Machine learning of double-valued nonadiabatic coupling vectors around conical intersections.
The Journal of Chemical Physics(2023)
摘要
In recent years, machine learning has had an enormous success in fitting ab initio potential-energy surfaces to enable efficient simulations of molecules in their ground electronic state. In order to extend this approach to excited-state dynamics, one must not only learn the potentials but also nonadiabatic coupling vectors (NACs). There is a particular difficulty in learning NACs in systems that exhibit conical intersections, as due to the geometric-phase effect, the NACs may be double-valued and are, thus, not suitable as training data for standard machine-learning techniques. In this work, we introduce a set of auxiliary single-valued functions from which the NACs can be reconstructed, thus enabling a reliable machine-learning approach.
更多查看译文
关键词
nonadiabatic coupling vectors,machine learning,double-valued
AI 理解论文
溯源树
样例
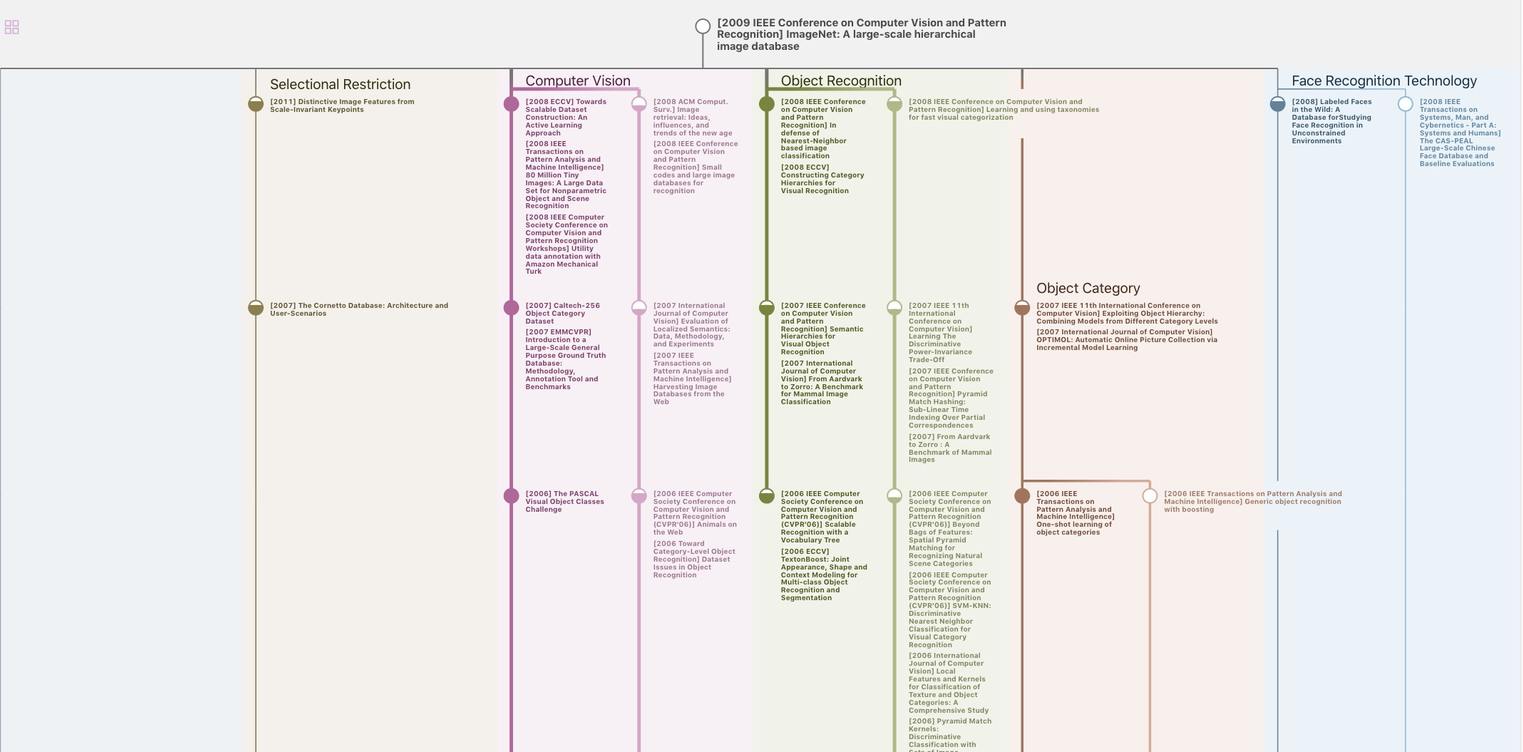
生成溯源树,研究论文发展脉络
Chat Paper
正在生成论文摘要