Predictive modelling of Parkinson’s disease progression based on RNA-Sequence with densely connected deep recurrent neural networks
Scientific reports(2022)
摘要
The advent of recent high throughput sequencing technologies resulted in unexplored big data of genomics and transcriptomics that might help to answer various research questions in Parkinson’s disease (PD) progression. While the literature has revealed various predictive models that use longitudinal clinical data for disease progression, there is no predictive model based on RNA-Sequence data of PD patients. This study investigates how to predict the PD Progression for a patient’s next medical visit by capturing longitudinal temporal patterns in the RNA-Seq data. Data provided by Parkinson Progression Marker Initiative (PPMI) includes 423 PD patients without revealing any race, sex, or age information with a variable number of visits and 34,682 predictor variables for 4 years. We propose a predictive model based on deep Recurrent Neural Network (RNN) with the addition of dense connections and batch normalization into RNN layers. The results show that the proposed architecture can predict PD progression from high dimensional RNA-seq data with a Root Mean Square Error (RMSE) of 6.0 and a rank-order correlation of (r = 0.83, p < 0.0001) between the predicted and actual disease status of PD.
更多查看译文
关键词
deep recurrent neural networks,parkinsons,disease progression,predictive modelling,rna-sequence
AI 理解论文
溯源树
样例
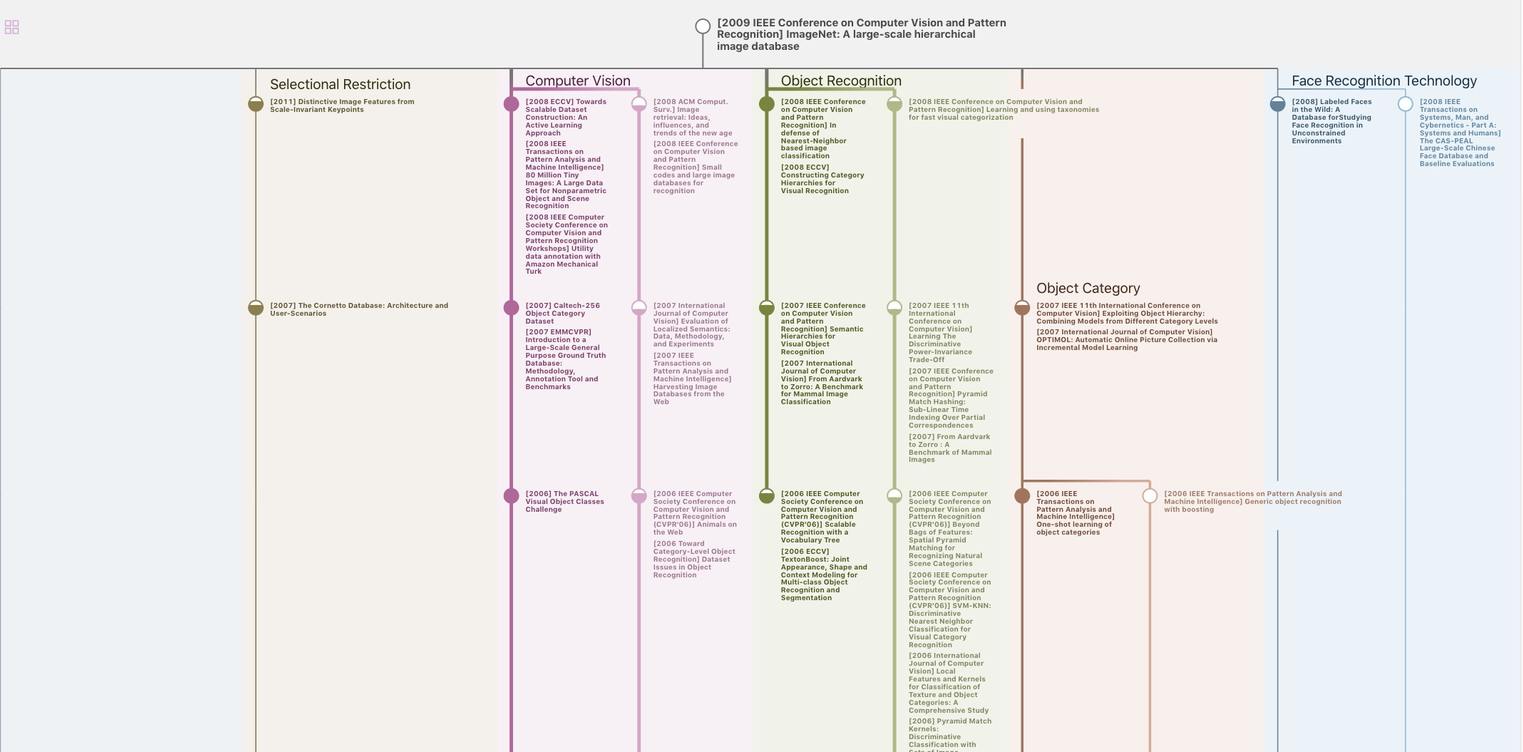
生成溯源树,研究论文发展脉络
Chat Paper
正在生成论文摘要