Time-Synchronized State Estimation Using Graph Neural Networks in Presence of Topology Changes
2023 NORTH AMERICAN POWER SYMPOSIUM, NAPS(2023)
摘要
Recently, there has been a major emphasis on developing data-driven approaches involving machine learning (ML) for high-speed static state estimation (SE) in power systems. The emphasis stems from the ability of ML to overcome difficulties associated with model-based approaches, such as handling of non-Gaussian measurement noise. However, topology changes pose a stiff challenge for performing ML-based SE because the training and test environments become different when such changes occur. This paper circumvents this challenge by formulating a graph neural network (GNN)-based time-synchronized state estimator that considers the physical connections of the power system during the training itself. The results obtained using the IEEE 118-bus system indicate that the GNN-based state estimator outperforms both the model-based linear state estimator and a data-driven deep neural network-based state estimator in the presence of non-Gaussian measurement noise and topology changes, respectively.
更多查看译文
关键词
Graph neural network (GNN),Machine learning (ML),State estimation (SE),and Topology change
AI 理解论文
溯源树
样例
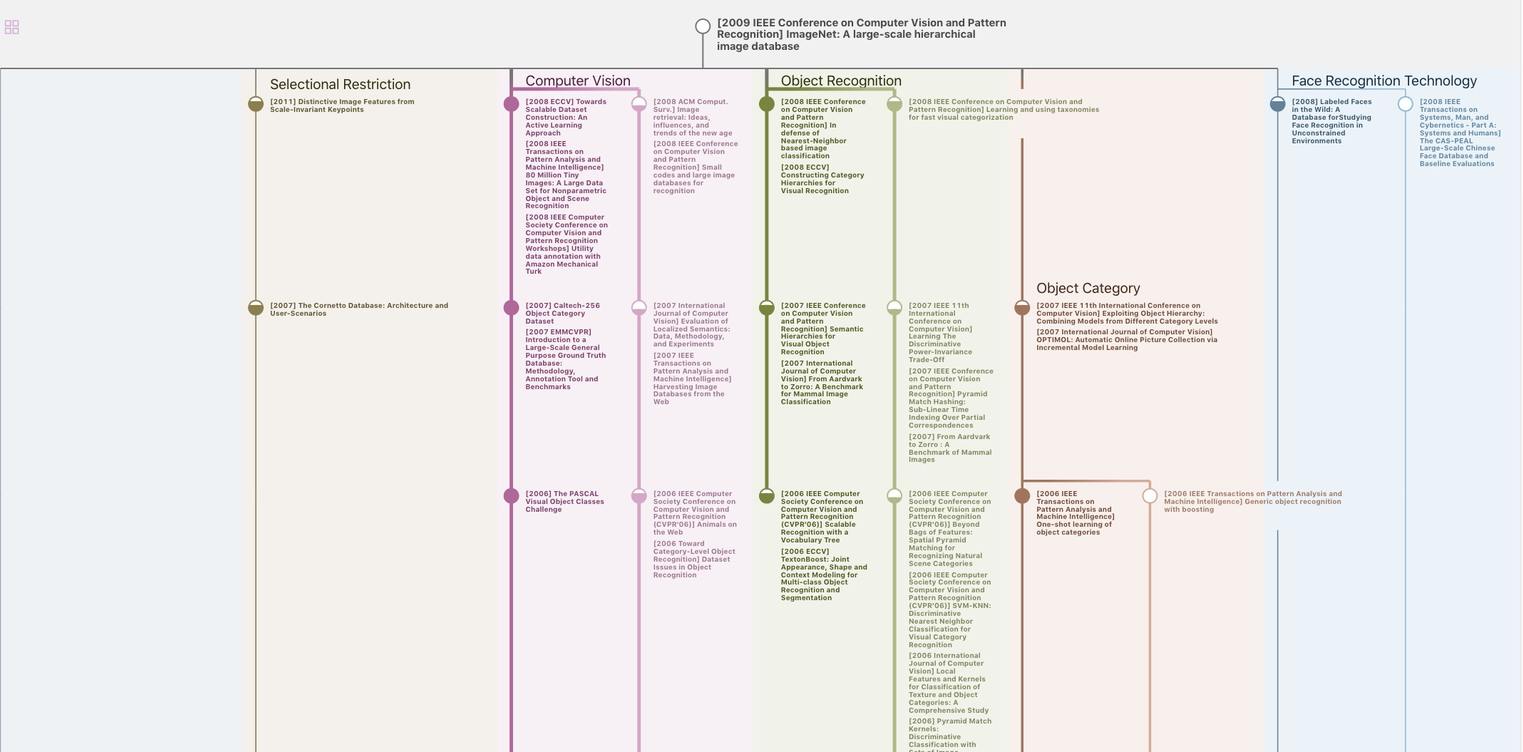
生成溯源树,研究论文发展脉络
Chat Paper
正在生成论文摘要