A Robust Algorithm for Candidate Pruning and Feature Weight Generation in E-Recruitment System
2022 24th International Multitopic Conference (INMIC)(2022)
摘要
Globalization, enhanced networking, and contemporary communication systems have resulted in a substantial increase in the number of resumes created. Processing this massive chunk manually is time-consuming. Multiple Criteria Decision Making (MCDM) techniques, such as “Analytic Hierarchy Process” (AHP), “The Technique for Order of Preference by Similarity to Ideal Solution” (TOPSIS), “VlseKriterijumska Optimizacija I Kompromisno Resenje” (VIKOR), and their state-of-the-art optimized variants, SlashRank, consider multiple factors and then rank the results accordingly to streamline the process. These algorithms primarily focus on reducing computational complexity, however, they ignore automated feature importance since they require manual features weights as an explicit input. In the end, this leads to a biased result and a decentralized method of hiring candidates. Our research addresses the human intervention observed in previously identified techniques and proposes a technique that automates candidate pruning, manual feature priority input, and generates new feature importance based on user feedback. Our approach can be decomposed into automated candidate pruning for the highest priority feature, candidate selection feedback, and trend-based feature weight generation to replicate actual recruitment feature priority fluctuations. Our algorithm demonstrates promising results in minimizing human biases and generating a dynamic trend of feature importance over time.
更多查看译文
关键词
feature weight,MCDM,AHP,feedback,automation,temporal analysis
AI 理解论文
溯源树
样例
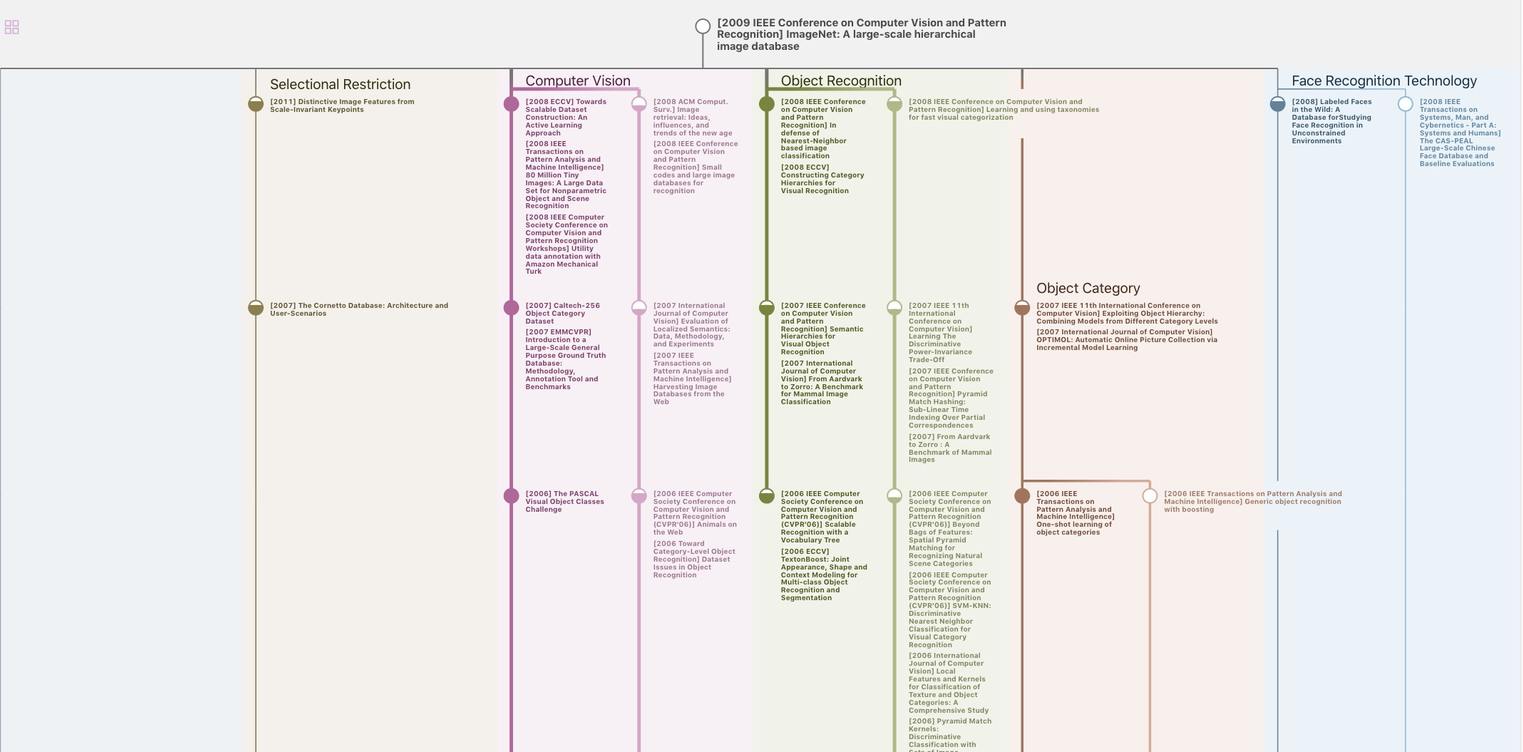
生成溯源树,研究论文发展脉络
Chat Paper
正在生成论文摘要