Masked Lip-Sync Prediction by Audio-Visual Contextual Exploitation in Transformers.
SIGGRAPH Asia(2022)
摘要
Previous studies have explored generating accurately lip-synced talking faces for arbitrary targets given audio conditions. However, most of them deform or generate the whole facial area, leading to non-realistic results. In this work, we delve into the formulation of altering only the mouth shapes of the target person. This requires masking a large percentage of the original image and seamlessly inpainting it with the aid of audio and reference frames. To this end, we propose the Audio-Visual Context-Aware Transformer (AV-CAT) framework, which produces accurate lip-sync with photo-realistic quality by predicting the masked mouth shapes. Our key insight is to exploit desired contextual information provided in audio and visual modalities thoroughly with delicately designed Transformers. Specifically, we propose a convolution-Transformer hybrid backbone and design an attention-based fusion strategy for filling the masked parts. It uniformly attends to the textural information on the unmasked regions and the reference frame. Then the semantic audio information is involved in enhancing the self-attention computation. Additionally, a refinement network with audio injection improves both image and lip-sync quality. Extensive experiments validate that our model can generate high-fidelity lip-synced results for arbitrary subjects.
更多查看译文
关键词
Lip-Sync, Transformer, Audio-Visual Learning
AI 理解论文
溯源树
样例
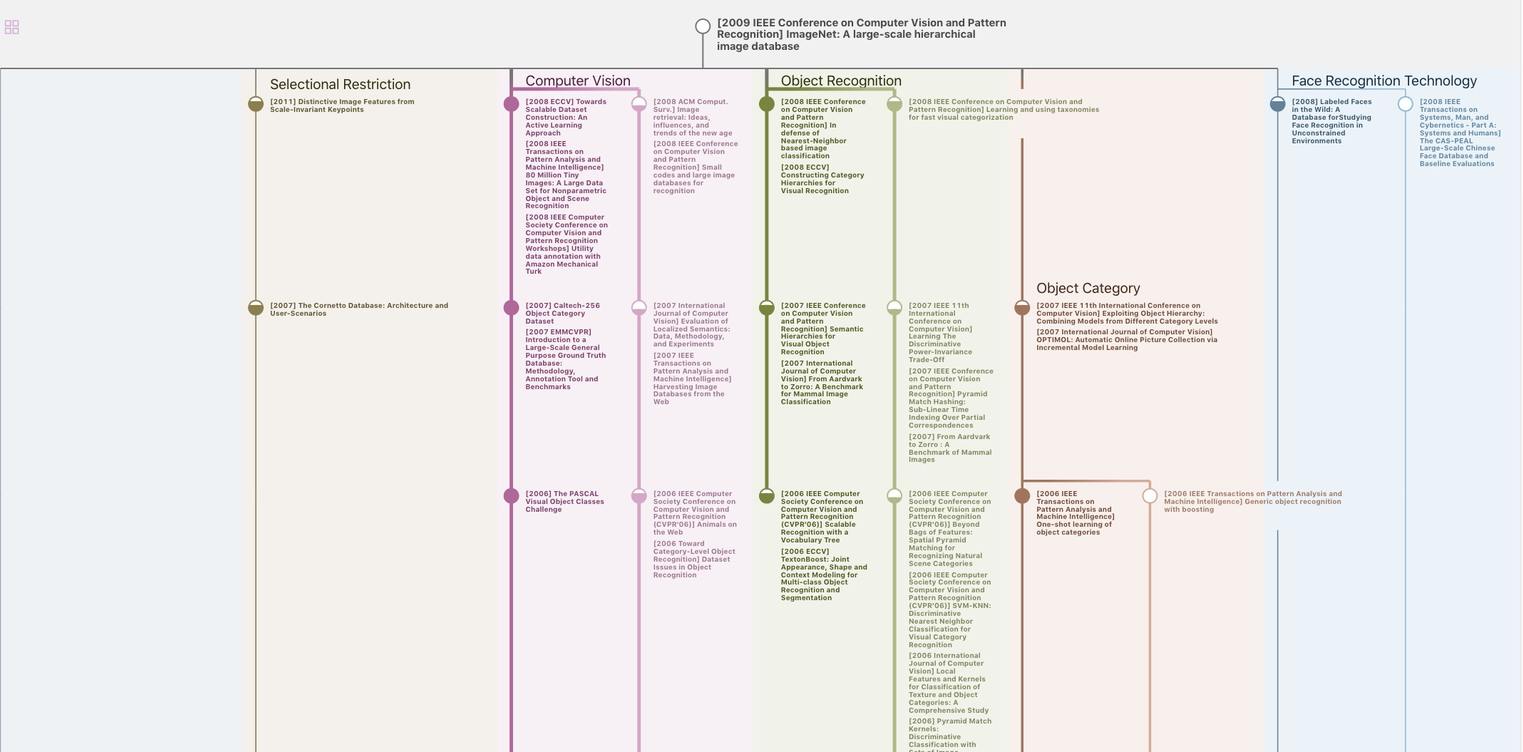
生成溯源树,研究论文发展脉络
Chat Paper
正在生成论文摘要