Pink urine syndrome in intensive care unit
Anaesthesia Critical Care & Pain Medicine(2023)
摘要
While clinical Artificial Intelligence (cAI) mortality prediction models and relevant studies have increased, limitations including the lack of external validation studies and inadequate model calibration leading to decreased overall accuracy have been observed. To combat this, we developed and evaluated a novel deep neural network (DNN) and a validation framework to promote transparent cAI development.Data from Japan’s largest ICU database was used to develop the DNN model, predicting in-hospital mortality including ICU and post-ICU mortality by days since ICU discharge. The most important variables to the model were extracted with SHapley Additive exPlanations (SHAP) to examine the DNN’s efficacy as well as develop models that were also externally validated.The area under the receiver operating characteristic curve (AUC) for predicting ICU mortality was 0.94 [0.93-0.95], and 0.91 [0.90-0.92] for in-hospital mortality, ranging between 0.91–0.95 throughout one year since ICU discharge. An external validation using only the top 20 variables resulted with higher AUCs than traditional severity scores.Our DNN model consistently generated AUCs between 0.91–0.95 regardless of days since ICU discharge. The 20 most important variables to our DNN, also generated higher AUCs than traditional severity scores regardless of days since ICU discharge. To our knowledge, this is the first study that predicts ICU and in-hospital mortality using cAI by post-ICU discharge days up to over a year. This finding could contribute to increased transparency on cAI applications.
更多查看译文
关键词
Pink urine syndrome,Propofol,Sedation
AI 理解论文
溯源树
样例
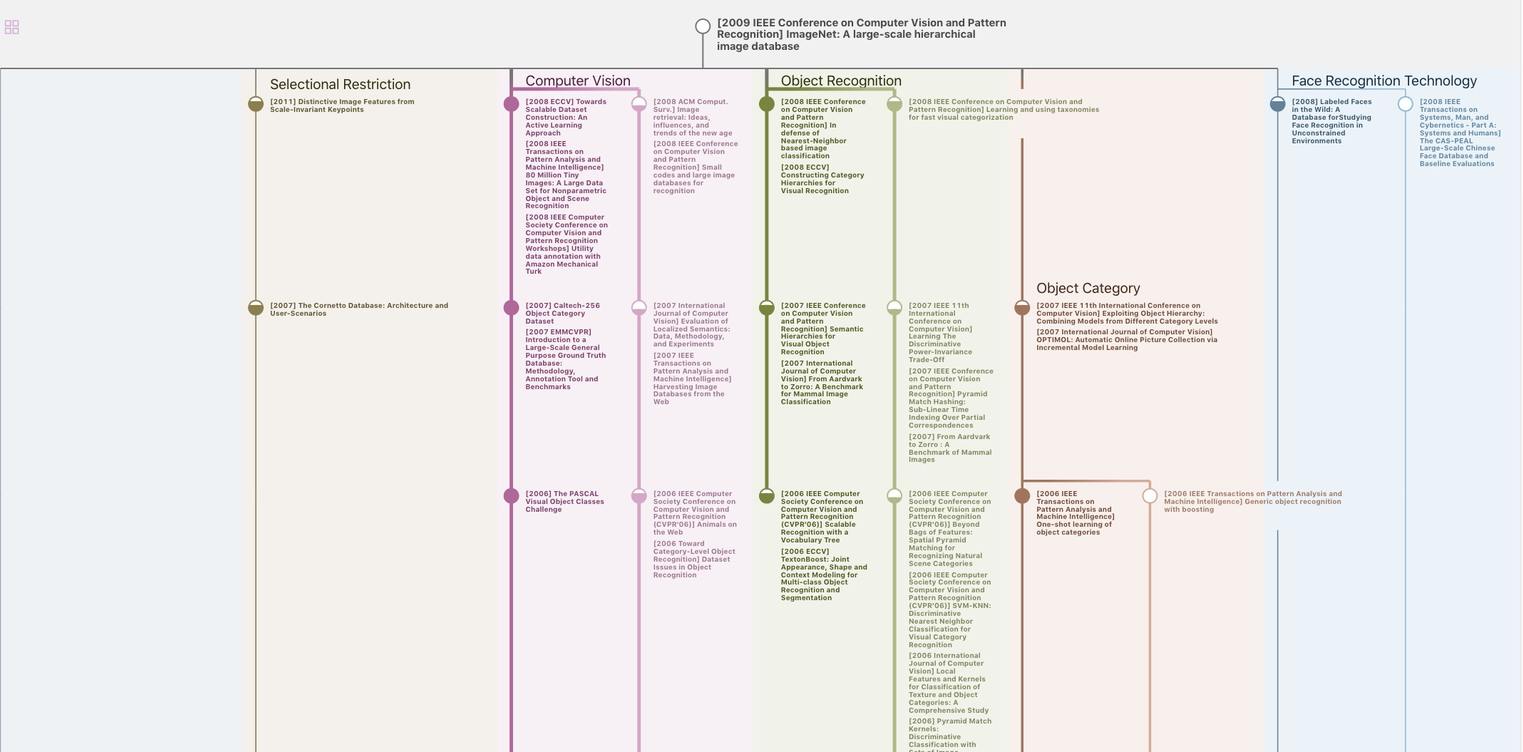
生成溯源树,研究论文发展脉络
Chat Paper
正在生成论文摘要