Multimodal Imaging-Based Classification of PTSD Using Data-Driven Computational Approaches: A Multisite Big Data Study from the ENIGMA-PGC PTSD Consortium
biorxiv(2022)
摘要
Background: Current clinical assessments of Posttraumatic stress disorder (PTSD) rely solely on subjective symptoms and experiences reported by the patient, rather than objective biomarkers of the illness. Recent advances in data-driven computational approaches have been helpful in devising tools to objectively diagnose psychiatric disorders. Here we aimed to classify individuals with PTSD versus controls using heterogeneous brain datasets from the ENIGMA-PGC PTSD Working group. Methods: We analyzed brain MRI data from 3,527 structural-MRI; 2,502 resting state-fMRI; and 1,953 diffusion-MRI. First, we identified the brain features that best distinguish individuals with PTSD from controls (TEHC and HC) using traditional machine learning methods. Second, we assessed the utility of the denoising variational autoencoder (DVAE) and evaluated its classification performance. Third, we assessed the generalizability and reproducibility of both models using leave-one-site-out cross-validation procedure for each modality. Results: We found lower performance in classifying PTSD vs. controls with data from over 20 sites (60% test AUC for s-MRI, 59% for rs-fMRI and 56% for d-MRI), as compared to other studies run on single-site data. The performance increased when classifying PTSD from HC without trauma history across all three modalities (75% AUC). The classification performance remained intact when applying the DVAE framework, which reduced the number of features. Finally, we found that the DVAE framework achieved better generalization to unseen datasets compared with the traditional machine learning frameworks, albeit performance was slightly above chance. Conclusion: Our findings highlight the promise offered by machine learning methods for the diagnosis of patients with PTSD. The utility of brain biomarkers across three MRI modalities and the contribution of DVAE models for improving generalizability offers new insights into neural mechanisms involved in PTSD.
### Competing Interest Statement
Dr Thompson received partial grant support from Biogen, Inc., and Amazon, Inc., for work unrelated to the current study; Dr. Lebois reports unpaid membership on the Scientific Committee for International Society for the Study of Trauma and Dissociation (ISSTD), grant support from the National Institute of Mental Health, K01 MH118467 and the Julia Kasparian Fund for Neuroscience Research, McLean Hospital. Dr. Lebois also reports spousal IP payments from Vanderbilt University for technology licensed to Acadia Pharmaceuticals unrelated to the present work. ISSTD and NIMH were not involved in the analysis or preparation of the manuscript; Dr. Etkin reports salary and equity from Alto Neuroscience, equity from Mindstrong Health and Akili Interactive. Other authors have no conflicts of interest to declare.
更多查看译文
关键词
multimodal,multisite big data-driven study,classification,imaging-based,enigma-pgc
AI 理解论文
溯源树
样例
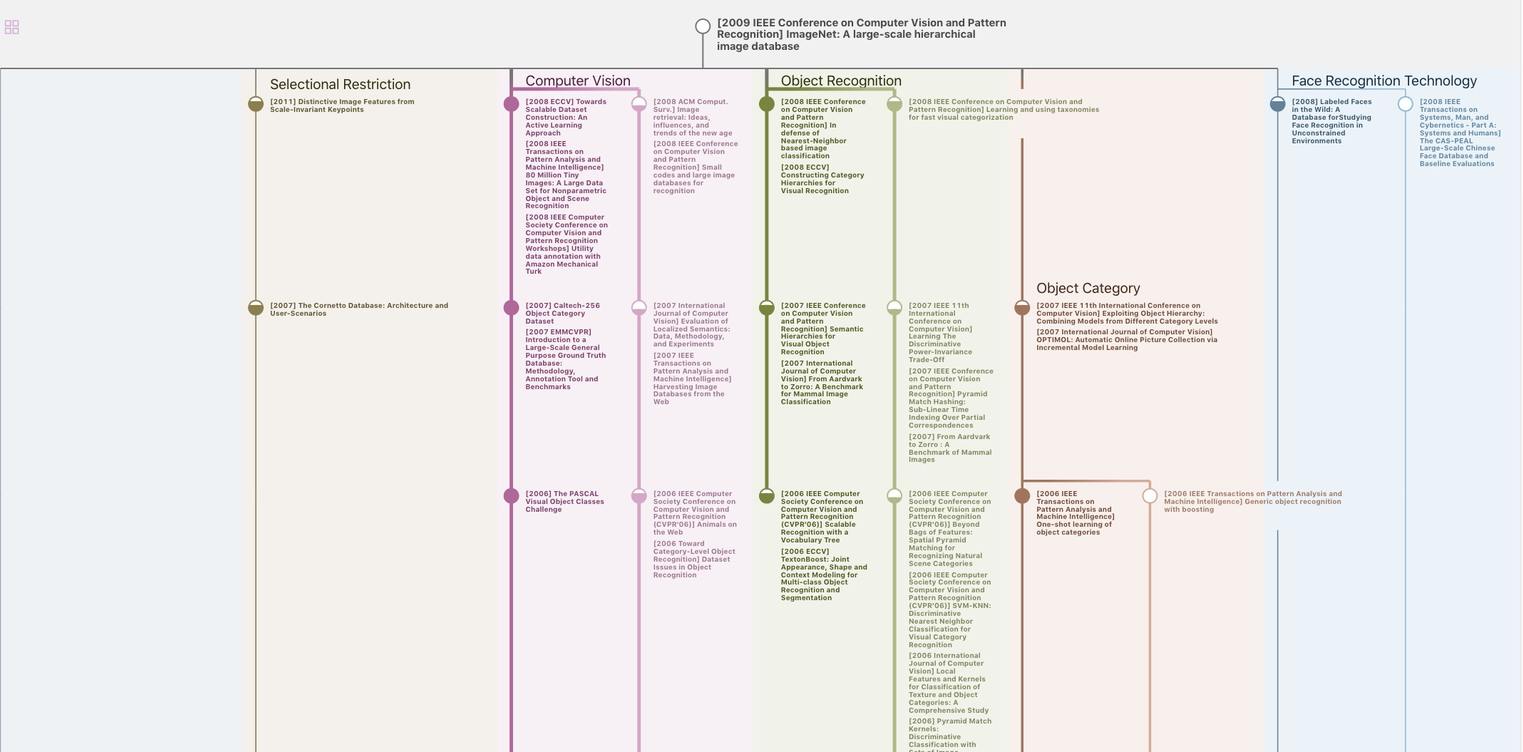
生成溯源树,研究论文发展脉络
Chat Paper
正在生成论文摘要