Self-Paced and Discrete Multiple Kernel k -Means
user-61447a76e55422cecdaf7d19(2022)
摘要
Multiple Kernel k-means (MKKM) uses various kernels from different sources to improve clustering performance. However, most of the existing models are non-convex, which is prone to be stuck into bad local optimum, especially with noise and outliers. To address the issue, we propose a novel Self-Paced and Discrete Multiple Kernel k-Means (SPD-MKKM). It learns the MKKM model in a meaningful order by progressing both samples and kernels from easy to complex, which is beneficial to avoid bad local optimum. In addition, whereas existing methods optimize in two stages: learning the relaxation matrix and then finding the discrete one by extra discretization, our work can directly gain the discrete cluster indicator matrix without extra process. What's more, a well-designed alternative optimization is employed to reduce the overall computational complexity via using the coordinate descent technique. Finally, thorough experiments performed on real-world datasets illustrated the excellence and efficacy of our method.
更多查看译文
关键词
Clustering, Multiple kernel k-means, Self-Paced Learning
AI 理解论文
溯源树
样例
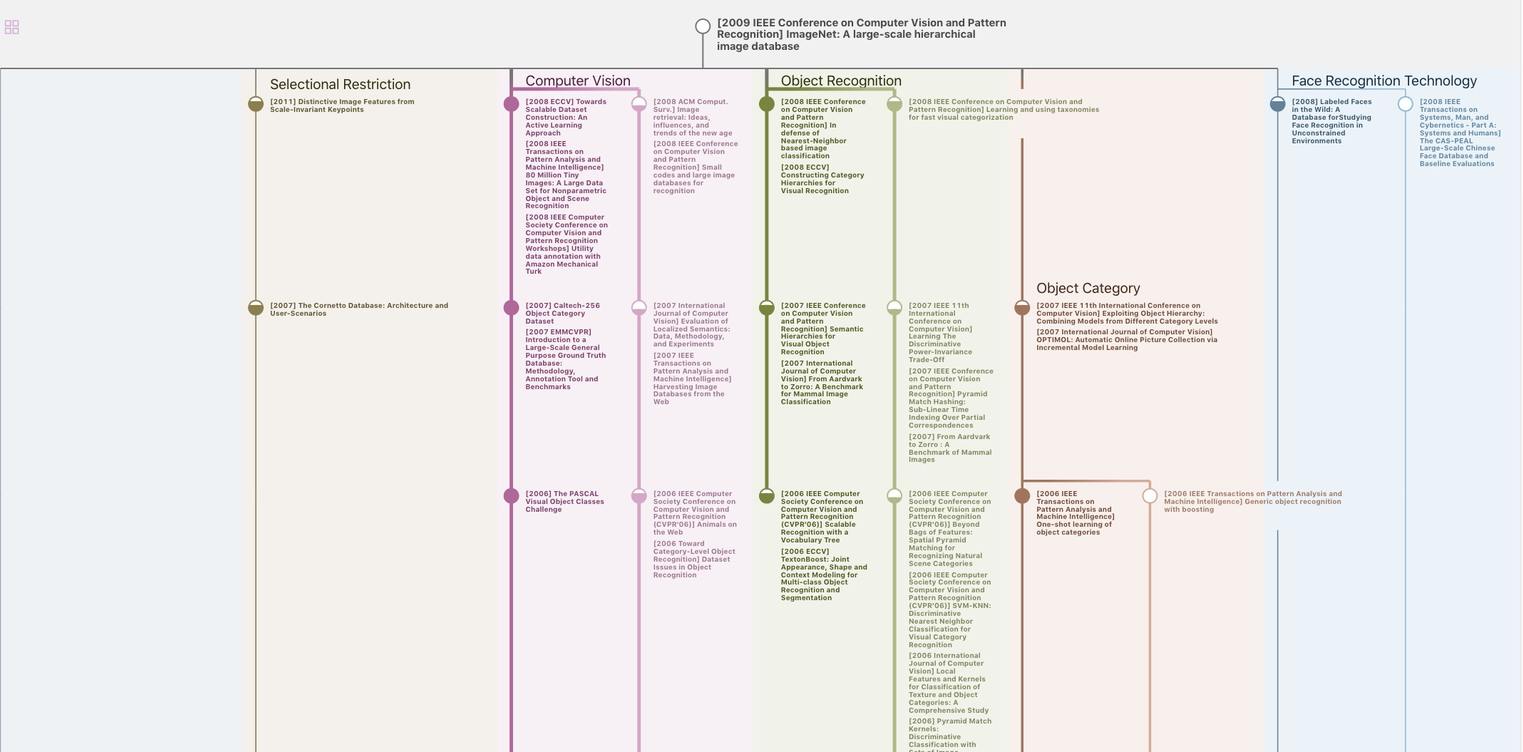
生成溯源树,研究论文发展脉络
Chat Paper
正在生成论文摘要