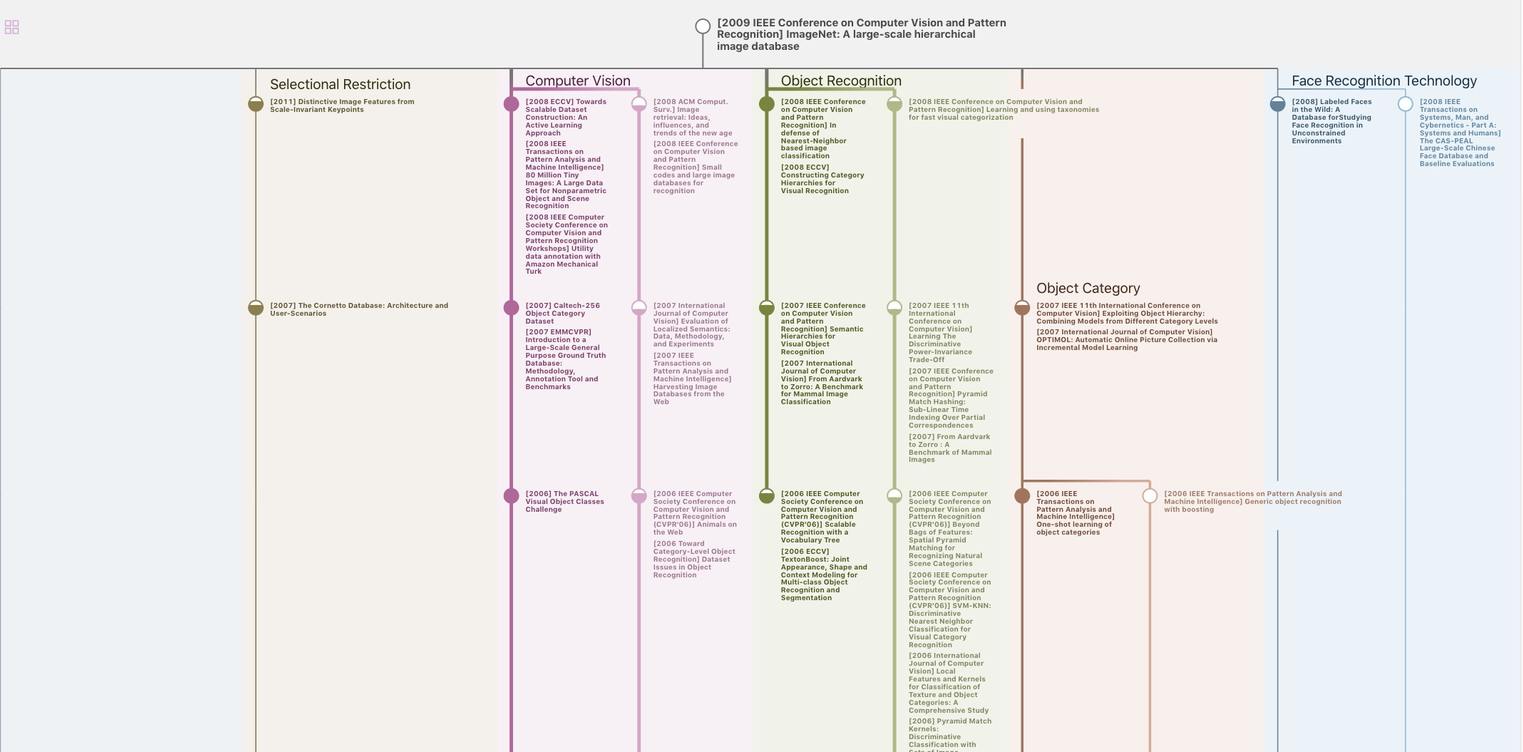
生成溯源树,研究论文发展脉络
Chat Paper
正在生成论文摘要