Regression with Label Differential Privacy
ICLR 2023(2022)
摘要
We study the task of training regression models with the guarantee of label differential privacy (DP). Based on a global prior distribution on label values, which could be obtained privately, we derive a label DP randomization mechanism that is optimal under a given regression loss function. We prove that the optimal mechanism takes the form of a ``randomized response on bins'', and propose an efficient algorithm for finding the optimal bin values. We carry out a thorough experimental evaluation on several datasets demonstrating the efficacy of our algorithm.
更多查看译文
关键词
label differential privacy,regression
AI 理解论文
溯源树
样例
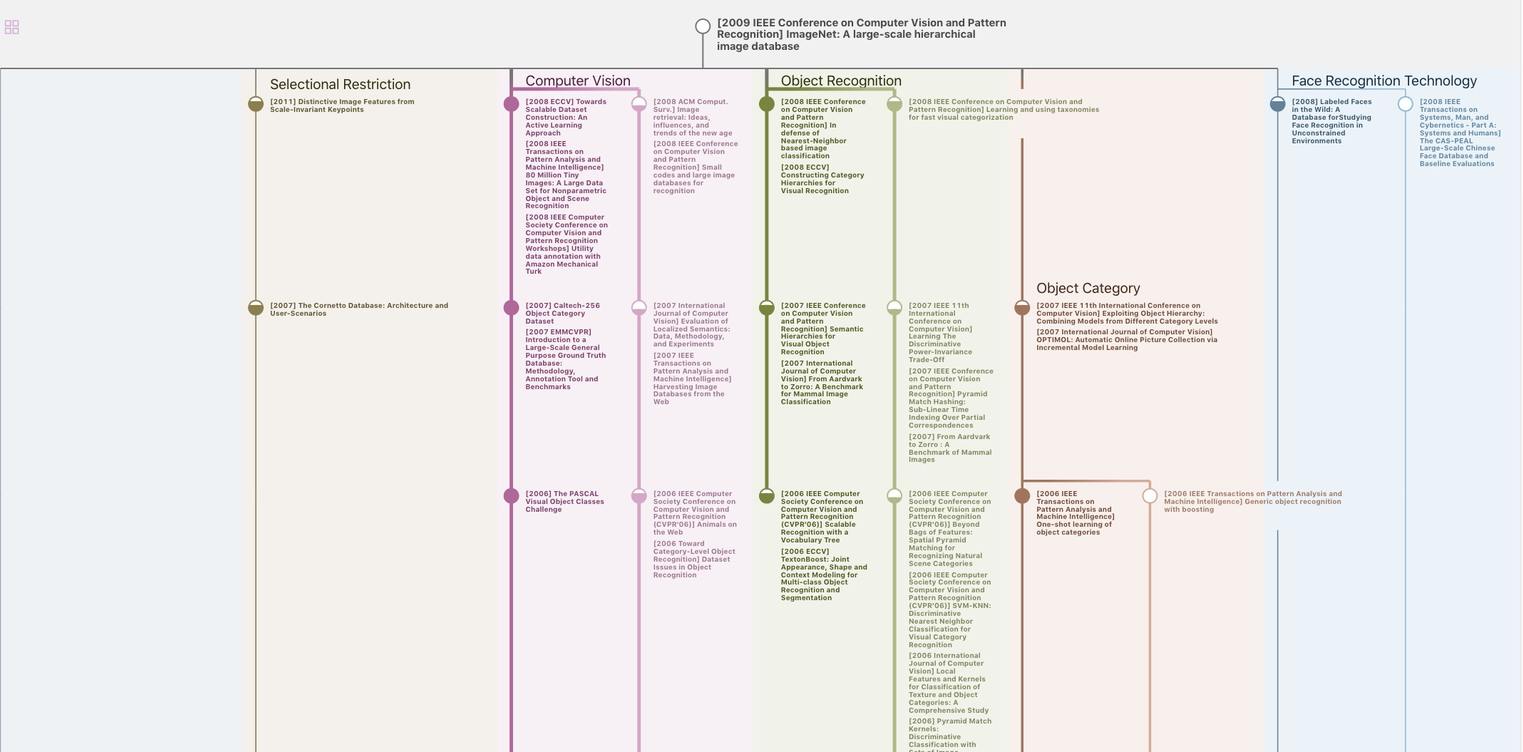
生成溯源树,研究论文发展脉络
Chat Paper
正在生成论文摘要