A Study on the Wind Power Forecasting Model Using Transfer Learning Approach
Electronics(2022)
摘要
Recently, wind power plants that generate wind energy with electricity are attracting a lot of attention thanks to their smaller installation area and cheaper power generation costs. In wind power generation, it is important to predict the amount of generated electricity because the power system would be unstable due to uncertainty in supply. However, it is difficult to accurately predict the amount of wind power generation because the power varies due to several causes, such as wind speed, wind direction, temperature, etc. In this study, we deal with a mid-term (one day ahead) wind power forecasting problem with a data-driven approach. In particular, it is intended to solve the problem of a newly completed wind power generator that makes it very difficult to predict the amount of electricity generated due to the lack of data on past power generation. To this end, a deep learning based transfer learning model was proposed and compared with other models, such as a deep learning model without transfer learning and Light Gradient Boosting Machine (LGBM). As per the experimental results, when the proposed transfer learning model was applied to a similar wind power complex in the same region, it was confirmed that the low predictive performance of the newly constructed generator could be supplemented.
更多查看译文
关键词
wind power forecasting,transfer learning,data-driven approach,mid-term forecasting
AI 理解论文
溯源树
样例
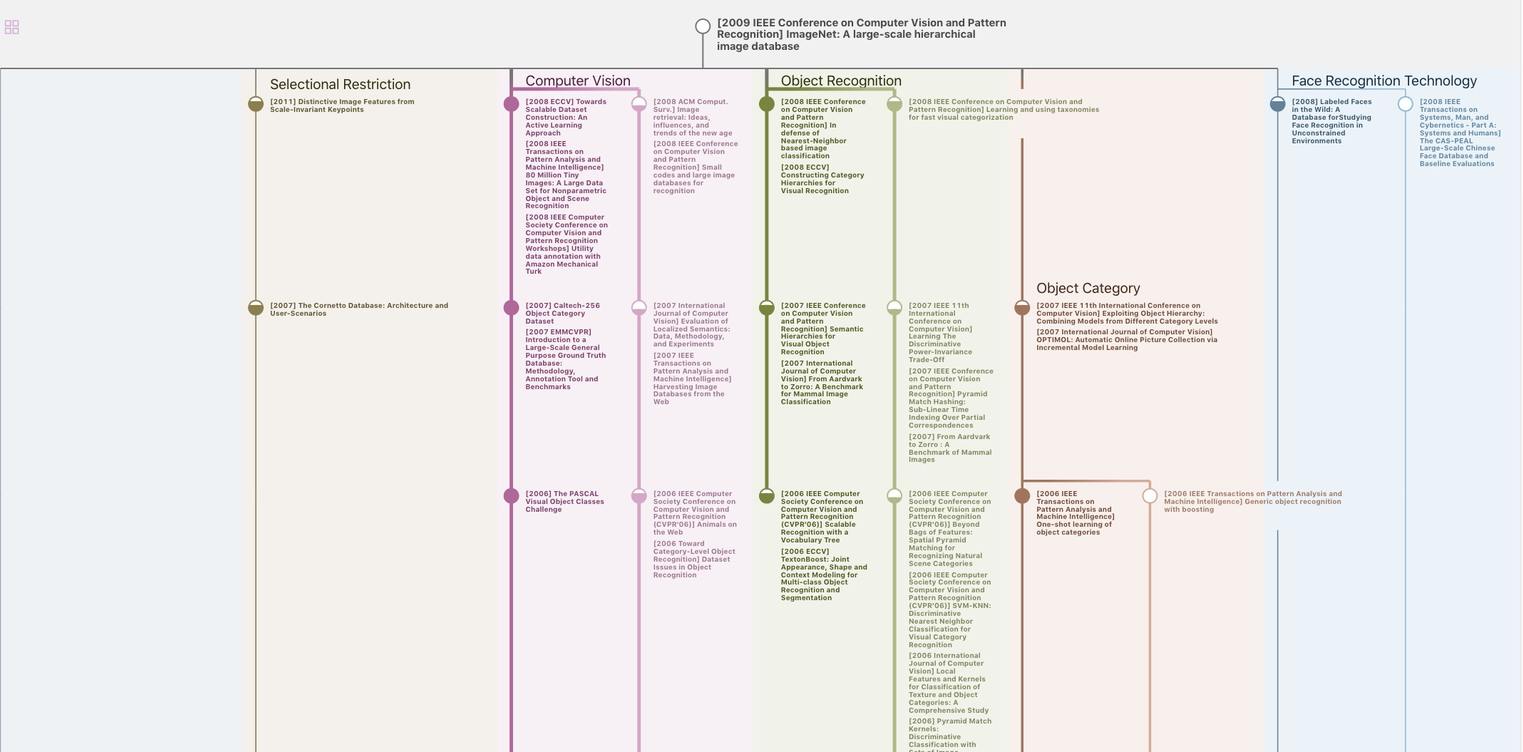
生成溯源树,研究论文发展脉络
Chat Paper
正在生成论文摘要