Least-squares reverse time migration with shifted total variation regularization
Geophysics(2023)
摘要
Reverse time migration (RTM), as a state-of-the-art imaging technique, provides outstanding imaging capabilities due to its use of a full wave equation. Least-squares RTM (LSRTM) seeks the solution of a linearized wave equation via the minimization of a data misfit term; however, the quality of the results de-creases when the assumptions of the method are not satisfied. This occurs, for example, when we use an erroneous velocity model or inadequate physics for inverting the data. In such cases, appropriate regularization is required to mitigate these shortcomings and stabilize the LSRTM solution. However, even for structurally simple earth models, the reflectivity images are complicated and may not be explained properly by particular regularization methods such as the Tikhonov, total variation (TV), or sparse regularization. Reflectivity images can be thought of as the difference between two structurally simpler components: a piecewise-constant component (the true squared slowness) and a smooth component (the background model). We have used a combined Tikhonov-TV regularizer to regular-ize these components separately, leading to an effective regulari-zation for the reflectivity image. Because the background model is known in advance, this combined regularization reduces to a shifted TV regularization for which the associated optimization problem is solved efficiently using a new implementation of the Bregmanized operator splitting algorithm applied to the shifted TV method and the usual TV method. We determine the perfor-mance of our method with a set of numerical examples. The results confirm that our shifted regularization increases the robustness of LSRTM and allows us to estimate high-quality reflectivity images and properly update the background velocity model.
更多查看译文
关键词
total variation regularization,reverse time migration,least-squares
AI 理解论文
溯源树
样例
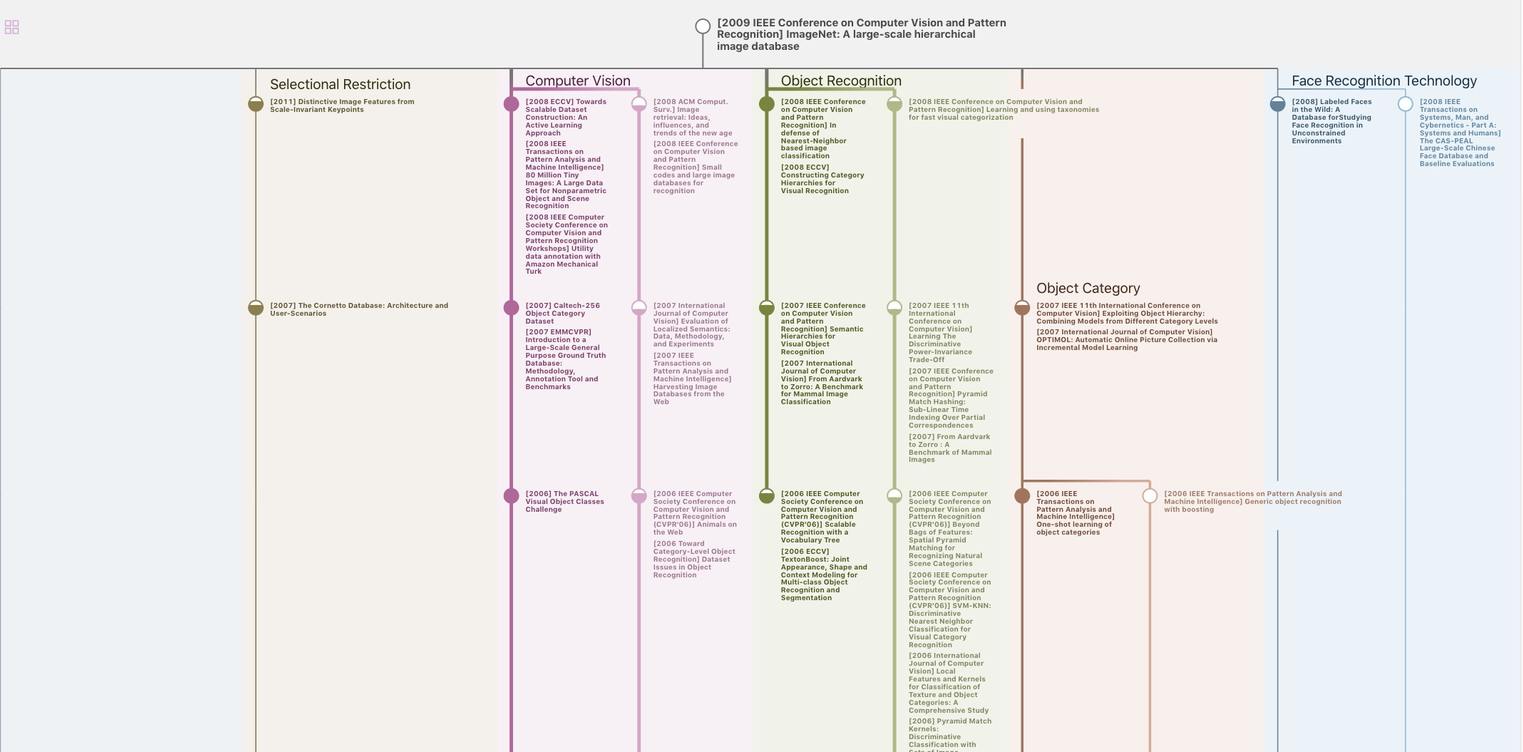
生成溯源树,研究论文发展脉络
Chat Paper
正在生成论文摘要