Quantitative in vitro to in vivo extrapolation of genotoxicity data provides protective estimates of in vivo dose.
Environmental and molecular mutagenesis(2023)
摘要
Genotoxicity assessment is a critical component in the development and evaluation of chemicals. Traditional genotoxicity assays (i.e., mutagenicity, clastogenicity, and aneugenicity) have been limited to dichotomous hazard classification, while other toxicity endpoints are assessed through quantitative determination of points-of-departures (PODs) for setting exposure limits. The more recent higher-throughput in vitro genotoxicity assays, many of which also provide mechanistic information, offer a powerful approach for determining defined PODs for potency ranking and risk assessment. In order to obtain relevant human dose context from the in vitro assays, in vitro to in vivo extrapolation (IVIVE) models are required to determine what dose would elicit a concentration in the body demonstrated to be genotoxic using in vitro assays. Previous work has demonstrated that application of IVIVE models to in vitro bioactivity data can provide PODs that are protective of human health, but there has been no evaluation of how these models perform with in vitro genotoxicity data. Thus, the Genetic Toxicology Technical Committee, under the Health and Environmental Sciences Institute, conducted a case study on 31 reference chemicals to evaluate the performance of IVIVE application to genotoxicity data. The results demonstrate that for most chemicals considered here (20/31), the PODs derived from in vitro data and IVIVE are health protective relative to in vivo PODs from animal studies. PODs were also protective by assay target: mutations (8/13 chemicals), micronuclei (9/12), and aneugenicity markers (4/4). It is envisioned that this novel testing strategy could enhance prioritization, rapid screening, and risk assessment of genotoxic chemicals.
更多查看译文
关键词
clastogen,genetic toxicology,in vitro to in vivo extrapolation,mutation,new approach methodologies
AI 理解论文
溯源树
样例
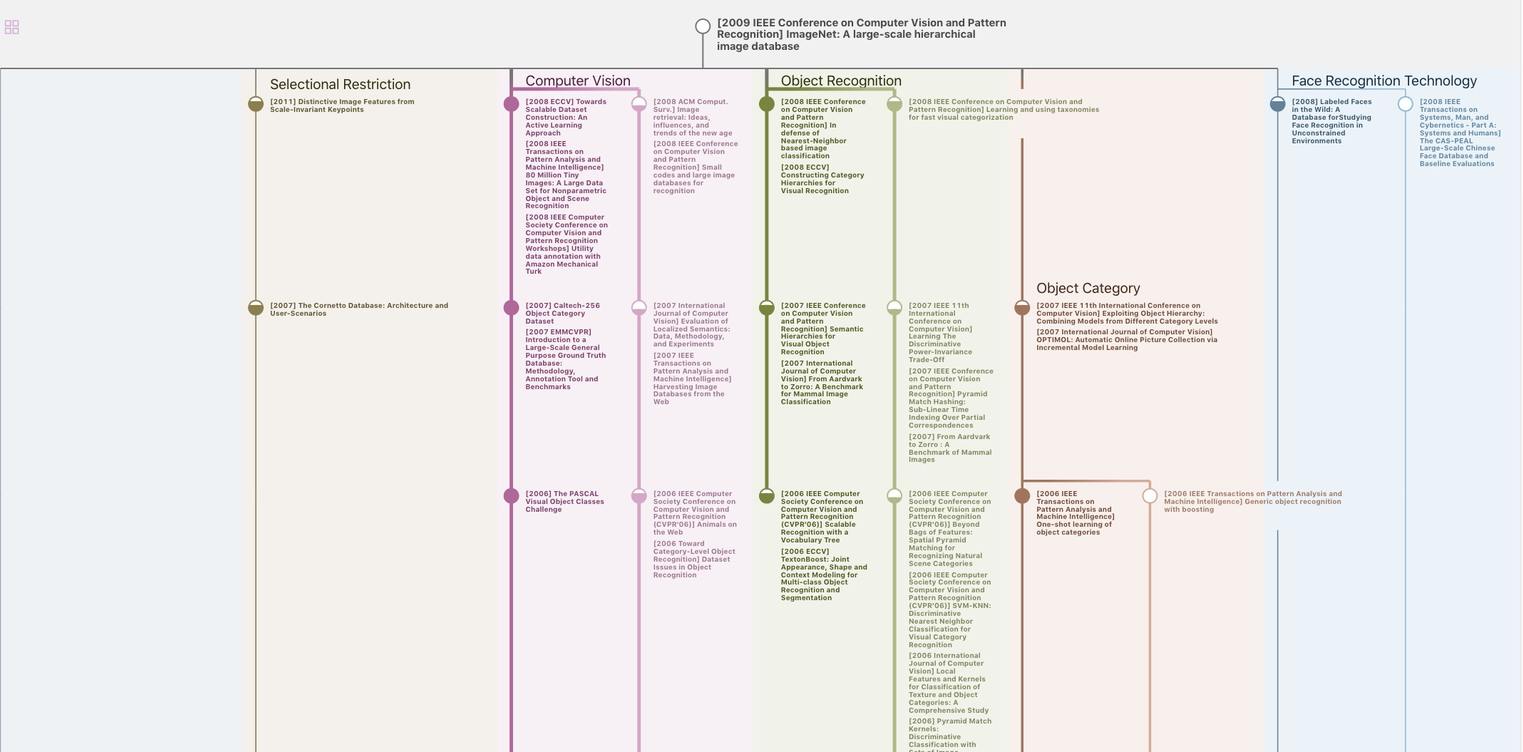
生成溯源树,研究论文发展脉络
Chat Paper
正在生成论文摘要