Causal Inference on Win Ratio for Observational Data with Dependent Subjects
arxiv(2022)
摘要
Composite endpoints are commonly used with an anticipation that clinically relevant endpoints as a whole would yield meaningful treatment benefits. The win ratio is a rank-based statistic to summarize composite endpoints, allowing prioritizing the important components of the composite endpoints. Recent development in statistical inference for the win ratio statistic has been focusing on independent subjects without any potential confounding. When analyzing composite endpoints using observational data, one of the important challenges is confounding at baseline. Additionally, hierarchical observational data structures are commonly seen in practice, especially in multi-center studies with patients nesting within hospitals. Such hierarchical structure can introduce potential dependency or cluster effects among observations in the analysis. To address these two issues when using the win ratio statistic, we propose a weighted stratified causal win ratio estimator with calibrated weights. The calibrated weights create balanced patient-level covariates and cluster effect distributions between comparison groups. We conducted extensive simulation studies and showed promising performance of the proposed estimator in terms of bias, variance estimation, type I error and power analysis, regardless of the allocation of treatment assignments at baseline and intra-cluster correlations within clusters. Lastly, the proposed estimator was applied to an observational study among children with traumatic brain injury.
更多查看译文
关键词
win ratio,observational data,dependent
AI 理解论文
溯源树
样例
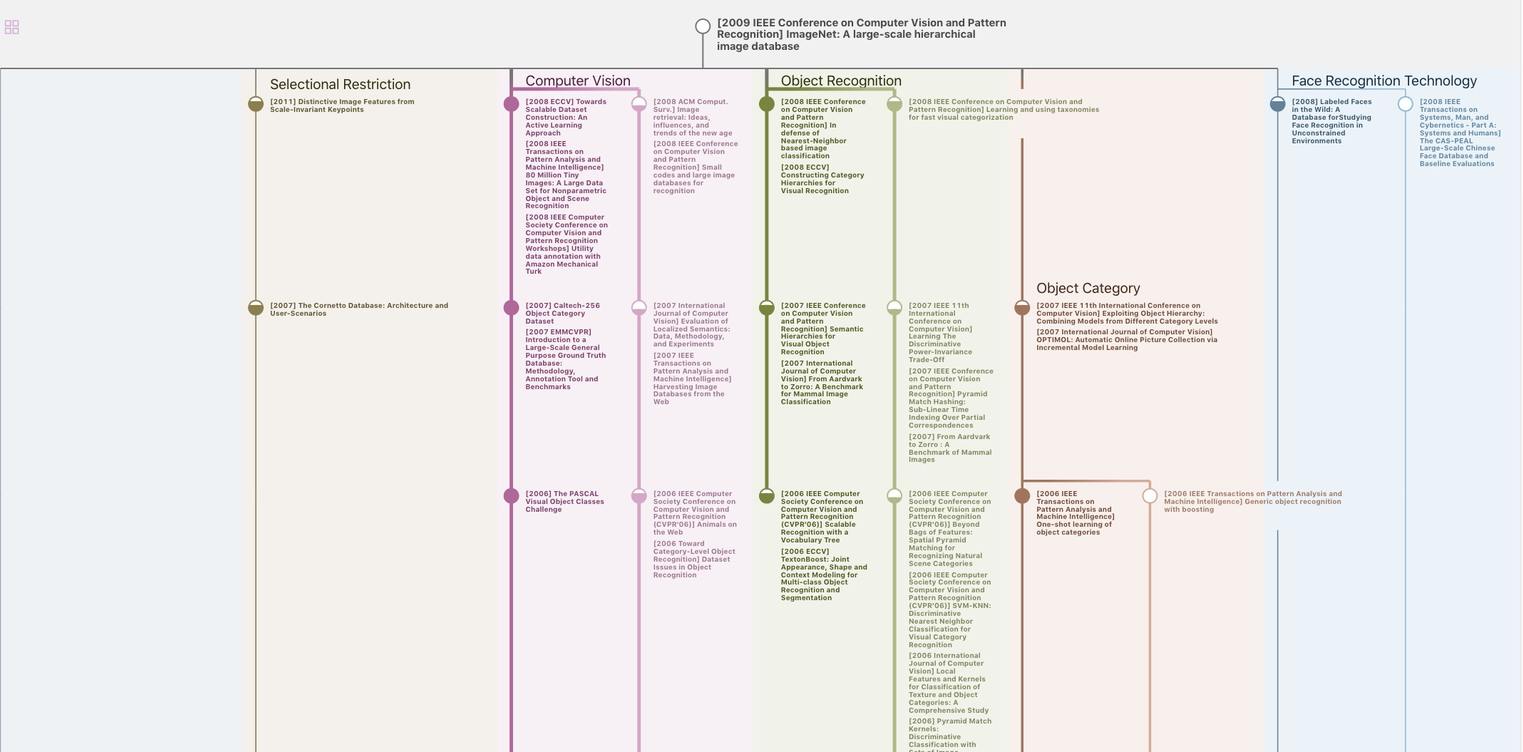
生成溯源树,研究论文发展脉络
Chat Paper
正在生成论文摘要