Flow-Based Encrypted Network Traffic Classification With Graph Neural Networks
IEEE Transactions on Network and Service Management(2023)
摘要
Classifying encrypted traffic from emerging applications is important but challenging as many conventional traffic classification approaches are ineffective, thus calling for novel methods for identifying encrypted network flows. Recent machine learning and deep learning-based approaches are severely limited by their feature selection and inherent neural network architecture. More importantly, they overlook the opportunity to capture latent information in the temporal dimension of packets. As network data by nature are of non-Euclidean distance space and carry abundant chronological and temporal relations, we are inspired to utilize geometric deep learning that simultaneously takes into account packet raw bytes, metadata and packet relations for classifying encrypted network traffic. Our proposed graph neural network (GNN) model outperforms the two reference methods, convolutional neural networks (CNN) and recurrent neural networks (RNN) quantitatively as indicated by three metrics: sensitivity, precision and F1 score.
更多查看译文
关键词
Encrypted network traffic analysis,network traffic classification,deep learning,graph neural networks,multimodal deep learning
AI 理解论文
溯源树
样例
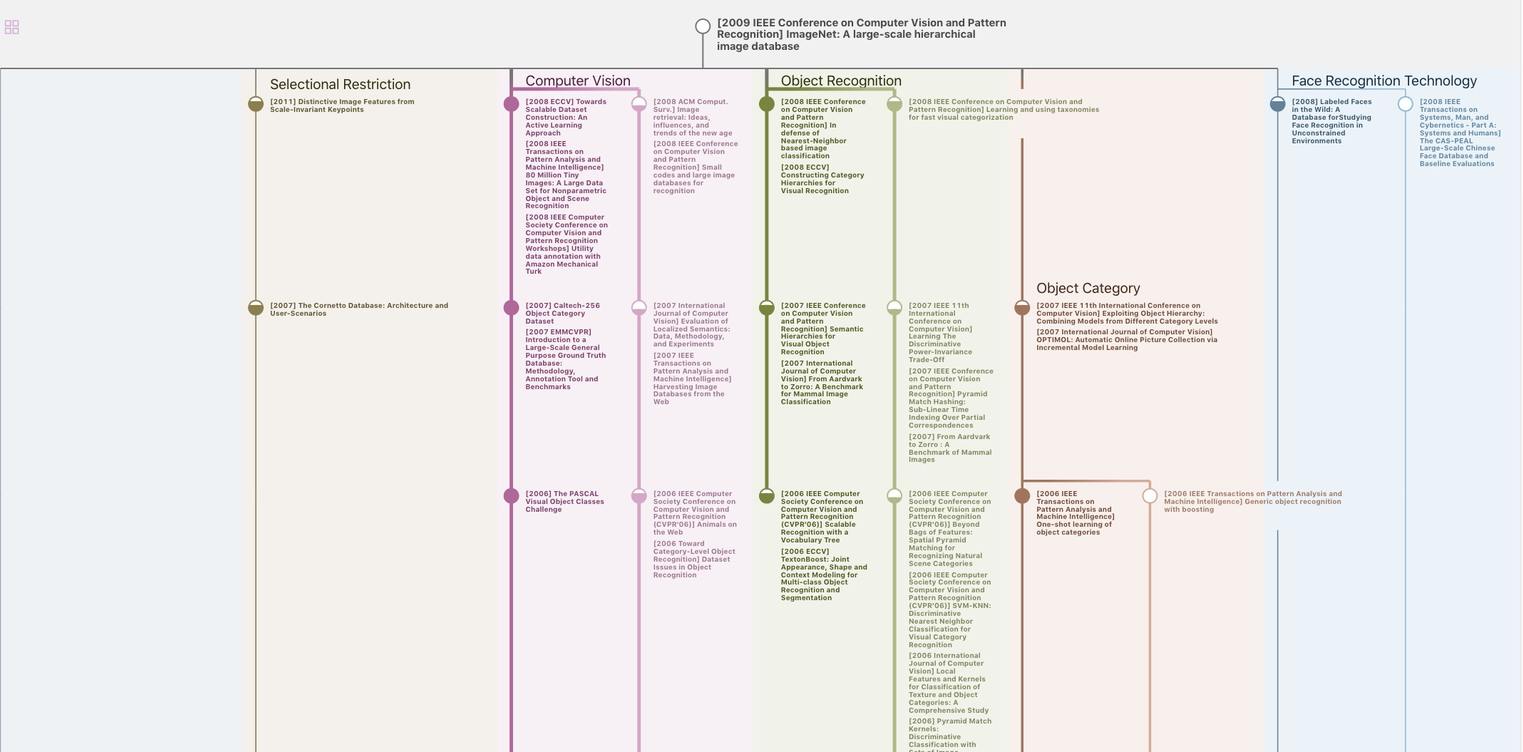
生成溯源树,研究论文发展脉络
Chat Paper
正在生成论文摘要