Leveraging class hierarchy for detecting missing annotations on hierarchical multi-label classification
Computers in Biology and Medicine(2022)
摘要
With the development of new sequencing technologies, availability of genomic data has grown exponentially. Over the past decade, numerous studies have used genomic data to identify associations between genes and biological functions. While these studies have shown success in annotating genes with functions, they often assume that genes are completely annotated and fail to take into account that datasets are sparse and noisy. This work proposes a method to detect missing annotations in the context of hierarchical multi-label classification. More precisely, our method exploits the relations of functions, represented as a hierarchy, by computing probabilities based on the paths of functions in the hierarchy. By performing several experiments on a variety of rice (Oriza sativa Japonica), we showcase that the proposed method accurately detects missing annotations and yields superior results when compared to state-of-art methods from the literature.
更多查看译文
关键词
Detecting missing annotations,Hierarchical multi-label classification,Structured output prediction,Gene function prediction,Gene ontology hierarchy,Random forest,Tree ensembles
AI 理解论文
溯源树
样例
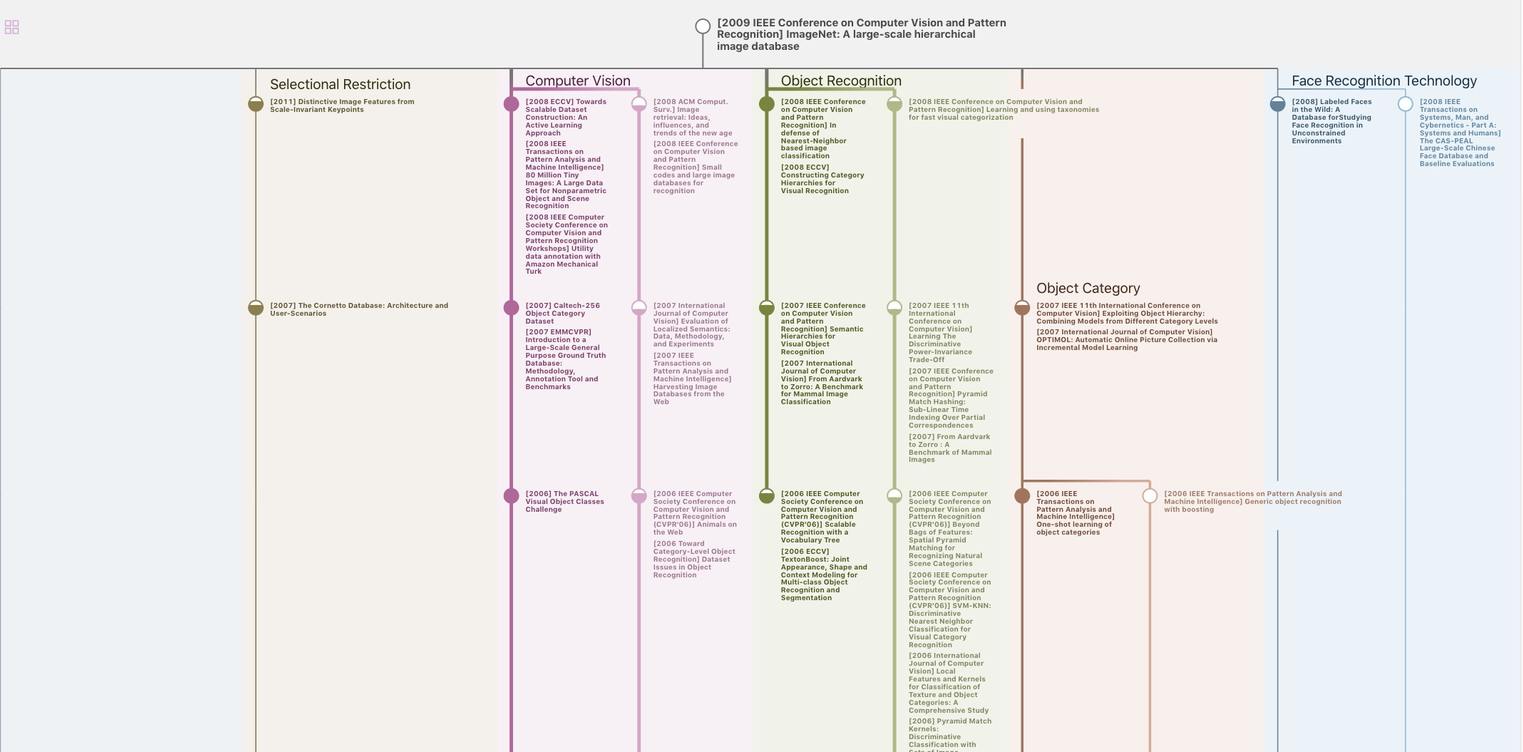
生成溯源树,研究论文发展脉络
Chat Paper
正在生成论文摘要