Dual attentive fusion for EEG-based brain-computer interfaces.
Frontiers in neuroscience(2022)
摘要
The classification based on Electroencephalogram (EEG) is a challenging task in the brain-computer interface (BCI) field due to data with a low signal-to-noise ratio. Most current deep learning based studies in this challenge focus on designing a desired convolutional neural network (CNN) to learn and classify the raw EEG signals. However, only CNN itself may not capture the highly discriminative patterns of EEG due to a lack of exploration of attentive spatial and temporal dynamics. To improve information utilization, this study proposes a Dual Attentive Fusion Model (DAFM) for the EEG-based BCI. DAFM is employed to capture the spatial and temporal information by modeling the interdependencies between the features from the EEG signals. To our best knowledge, our method is the first to fuse spatial and temporal dimensions in an interactive attention module. This module improves the expression ability of the extracted features. Extensive experiments implemented on four publicly available datasets demonstrate that our method outperforms state-of-the-art methods. Meanwhile, this work also indicates the effectiveness of Dual Attentive Fusion Module.
更多查看译文
关键词
P300,brain-computer interface,dual attentive fusion,electroencephalography,motor imagery
AI 理解论文
溯源树
样例
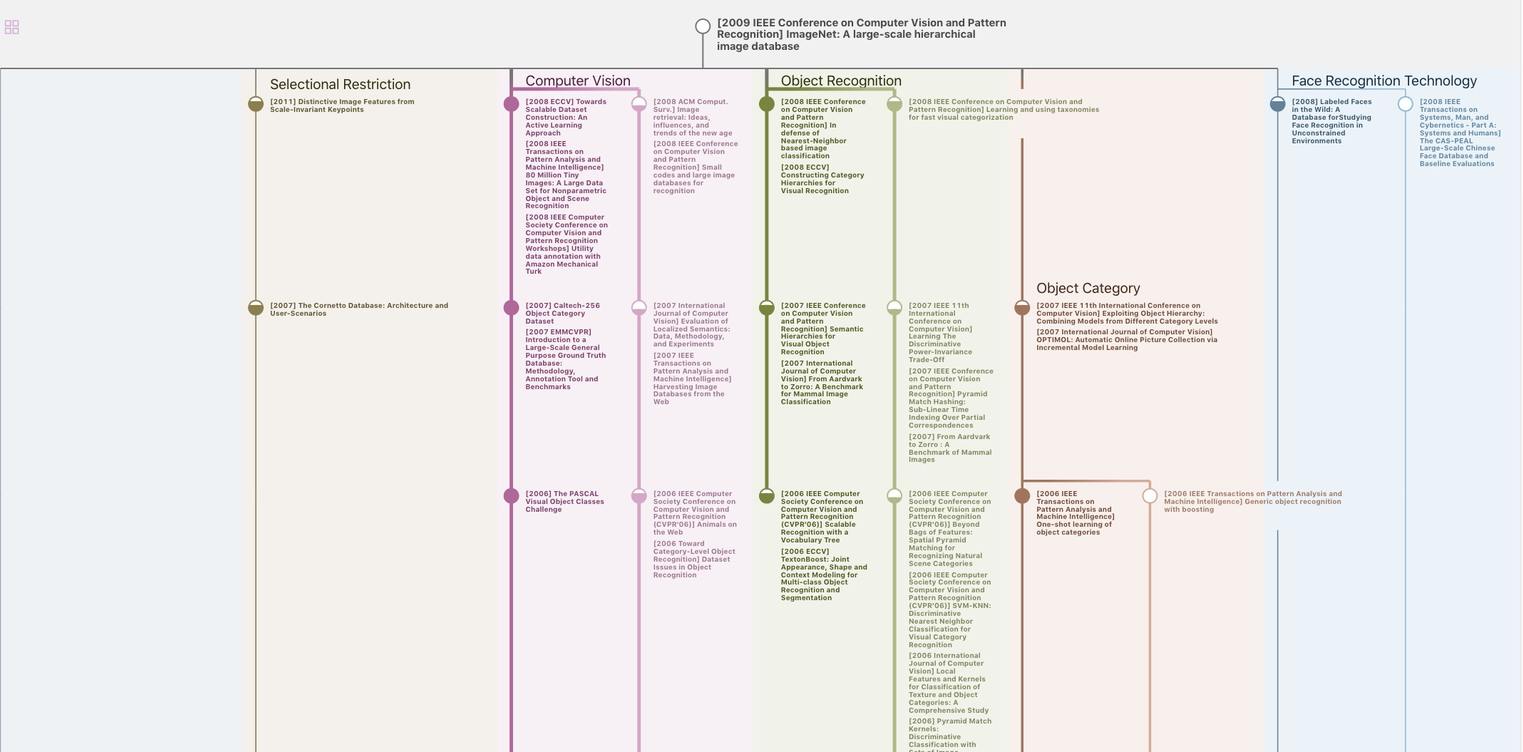
生成溯源树,研究论文发展脉络
Chat Paper
正在生成论文摘要