DDM-NET: End-to-end learning of keypoint feature Detection, Description and Matching for 3D localization
arxiv(2023)
摘要
In this paper, we propose an end-to-end framework that jointly learns keypoint detection, descriptor representation and cross-frame matching for the task of image-based 3D localization. Prior art has tackled each of these components individually, purportedly aiming to alleviate difficulties in effectively train a holistic network. We design a self-supervised image warping correspondence loss for both feature detection and matching, a weakly-supervised epipolar constraints loss on relative camera pose learning, and a directional matching scheme that detects key-point features in a source image and performs coarse-to-fine correspondence search on the target image. We leverage this framework to enforce cycle consistency in our matching module. In addition, we propose a new loss to robustly handle both definite inlier/outlier matches and less-certain matches. The integration of these learning mechanisms enables end-to-end training of a single network performing all three localization components. Bench-marking our approach on public data-sets, exemplifies how such an end-to-end framework is able to yield more accurate localization that out-performs both traditional methods as well as state-of-the-art weakly supervised methods.
更多查看译文
关键词
keypoint feature detection,3d,localization,ddm-net,end-to-end
AI 理解论文
溯源树
样例
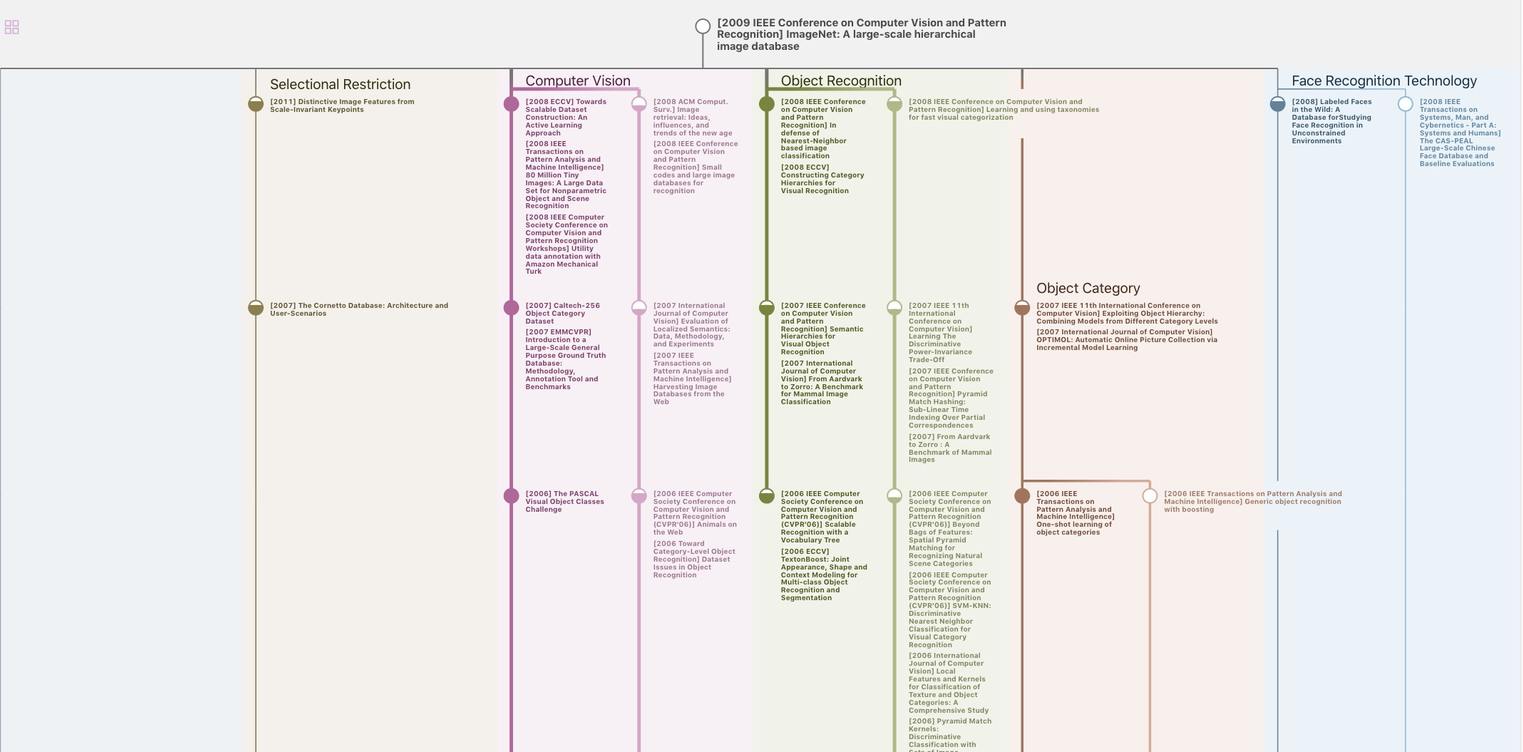
生成溯源树,研究论文发展脉络
Chat Paper
正在生成论文摘要