CamoFormer: Masked Separable Attention for Camouflaged Object Detection
arxiv(2022)
摘要
How to identify and segment camouflaged objects from the background is challenging. Inspired by the multi-head self-attention in Transformers, we present a simple masked separable attention (MSA) for camouflaged object detection. We first separate the multi-head self-attention into three parts, which are responsible for distinguishing the camouflaged objects from the background using different mask strategies. Furthermore, we propose to capture high-resolution semantic representations progressively based on a simple top-down decoder with the proposed MSA to attain precise segmentation results. These structures plus a backbone encoder form a new model, dubbed CamoFormer. Extensive experiments show that CamoFormer surpasses all existing state-of-the-art methods on three widely-used camouflaged object detection benchmarks. There are on average around 5% relative improvements over previous methods in terms of S-measure and weighted F-measure.
更多查看译文
关键词
camouflaged object detection,separable attention,object detection,masked
AI 理解论文
溯源树
样例
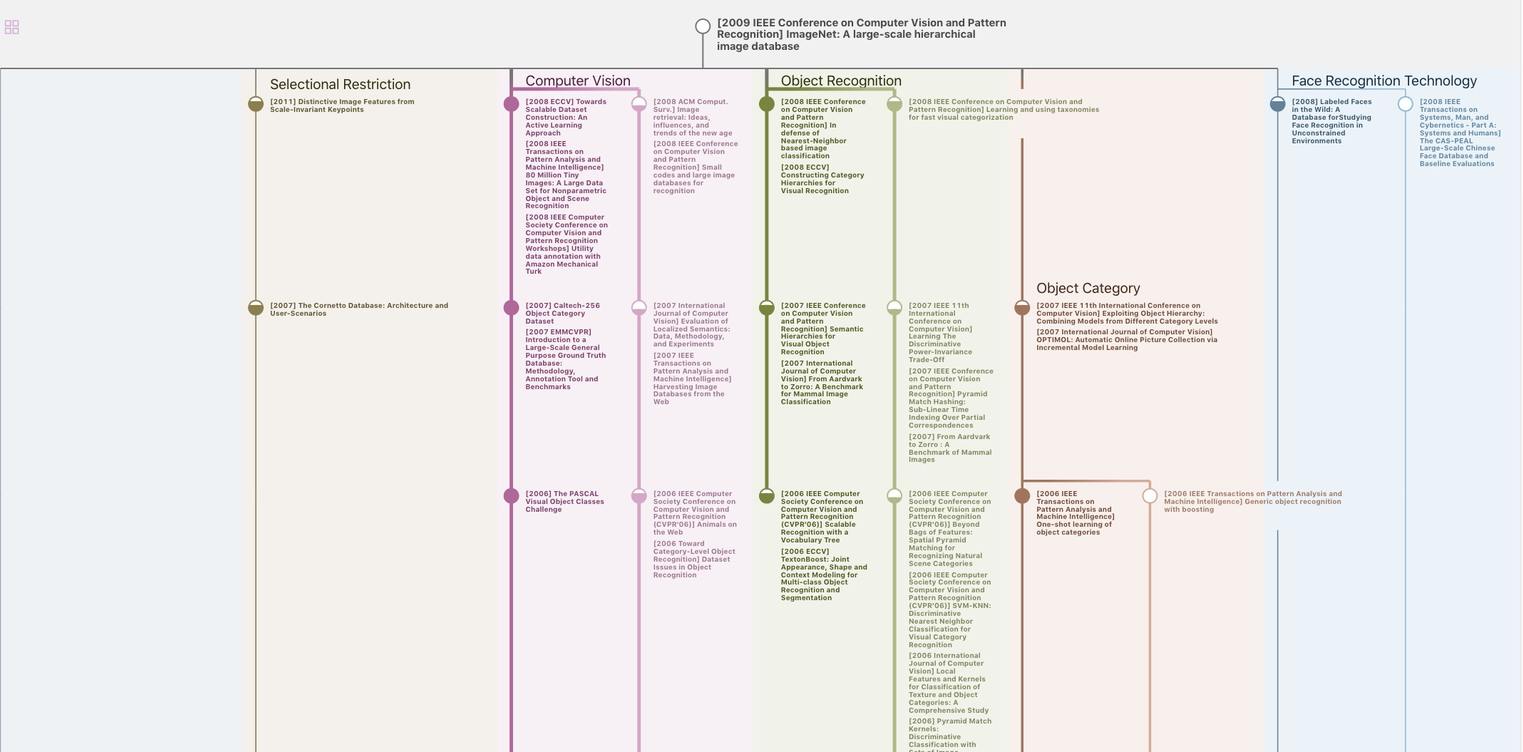
生成溯源树,研究论文发展脉络
Chat Paper
正在生成论文摘要