Bearing fault diagnosis based on particle swarm optimization fusion convolutional neural network.
Frontiers in neurorobotics(2022)
摘要
Bearings are the most basic and important mechanical parts. The stable and safe operation of the equipment requires bearing fault diagnosis in advance. So, bearing fault diagnosis is an important technology. However, the feature extraction quality of the traditional convolutional neural network bearing fault diagnosis is not high and the recognition accuracy will decline under different working conditions. In response to these questions, a bearing fault model based on particle swarm optimization (PSO) fusion convolution neural network is proposed in this paper. The model first adaptively adjusts the hyperparameters of the model through PSO, then introduces residual connections to prevent the gradient from disappearing, uses global average pooling to replace the fully connected layer to reduce the training parameters of the model, and finally adds a dropout layer to prevent network overfitting. The experimental results show that the model is under four conditions, two of which can achieve 100% recognition, and the other two can also achieve more than 98% accuracy. And compared with the traditional diagnosis method, the model has higher accuracy under variable working conditions. This research has important research significance and economic value in the field of the intelligent machinery industry.
更多查看译文
关键词
CNN,PSO,adaptive adjustment,bearing,fault diagnosis
AI 理解论文
溯源树
样例
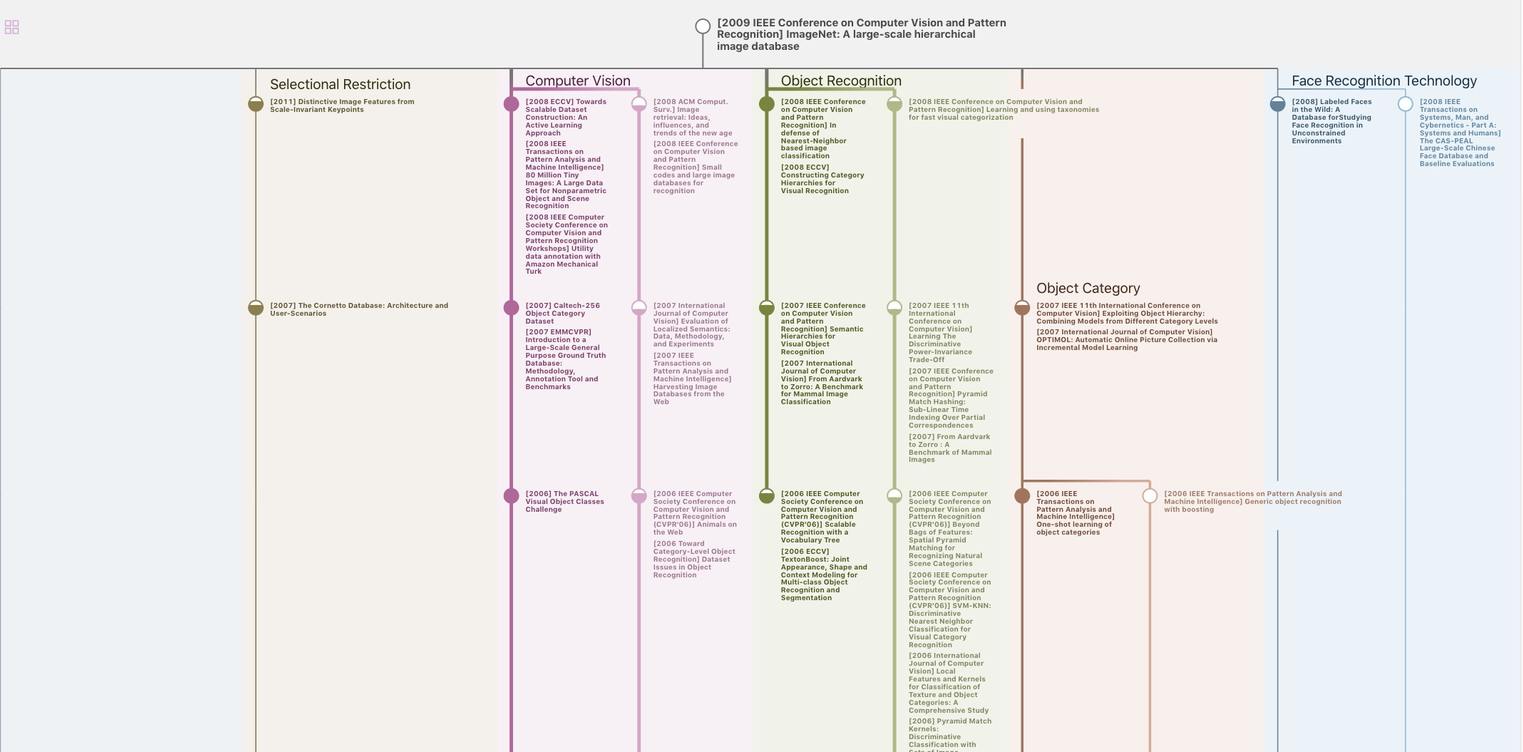
生成溯源树,研究论文发展脉络
Chat Paper
正在生成论文摘要