Towards more personalized digital health interventions: a clustering method of action and coping plans to promote physical activity
BMC public health(2022)
摘要
Background Despite effectiveness of action and coping planning in digital health interventions to promote physical activity (PA), attrition rates remain high. Indeed, support to make plans is often abstract and similar for each individual. Nevertheless, people are different, and context varies. Tailored support at the content level, involving suggestions of specific plans that are personalized to the individual, may reduce attrition and improve outcomes in digital health interventions. The aim of this study was to investigate whether user information relates toward specific action and coping plans using a clustering method. In doing so, we demonstrate how knowledge can be acquired in order to develop a knowledge-base, which might provide personalized suggestions in a later phase. Methods To establish proof-of-concept for this approach, data of 65 healthy adults, including 222 action plans and 204 coping plans, were used and were collected as part of the digital health intervention MyPlan 2.0 to promote PA. As a first step, clusters of action plans, clusters of coping plans and clusters of combinations of action plans and barriers of coping plans were identified using hierarchical clustering. As a second step, relations with user information (i.e. gender, motivational stage, ...) were examined using anova’s and chi 2 –tests. Results First, three clusters of action plans, eight clusters of coping plans and eight clusters of the combination of action and coping plans were identified. Second, relating these clusters to user information was possible for action plans: 1) Users with a higher BMI related more to outdoor leisure activities (F = 13.40, P < .001), 2) Women, users that didn’t perform PA regularly yet, or users with a job related more to household activities (X 2 = 16.92, P < .001; X 2 = 20.34, P < .001; X 2 = 10.79, P = .004; respectively), 3) Younger users related more to active transport and different sports activities (F = 14.40, P < .001). However, relating clusters to user information proved difficult for the coping plans and combination of action and coping plans. Conclusions The approach used in this study might be a feasible approach to acquire input for a knowledge-base, however more data (i.e. contextual and dynamic user information) from possible end users should be acquired in future research. This might result in a first type of context-aware personalized suggestions on the content level. Trial registration The digital health intervention MyPlan 2.0 was preregistered as a clinical trial (ID:NCT03274271). Release date: 6-September-2017.
更多查看译文
关键词
Behaviour change,Decision support systems,Digital health,E-health,Knowledge-base,M-health,Personalization,Physical activity
AI 理解论文
溯源树
样例
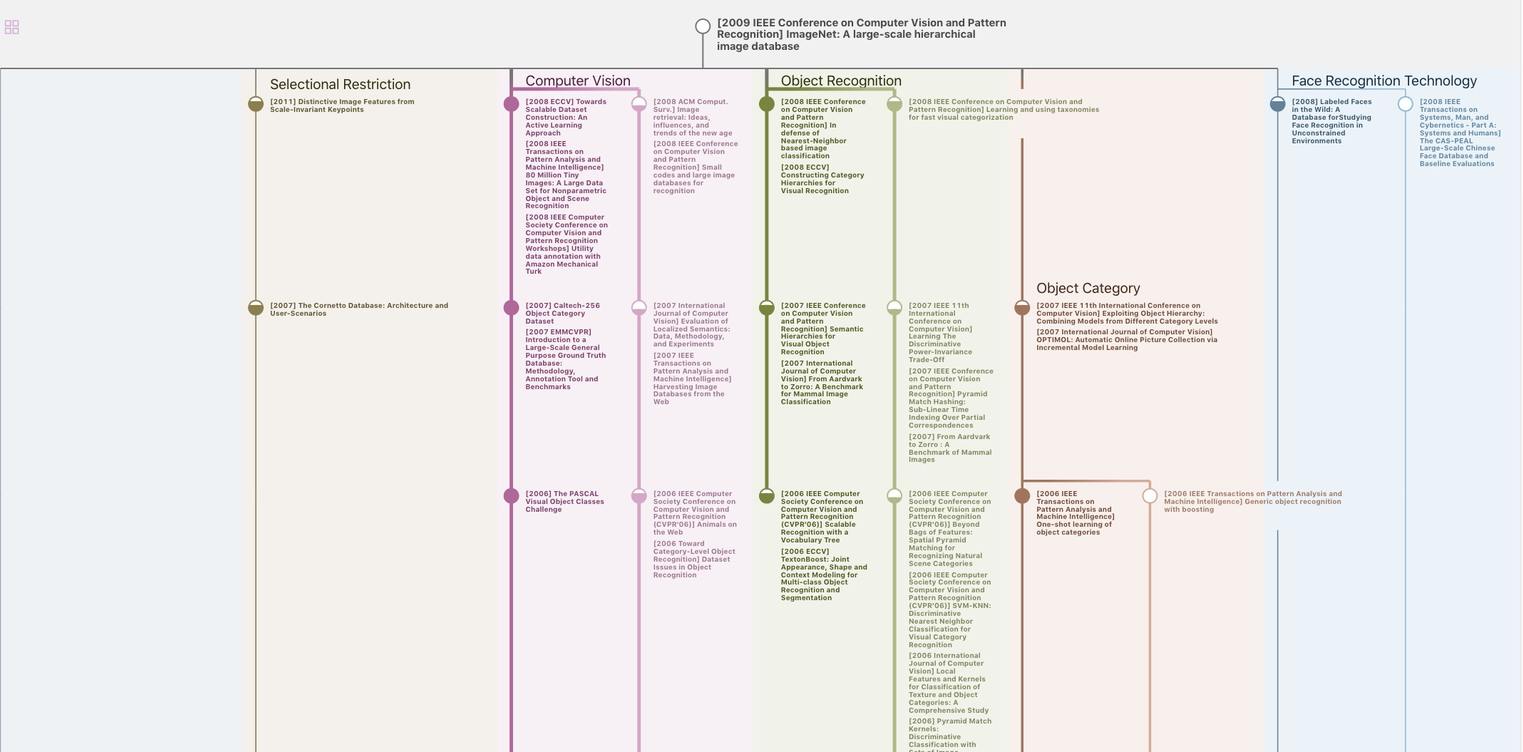
生成溯源树,研究论文发展脉络
Chat Paper
正在生成论文摘要