Statistical Safety and Robustness Guarantees for Feedback Motion Planning of Unknown Underactuated Stochastic Systems
arxiv(2023)
摘要
We present a method for providing statistical guarantees on runtime safety and goal reachability for integrated planning and control of a class of systems with unknown nonlinear stochastic underactuated dynamics. Specifically, given a dynamics dataset, our method jointly learns a mean dynamics model, a spatially-varying disturbance bound that captures the effect of noise and model mismatch, and a feedback controller based on contraction theory that stabilizes the learned dynamics. We propose a sampling-based planner that uses the mean dynamics model and simultaneously bounds the closed-loop tracking error via a learned disturbance bound. We employ techniques from Extreme Value Theory (EVT) to estimate, to a specified level of confidence, several constants which characterize the learned components and govern the size of the tracking error bound. This ensures plans are guaranteed to be safely tracked at runtime. We validate that our guarantees translate to empirical safety in simulation on a 10D quadrotor, and in the real world on a physical CrazyFlie quadrotor and Clearpath Jackal robot, whereas baselines that ignore the model error and stochasticity are unsafe.
更多查看译文
关键词
robustness guarantees,feedback motion
AI 理解论文
溯源树
样例
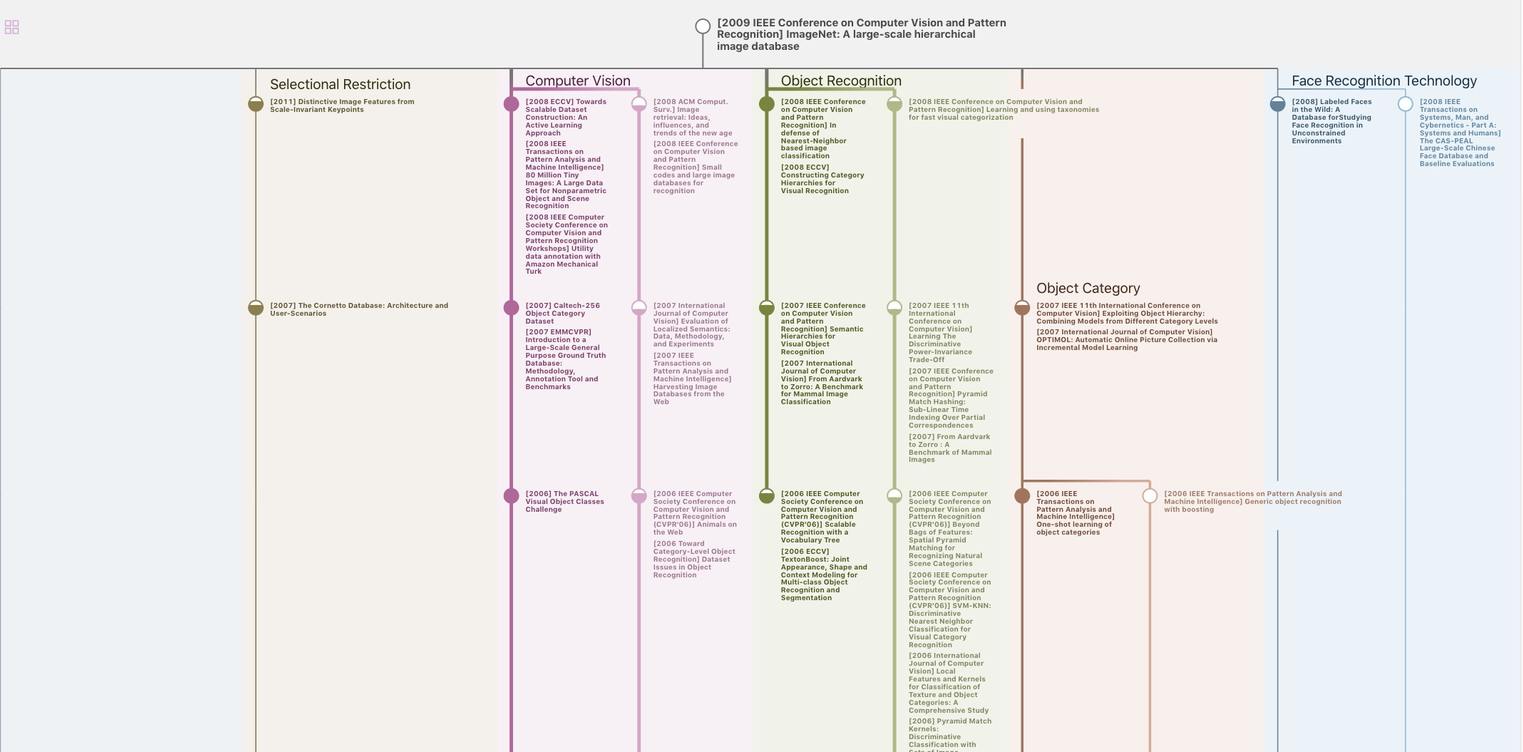
生成溯源树,研究论文发展脉络
Chat Paper
正在生成论文摘要