RLEKF: an optimizer for deep potential with ab initio accuracy
arxiv(2023)
摘要
It is imperative to accelerate the training of neural network force field such as Deep Potential, which usually requires thousands of images based on first-principles calculation and a couple of days to generate an accurate potential energy surface. To this end, we propose a novel optimizer named reorganized layer extended Kalman filtering (RLEKF), an optimized version of global extended Kalman filtering (GEKF) with a strategy of splitting big and gathering small layers to overcome the O ( N 2 ) computational cost of GEKF. This strategy provides an approximation of the dense weights error covariance matrix with a sparse diagonal block matrix for GEKF. We implement both RLEKF and the baseline Adam in our řDynamics package and numerical experiments are performed on 13 unbiased datasets. Overall, RLEKF converges faster with slightly better accuracy. For example, a test on a typical system, bulk copper, shows that RLEKF converges faster by both the number of training epochs (× 11.67) and wall-clock time (× 1.19). Besides, we theoretically prove that the updates of weights converge and thus are against the gradient exploding problem. Experimental results verify that RLEKF is not sensitive to the initialization of weights. The RLEKF sheds light on other AI-for-science applications where training a large neural network (with tons of thousands parameters) is a bottleneck.
更多查看译文
关键词
deep potential,ab initio accuracy,optimizer
AI 理解论文
溯源树
样例
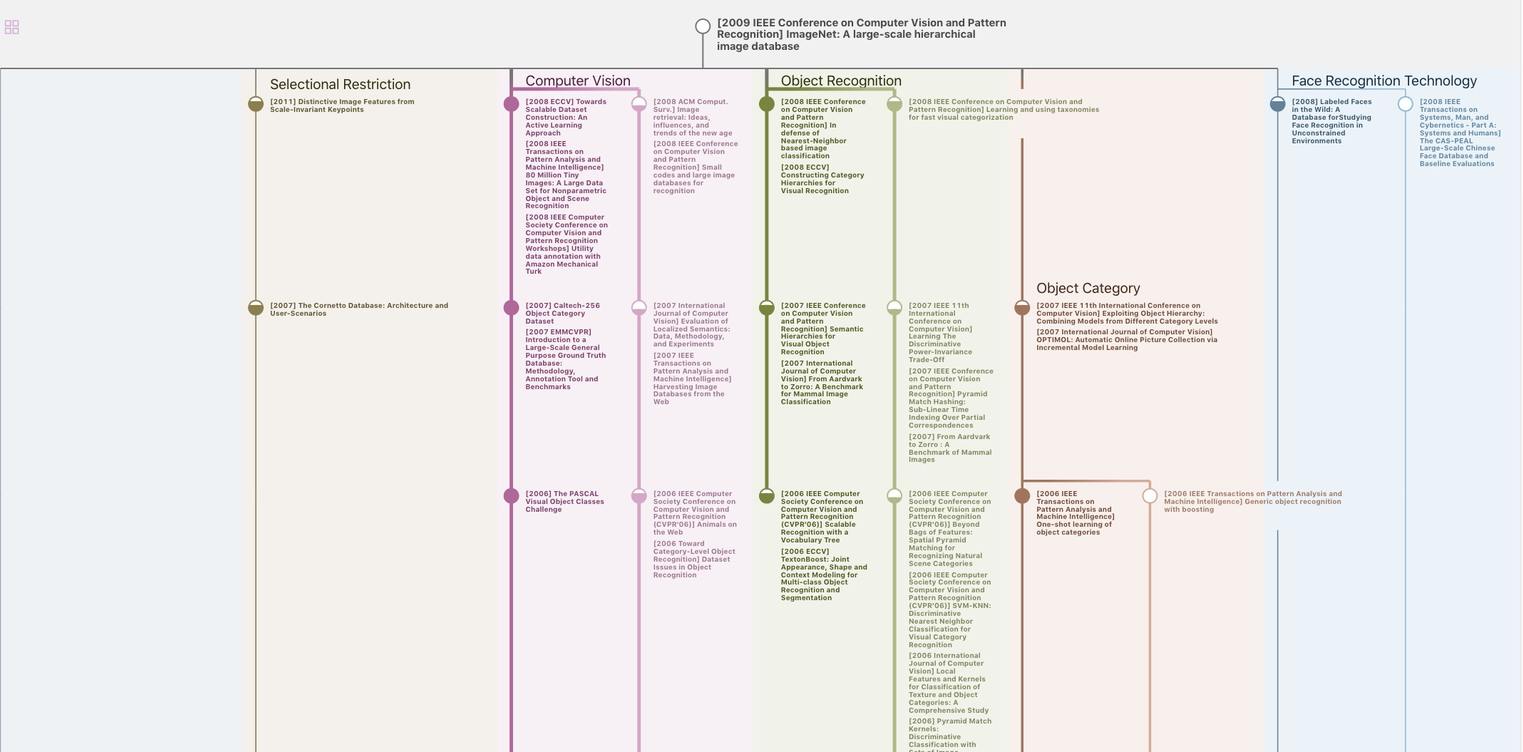
生成溯源树,研究论文发展脉络
Chat Paper
正在生成论文摘要